This book is distributed under the terms of the Creative Commons Attribution-NonCommercial-NoDerivs License which permits noncommercial use and distribution provided the original author(s) and source are credited. (See https://creativecommons.org/licenses/by-nc-nd/4.0/
NCBI Bookshelf. A service of the National Library of Medicine, National Institutes of Health.
Structured Abstract
Background:
When making complicated health-related decisions, both patients and clinicians want to understand which treatments work best. The decisions may be supported by findings from randomized trials or, when they are not available, by the findings from observational data analyses that explicitly emulate a hypothetical randomized trial: the target trial.
Objectives:
We developed Comparative Effectiveness Research Based on Observational Data to Emulate a Target (CERBOT) Trial, a web-based tool that provides a structured standardized algorithm to define and emulate a target trial using observational data.
Methods:
The research team designed and developed CERBOT using the following process: (1) stakeholders and expert engagement; (2) background literature review; (3) content development; (4) development of virtual wireframe and CERBOT website; and (5) alpha testing. We established the guiding principles for CERBOT development through a consensus process involving an 8-member advisory committee. As its basis, CERBOT uses a conceptual framework that identifies observational analysis as a means to emulate a target trial. This could be achieved by explicitly formulating the protocol of the target trial and addressing the feasibility of the conditions that must be met in order to emulate the target trial using observational data. We developed the actual CERBOT website through close collaboration with a web development firm using the most up-to-date technologies.
Results:
We developed an interactive user-friendly online tool, CERBOT.org. It includes 5 modules used to design and operationalize a comparative effectiveness research study by emulating a target trial. By synthesizing the information entered by users, CERBOT provides specific recommendations for causal inference analytical methods based on each user's individual research question. CERBOT also facilitates the formation and use of a multidisciplinary stakeholder research team. Through use of this research circle, it creates a communication platform for team members to collaboratively complete CERBOT modules by sharing ideas, comments, and conclusions.
Conclusions:
CERBOT makes it easier for patients to participate in clinical research, by expressing their priorities and preferences in the design of the target trial, and assures researchers that their observational analysis is consistent with fundamental principles of causal inference.
Limitations:
More in-depth case studies are needed to demonstrate CERBOT's applicability for more complex interventions. Extensive testing in real-world settings is also warranted to assess merits, deficits, and feasibility of use.
Background
To understand whether or how a therapy or intervention may affect a patient outcome, causal inference is often sought.1 For example, “What is the benefit or harm of using a new medication to ameliorate the outcomes of Alzheimer's disease?” is a causal question. While randomized clinical trials (RCTs), which determine the effects of various interventions on patient outcomes, remain the gold standard for answering such a question, they often are not ethically, practically, or economically feasible. In these cases, causal inference must be based instead on comparative effectiveness research (CER) studies that use observational data to provide timely answers.2,3 With the growth of electronic health records and increasing efforts to systematically collect information from routine clinical encounters, the potential for use of real-world observational data is exploding.4,5
Causal inference from observational data requires 2 steps6:
- Step 1 is formulating a well-defined causal question relevant to decision-making.7 A well-defined causal question is a question for which one can specify the hypothetical RTC that would answer it.6,8 We refer to this hypothetical trial as the target trial.8,9,10 For example, several anemia management strategies commonly used among dialysis patients have not been examined in actual randomized studies. A well-defined causal question to compare anemia management strategies using observational data can be formulated by explicitly specifying the protocol of the target trial that stakeholders would seek to conduct to address the question.7,8 Components of the target trial protocol can be specified in formats similar to those defined in the PICO (population, intervention, comparator, and outcome) framework.11
- Step 2 is providing an answer by emulating the target trial specified in step 1 using the available observational data.9
We developed a web-based tool called Comparative Effectiveness Research Based on Observational Data to Emulate a Target (CERBOT) Trial to help researchers specify and emulate target trials using observational data, a typically iterative process (Figure 1). Specifically, CERBOT does the following: (1) guides users to explicitly specify and emulate 5 critical components of the target trial protocol, which include eligibility criteria, treatment strategies, outcome of interest, follow-up period, and causal contrast(s) of interest; (2) helps users select appropriate methods for analysis; and (3) provides a structure for stakeholders and methodologists to engage in both specification and emulation of the target trial.

Figure 1
CERBOT Flowchart Depicting Completion of 5 Modules.
This report describes the features of CERBOT (http://CERBOT.org), which is designed to be used by researchers and clinicians with basic statistical training, who will work with selected teams of other researchers and stakeholders. Depending on the specific question, such stakeholders could include clinicians, policy makers, regulators, patients, patient advocates, caregivers, third-party payers, and manufacturers.
Methods
The research team designed and developed CERBOT over 3 years using an approach comprising 5 major parts: (1) stakeholders and expert engagement; (2) background research; content development; (4) development of virtual wireframe and CERBOT website; and (5) conduct of in-house and CITAC testing (alpha testing).
Stakeholders and Expert Engagement
The research team developed CERBOT through a consensus process that involved a group of academic, industry, and clinician researchers. This consultation group was tasked with reviewing feasible frameworks to help in the development and implementation of a causal inference toolkit. At the beginning of the project, the research team formed an 8-member Causal Inference Toolkit Advisory Committee (CITAC) that included clinicians, applied researchers, elite statisticians, experts in dissemination and implementation fields, and leaders in the pharmaceutical industry (Table 1).
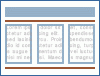
Table 1
CITAC Members.
We achieved consensus through the nominal group technique (NGT), which is a structured variation of a small-group discussion to reach consensus.12,13 The NGT process prevents the domination of discussions by a single person or opinion leader and instead encourages all group members to participate; it results in a set of prioritized solutions or recommendations that represent the group's preferences. The NGT also has the advantage of diminishing competition and pressure to conform based on the status within a group. Specifically, we utilized the general 4-step process to conduct the NGT as follows:
- The research team presented the “problem” of designing, creating, and implementing CERBOT.
- CITAC engaged in round-robin feedback sessions during a 2-day meeting (described below) to discuss various ideas until all members' ideas/thoughts/recommendations were documented.
- Individual ideas were discussed in more detail to determine clarity and importance. This step provided the opportunity for CITAC members to express understanding of the logic and relative importance of each idea.
- The research team identified the most high-priority ideas/suggestions based on CITAC members' advocacy related to specific aspects of CERBOT design and implementation.
The remainder of this section provides details on how the NGT was implemented. The research team's first CITAC in-person daylong meeting took place on March 21, 2014. There the research team presented to CITAC the objectives of the project and introduced general conceptual steps for conducting causal inference using observational data. Globally, the research team outlined and discussed 3 missions of the causal inference toolkit: (1) Educate stakeholders in the development of a well-defined research question via interactive step-by-step instructions on how to emulate a randomized hypothetical trial using observational data; (2) provide guidance on the selection and use of CER techniques—especially causal modeling techniques; and (3) foster the collaboration of various expert stakeholders with a wide variety of complementary skill sets.
CITAC members provided important feedback regarding the aim, scope, functionality, and target audience of the toolkit; overall framework for building the toolkit; and ways to make the toolkit more user friendly and accessible. The team and CITAC also discussed and agreed on the following 4 guiding principles for toolkit development:
- The information that CERBOT offers needs to be explicit regarding its usability as well as its limitations.
- CERBOT must be welcoming (instead of intimidating) to the diverse needs and knowledge of its users, who may have varying levels of skill and experience in CER.
- The toolkit has to be easy to read and navigate to ensure its relevance, acceptability, usefulness, and value.
- Instructions for using the toolkit need to be illustrated by simple and straightforward examples rather than long texts.
During the second day of the meeting, the research team and CITAC agreed on using the target trial framework as the conceptual basis for developing CERBOT. Attendees further discussed both traditional and nontraditional ways to make the tool more user friendly and patient centered. For example, committee members emphasized that the toolkit needed to be welcoming, not intimidating, at every stage of the process; examples should always be provided to illustrate complex concepts or ideas; didactic content should be succinct and to the point; and the toolkit should be easy to access and navigate and should have interactive features.
The research team and CITAC agreed that the optimal format of the toolkit should be an interactive, cloud-based website that would house the learning modules, examples, and reporting functionality. Given the scientific nature of the website, CITAC suggested that the target audience for the website would be health care and/or clinician researchers who had previous experience with epidemiological investigations. The tool will be particularly useful to researchers who are currently using traditional noncausal techniques and until recently have lacked guidance in how to conduct causal inference studies, but who, now, may consider shifting to causal inference studies. By following the structure and template provided by CERBOT, users are assured that their CER study design is consistent with the fundamental principles of CER for causal inference.
Based on the committee's feedback, particularly regarding identification of CERBOT's target audience as researchers with basic statistical knowledge, the team developed the preliminary website wireframe and content pages, and distributed related documents to CITAC on January 15, 2015. Based on CITAC's subsequent feedback, the research team finalized the main features of CERBOT and shared them with CITAC members in June 2015.
Following Years 2 and 3, the focus was delineating CERBOT requirements regarding user experience and building the CERBOT website based on the defined requirements. During these 2 years, the focus was 2-fold: technological development of the website itself, by collaboration with a web development subcontractor; and continued communication with committee members through regular contact to discuss incremental progress of the website development as well as its specific content. The advisory committee led the effort to promote user-friendliness, as general clinicians may have limited knowledge regarding causal inference but are interested in learning more about using it to conduct their CER research.
During September and November of 2016, a preliminary version of the tool was piloted within CITAC. The research team asked the committee to evaluate the user-friendliness of the site and to assess the content of the website itself, specifically its value and utility as a resource for a CER team. Committee members provided their views and suggestions on the potential utility of the modules, interactive features, and navigation of CERBOT. After addressing CITAC's concerns, the team made CERBOT version 1.0 available to the public in January 2017. By actively engaging CITAC members in the process of developing the causal inference toolkit, the project ensured credibility, transparency, user-friendliness, and enhanced value for a wide variety of stakeholders.
Background Research
The research team conducted background research to help establish guiding principles and to refine the conceptual framework for the development of CERBOT. As described in the Background section, the basic idea behind CERBOT is that CER observational studies need to emulate hypothetical RCTs as closely as possible. Thus, the background research included a literature review of articles published in academic journals. Specifically, we performed a PubMed search for English-language articles from 1975 to January 2017, using the following keywords: causal inference framework, target (or hypothetical) trial, and emulation. We grouped articles into 2 categories.
The first category included review studies that introduced and discussed the need to frame research questions as hypothetical randomized trials in order to make them directly relevant for decision making. Hernán and Robins outlined a target trial framework and emphasized that causal analyses of observational data need to be evaluated in terms of how well they emulate a particular target trial.8
The second category of articles included actual CER studies that explicitly or inexplicitly followed the target trial framework in their design and analysis of observational studies for comparative effectiveness and safety. Prior explicit attempts to emulate trials using observational data have studied, for example, postmenopausal hormone therapy,14 statins,15 epoetin,16,17 screening colonoscopy,18 and antiretroviral therapy.19 Some studies followed the target trial framework to analyze observational data, although the target trial was not explicitly described.18,20 Review of the literature helped the team envision how specific steps within this general framework should be constructed for CERBOT users to follow.
Additionally, the research team conducted a search on best CER practices, reporting guidelines, methodology standards, and frameworks related, directly or indirectly, to the design and implementation of causal inference methods. The purpose of the scan was to better understand existing work, to ensure that the development of CERBOT was evidence based, and to help identify the original and unique features of CERBOT.21-33
Content Development
The content builds on the framework that the project team and CITAC developed for the CER study design and CER analysis. Consistent with the framework, CERBOT guides users to conduct their observational analyses according to the following steps: (1) engage relevant stakeholders to work collaboratively on the CER question; (2) articulate the research question in terms of a hypothetical randomized trial (the target trial); (3) outline how to emulate the target trial using observational data; and (4) select suitable analytical methods based on user's specific research question.
CERBOT supports the full scope of CER questions. Our premise is that any CER question can be articulated in terms of a hypothetical target trial. If a target trial cannot be proposed, then the comparative effectiveness question is deemed ill defined. The use of the target trial framework ensures that the research focuses on the effects of interventions that occur in the data and are well defined.21-23
The intention was for the tool to be useful to researchers and others with varying levels of experience in causal inference research, and for the text to be informative and instructive for both neophytes and experts. For example, when guiding users to emulate the target trial, a template is provided to facilitate the process. Project leaders prepared the first draft of CERBOT pages, using the results of their background research as well as communication with CITAC. Then all project team members reviewed and edited CERBOT pages for content and structure. The investigative team conducted past and ongoing content management, continuously making small-scale modifications.
Development of Virtual Wireframe and CERBOT Website
Since July 2016, the project team has been working closely with a web development team to create the actual website that will deliver the required functionalities. The team used Agile methodology to work with the web development team in the virtual wireframe and website development. During this process, the investigative team oversaw the entire effort, including design, development, deployment, regression testing, bug fixing, and performance testing, by maintaining a close working relationship with the web development team. Specifically, the project team conducted in-person meetings and conference calls with the web development team to share user stories and to help team members understand project requirements. The project team also communicated closely with web development team to ensure appropriate design development, project progress, and on-time delivery.
For website quality control, the project team tested CERBOT's features and used the Jira tracking system21 for compiling and monitoring reported problems. Subsequently, the research team and developers documented, discussed, and rectified most of the issues recorded in Jira. Finally, we set up a dedicated server responsible for incorporating the latest changes and updates, known as a build server, for the developers to ensure constant availability of the latest revised version.
Conduct of In-house and CITAC Testing
To assess the functionality of CERBOT and to ensure that it met all project objectives, the research team began testing CERBOT in December 2016 after it was first released by the developers to the research team. We conducted 2 stages of alpha testing. Members from the project team, including the principal investigator (PI), co-PI, and 2 other key personnel, conducted comprehensive testing of CERBOT functionalities during the first stage. Defects and bugs were identified and remedies were prioritized during several iterations of testing. At the completion of the first testing stage, we held a meeting with the website developer, during which we used a structured questionnaire to determine unmet requirements regarding CERBOT flow and functionality. Considering budget limits, the research team prioritized issues to be addressed and then worked closely with the web development team to fix the 5 most critical defects: navigation, parallel process of specification and emulation, research circle functionality, interactive features, and reporting functionalities.
After the main defects were fixed, the research team started the second stage of alpha testing by releasing CERBOT to CITAC and instructing CITAC members to conduct 1 to 2 hours of testing. Because CITAC members were involved in conceptualizing CERBOT (although they were not involved directly in actual development of the CERBOT website), we define testing conducted by expert committee members as alpha testing. Three committee members provided feedback, which focused primarily on 3 areas:
- Functionality issues. Reviewers identified additional defects, chiefly related to interactive features. We again organized, prioritized, and addressed additional defects, according to their importance.
- Case studies. All reviewers considered case studies critical to understanding how to use the website and suggested better ways to present case studies for users. We subsequently developed a new tutorial video and included 2 existing studies15,22 in CERBOT to illustrate how each module should be completed.
- Better presentation of important causal concepts. All tasks and testing activities described above were managed by the Jira system21 and discussed with the web development team.
Results
Building on the conceptual framework of the target trial approach, the following features formed the core elements of CERBOT.
Formation of a Research Circle
To foster collaboration between researchers and stakeholders, CERBOT facilitates joint work by a research team to complete the modules and includes, for each module, the format for a discussion section among research team members. This allows for the creation of a research circle, made up of all team members working on a single research question, and enables members to interact and share ideas about CER study design and analysis.
Each collaborative research circle is led by the user who created the research question using CERBOT. To build a research circle, a user invites other people to join the team and collaborate on a specified research question via email exchanges. Invitees join the circle via a link provided in the email text. Research circle members can then jointly and concurrently work on completing the modules. For example, if a researcher does not know how to define acute kidney failure, using ICD-9 diagnosis or procedure codes, the researcher has 3 options: (1) Notify a team clinician of this in the “Concerns” box; (2) raise the issue in the “Discussion” section following the module report; or ask the question directly via email message in the “Research Circle” box included in CERBOT. Modules and reports are then automatically updated after a team member addresses and resolves the concern. In addition, team members can post/create topics and comments in the discussion section linked to specific modules.
Five Modules Support Parallel and Iterative Processes of Specifying and Emulating the Target Trial
In Figure 1, the CERBOT flowchart illustrates the process of specifying and emulating the target trial. Five essential CERBOT modules—eligibility criteria, study outcomes, follow-up, treatment strategies, and causal contrast and adjustment variables—correspond to 5 key components of the protocol of the target trial. Each module requires 3 types of input from the users:
- Specification of the corresponding component of the target trial protocol
- Emulation of the corresponding component of protocol, using the available observational data
- Concerns about the feasibility of using the observational data to emulate the corresponding component
For example, if the eligibility criteria require patients to have both normal hemoglobin and cholesterol levels at the baseline, but hemoglobin information is not included in the data set, the user repeats the process with new input; for example, redefining the target population, by dropping the requirement for hemoglobin levels, may achieve better results.
CERBOT is structured to support users by facilitating their specification and emulation of the target trial through a side-by-side and iterative process. If users need to learn about the meaning of a certain component or question, they can read the helper text under each component or click on the “Tip” link provided. A small pop-up window opens with instructions, examples regarding completion of the specific component, and answers to specific questions. Users can view, revise/edit, save module updates, and continue to research previously saved questions. Two case studies included in CERBOT show examples using the complete process, from the initial specification, emulation, and resolving of concerns through the report, any revisions, and results.
CERBOT Guides the Selection of Causal Inference Methods
Using the information entered by the user in previous modules, CERBOT recommends appropriate causal inference approaches for data analysis. Specifically, CERBOT asks 7 questions to recommend a specific method (Figure 2). It is important to note that CERBOT is not intended to serve as a data-processing or computing software that constructs analytical data or calculates estimated risks. For implementation of CERBOT-recommended methods, a brief tutorial and pertinent literature from the “CERBOT Resource” page are provided on the website. The 7 questions driving the decision regarding appropriate analytical methods are the following:

Figure 2
Algorithms used by CERBOT for recommending analytical methods based on answers to 7 questions from CERBOT users.
- What type of treatment strategy do you want to compare: point or sustained over time? The compared strategies can be baseline interventions, which happen only at a single point in time (eg, immediate surgery), or strategies that are sustained over time (eg, taking 75 mg of aspirin every day for the rest of one's life). Sustained treatment strategies are static when all treatment decisions over the follow-up period are predetermined at the baseline, as in the aspirin example; they are dynamic when treatment decisions, at different times during follow-up, depend on time-evolving patient characteristics.23,24 Many treatment strategies for the care of people with chronic medical conditions are dynamic. For example, patients receiving dialysis receive epoetin to treat their anemia, with the dose adjusted over time, according to both prior epoetin dose and hematocrit or hemoglobin levels (laboratory values that measure the extent of anemia). The choice of methods for causal inference primarily pertains to the types of interventions or treatment strategies being compared.9,25
- What is your causal effect of interest: intention-to-treat or per-protocol? Intention-to-treat effect is the comparative effect of being assigned to the treatment strategies at baseline, regardless of whether the individuals continue following the strategies after baseline. When comparing sustained treatment strategies, however, patients and clinicians are often interested in estimating the effect of following the treatment strategies specified in the protocol of the target trial—ie, the per-protocol effect, not the intention-to-treat effect.26,27 Generally, estimating the per-protocol effect requires adjustment for prebaseline and postbaseline prognostic factors that affect adherence to the protocol and/or loss to follow-up.28,29 Conventional statistical methods, however, cannot appropriately adjust for confounding due to postbaseline prognostic factors that affect treatment levels and are themselves affected by past treatment (often referred to as treatment-confounder feedback30). Examples include hematocrit when comparing dynamic strategies for epoetin among dialysis patients17 and CD4 counts when comparing antiretroviral treatment strategies among HIV patients.31,32 In contrast, g-methods23,33 and doubly robust methods34,35 are specifically designed to handle time-varying confounders affected by previous treatment.
- Do you have an instrumental variable? When large or unknown sources of unmeasured confounding are suspected, investigators may consider turning to instrumental variable (IV) methods.36 A suitable IV should be strongly associated with the treatment of interest and affect the outcome only through the treatment received, and should not be associated with any measured and unmeasured confounders.37 If an instrumental variable is proposed, investigators can choose this method for intention-to-treat analysis. The IV approach, with several strong conditions, may consistently estimate the average causal effect of an exposure on an outcome even in the presence of unmeasured confounding.38
- Do you have treatment-confounder feedback (time-dependent confounding)? As described above, standard methods cannot be used for sustained interventions when there is treatment-confounder feedback.39 Rather, valid adjustments to measure confounding require the use of g-methods.40,41To summarize 1 to 4 above, g-methods should be used when there is treatment-confounder feedback of a sustained exposure regimen and the per-protocol effect is targeted; otherwise, alternative approaches can be utilized. If there is an IV for a point-treatment question, consider using the IV.
- Is censoring or loss to follow-up informative? In the presence of selection bias due to loss to follow-up, adjustment for postbaseline factors may also be needed to validly estimate both intention-to-treat effects and per-protocol effects. Such adjustments may be necessary in both actual trials and observational analyses that emulate a target trial.42 When postbaseline adjustment factors are affected by the treatment strategies themselves, g-methods are generally needed.14,43
- Do treatment strategies have a grace period? A grace period is the designated time period during which the intervention can happen. A consequence of having a grace period is that, during the grace period, an individual's observational data can be consistent with >1 strategy. Using an epoetin-initiating strategy as a grace period example, a 4-week period implies that the strategies could be redefined as “initiate ESA therapy within 4 weeks of hemoglobin drops below 11” or “initiate ESA therapy within 4 weeks of hemoglobin drops below 12.” Therefore, an individual who starts therapy in week 4 postbaseline has data consistent with all strategies during weeks 1, 2, and 3. One way to measure a grace period is to randomly assign the individual to one of the strategies of interest. Another way is to create exact copies (clones), with each copy assigned to a different strategy.44 Each copy is censored when it deviates from its originally assigned treatment strategy, often referred to as artificial censoring. An intention-to-treat analysis is not suitable because each individual may have been assigned to several strategies at baseline and thus has several copies. Additionally, the potential selection bias, introduced by artificial censoring, needs to be corrected by appropriate adjustment for time-varying factors (eg, via inverse probability weighting).45
- Is your study outcome a binary, a continuous, or a failure time variable? Logistic regression, linear regression, and survival curves can be constructed to handle a binary outcome variable, a continuous outcome variable, and a time-to-event outcome variable, respectively.46
CERBOT “reacts” or responds to the individual user's specific input. Its dynamic structure picks up the user's choices and accordingly structures the study design, adjustment variables, and choice of causal inference methods. For example, if the answer to the question “Do you expect that censoring may result in selection bias?” in module 3 (Study Follow-up) is “Yes,” then CERBOT prompts users to define a time-varying variable in module 5. This added variable is then included in the analysis plan to account for loss to follow-up and/or other censoring events.
Generation of Individual Module Reports and Final Comprehensive Analysis Plan
Each module produces an individual report, which is a summary of all the questions/answers in the current module. Each automatic module report is intended to serve as the basis for discussion among research team members and illuminates any subsequent revisions of the input. Users can view or download this report after submitting answers to required questions. Upon completion of modules 1 to 5, CERBOT users are provided with a final report page summarizing the entire study design. Also provided are recommended methods that include standard statistical methods as well as new methods developed for causal inference that use observational data, such as g-methods,33 instrumental variables,47 and doubly robust methods35,48 (Figure 2). These reports are downloadable, can be edited in Microsoft Word format, and can be shared among the entire research team.
CERBOT Tutorial and Case Studies
The research team created a short tutorial video, linked to the CERBOT website, to help users understand the overall functionality of CERBOT. The tutorial provides an overview of the main objectives of CERBOT, how to create a research question, how to invite team members as part of the research circle, and how generally to navigate the website. To illustrate how each module should be completed, the research team added 2 case studies based on the team's published research,17,49 using sustained interventions that explicitly follow the framework for specifying and emulating a target trial. Each case study showcases how the specification and emulation process should be completed and reported. Case studies can be browsed by clicking the “Case Studies” button in the sidebar of each module page.
CERBOT Dissemination Activities
We conducted many limited dissemination activities to identify potential CERBOT users and familiarize them with the concepts and functionality of the causal inference website. First, Yi Zhang, PhD, MS, project PI, gave 2 presentations on CERBOT use and functionality at the Joint Statistical Meeting in 2015 and 2016, respectively. Her presentations generated interest among conference attendees, who are currently awaiting the final finished website. Second, at the PCORI-funded Causal Inference Methods for PCOR Using Observational Data (CIMPOD) meeting held in February 2017, we set up a booth to allow conference attendees—most of whom were statisticians or clinical researchers with interest in using causal methods—to “test” CERBOT and provide feedback. Overall, we found tremendous interest in using CERBOT among CIMPOD attendees; their feedback included the need for case studies as well as a tutorial to provide guidance in the use of CERBOT for a novice user. The research team has subsequently addressed these suggestions. Third, we have introduced CERBOT to 5 sites comprising 11 research teams that we are currently working with under a PCORI dissemination and implementation (D&I) project to disseminate use of g-methods to researchers with ongoing CER questions. These teams are currently in the process of receiving tutorials in the use of g-methods, particularly the more complex g-formula, and hope to use CERBOT after this foundational work to augment their current software and research tools.
CERBOT Development, Maintenance, and License
We built the CERBOT website on NodeJS, React, and Redux technologies with MongoDB as database configuration and Amazon EC2 as the production server. The maintenance of CERBOT includes updates and changes to the contents of the website, troubleshooting, and data backup and archiving using Amazon Web Services. The production website URL (http://www.cerbot.org) serves as the CERBOT tool. It can be accessed through standard web browsers running in various operating systems and hardware platforms. CERBOT is available at no cost to the public and can be distributed or shared freely at any time, provided the original work is properly cited and the use is noncommercial.
Discussion
Study Results in Context
The key concept underlying CERBOT is that observational analyses can be viewed as an endeavor to emulate a hypothetical randomized trial—the target trial—to answer a causal question that is not feasible for RCTs.9 The target trial approach is consistent with the formal counterfactual theory of causal inference,50,51 provides an organizing principle for causal inference methods that implicitly rely on counterfactual reasoning,52,53 and prevents common methodological pitfalls, such as immortal time bias and selection bias. The target trial approach also facilitates a systematic and transparent evaluation of the observational study design9 and thus promotes better communication and understanding. The utility of this causal inference framework has been demonstrated in a variety of applications and across many disease areas.29,54-56
CERBOT implements the target trial approach primarily by guiding users to complete 5 modules included in CERBOT. Consistent with existing study design and reporting guidelines,57-61 as well as PCORI Methodology Standards. these 5 modules are structured to support the parallel process of specifying and emulating the target trial for the question at hand. Many investigators, for example, are unfamiliar with the idea of emulation of a target trial and may have trouble trying to implement it in practice. CERBOT helps investigators make the emulation explicit, by breaking up this complicated concept into easy-to-follow steps. For example, necessary components listed in each module are accompanied by instructions and tips to guide researchers or clinicians. This allows people with limited knowledge of causal inference to design a study and select an analytic approach that is consistent with causal inference principles. Researchers can continue to apply the structure provided by CERBOT to clarify the target trial and to organize information about available data to emulate the trial.
CERBOT can be used as a complement to several other causal inference introductory tools and/or educational materials that now exist, for use in observational data to better assess causality and to allow for less biased estimates of effect. Multiple tools that address many CER topics and are presented in a variety of ways include tutorials, conferences62 and workshops,63 presentation slides, videos, short courses, webinars, and software supports. For example, the complete online course “Introduction to Causal Inference” (www.ucbbiostat.com) by Petersen and Balzer provides a roadmap and necessary steps for causal inference studies using observational data; journal articles by Greenland,37 Ahern et al,64 and Naimi33 provide tutorials on the methods presented in CERBOT.
Compared with existing introductory tools for causal inference, CERBOT has a unique focus on explicitly defining causal questions and provides an approach to emulate a target trial within an observational setting. An innovative feature of CERBOT is that it is organized as a collaboration site for stakeholders and researchers to jointly contribute their knowledge and expertise.
Uptake of Study Results
The research team recognizes several challenges in promoting and disseminating CERBOT for designing CER and implementing causal inference methods. Most important, CERBOT is still in its inaugural phase and an extensive assessment for its feasibility in real-world settings is needed. Such an assessment would include a collection of case studies that illustrate, by detailed examples, how to explicitly emulate a target trial using CERBOT. Further adaptations may be useful to refine the components included in each module or flowchart used for selecting appropriate methods. To date, the CERBOT development process has involved only project investigators and CITAC committee members. Although all committee members are notable researchers in the fields of CER and public health, successful promotion and dissemination of CERBOT must include other researchers, methodologists, and statisticians across various health and related research fields. To enhance future updates of CERBOT, we will apply for a PCORI D&I project to conduct a pseudo-experimental study in order to assess and compare study designs and outcomes, among a cohort of researchers using CERBOT, vs contemporaries who use another educational platform. In future efforts, we propose the inclusion of CERBOT in introductory statistical courses as well as its use in biostatistical departments across various academic centers. Finally, we aim to present CERBOT capabilities and functionalities at upcoming meetings regarding statistical and health services research, and patient outcomes issues.
Future Research
Several limitations are noteworthy. Most notably, extensive testing for feasibility in real-world settings is warranted to assess both merits and deficiencies of using CERBOT. While CERBOT presents complex topics in simple language for purposes of accessibility and understanding, users may be required to review material elsewhere for more in-depth understanding and project specificity. Finally, additional case studies that present complex dynamic interventions are required and are not currently available to permit users to better understand design and analysis of such studies using observational data.
Future work will focus on adding components to CERBOT to improve ease of use, integrate other existing CER tools into CERBOT, and enhance interactive features to improve the decision tree for choosing appropriate methods. Planned tool developments include the following:
- Integrate additional complex case studies into CERBOT using a tutorial option. It will allow investigators, first, to input the type of strategy of interest to them and, then, to follow the existing 5 modules and obtain step-by-step guidance—from study design through implementation of causal methods. Alternately, researchers can peruse existing case studies to learn how to conduct or design projects based on the types of intervention of interest to them. To increase user-friendliness, all case studies and demos will include YouTube videos in addition to static content. Clicking the case study links in CERBOT will take investigators directly to content relevant to the section that they are currently viewing.
- Enhance the CERBOT reporting feature with better visual displays of tables so that users can directly extract them to include in a research proposal or manuscript.
- Integrate CERBOT with other causal inference-related tools. For example, a recent addition to causal inference methodology is the use of causal diagrams (directed acyclic graphs).65 Although not a data analysis method itself, a causal diagram is used to represent the structure of causal networks linking exposure, outcome, confounders, and other variables; however, it requires an explicit formulation of the relationships among these factors.
- Improve the functionality of CERBOT techniques such as auto-save, enhanced discuss thread, cell phone use, log, and search functions. For example, a partial save function is a useful feature to formulate for future use.
Conclusions
Nonrandomized, or observational, studies are critical in proving cause and effect for treatments and patient outcomes. Such studies provide evidence about real-world populations and practices that complement the information available from RCTs. In some cases, observational studies present data not otherwise available. The research team developed a user-friendly web application to improve CER study design and analysis by means of explicitly emulating a target trial. The causal inference framework, developed by the project co-PI and his colleagues, is essentially the ability to conceptualize observational studies for comparative purposes and as attempts to emulate randomized experiments. Key features of CERBOT include steps that are easy to follow, in order to formulate a detailed study design; recommendations for analytical methods, based on specific types of research questions and specific types of biases and confounding issues (as also recommended by PCORI Methodology Standards); and facilitated teamwork among researchers and stakeholders, who include researchers, clinicians, patients, and others suitable for addressing specific PCOR CER questions.
In the current era of big data, the use of CERBOT—in combination with subject matter expertise, epidemiologic and methodologic proficiency, and innovative computer science tools—can help maximize the societal benefits of big data for causal inference.
References
- 1.
- Stuart EA, Naeger S. Introduction to causal inference approaches. In: Sobolev B, Gatsonis C, eds. Methods in Health Services Research. Springer; 2017. Accessed July 19, 2019. https://link
.springer .com/content/pdf/10 .1007/978-1-4939-6704-9_8-1.pdf - 2.
- Black N. Why we need observational studies to evaluate the effectiveness of health care. BMJ. 1996;312(7040):1215. [PMC free article: PMC2350940] [PubMed: 8634569]
- 3.
- Institute of Medicine. Initial National Priorities for Comparative Effectiveness Research. National Academies Press; 2009.
- 4.
- PCORI Methodology Committee. The PCORI methodology report. Accessed July 19, 2019. https://www
.pcori.org /research-results/about-our-research /research-methodology - 5.
- National Research Council. Frontiers in Massive Data Analysis. National Academies Press; 2013.
- 6.
- Hernán MA, Robins JM. Causal Inference. Chapman & Hall/CRC; 2018.
- 7.
- Richardson WS, Wilson MC, Nishikawa J, et al. The well-built clinical question: a key to evidence-based decisions. ACP J Club. 1995;1233:A12-A13. [PubMed: 7582737]
- 8.
- Hernán MA, Robins JM. Observational Studies Analyzed Like Randomized Trials, and Vice Versa. Chapman & Hall/CRC Press; 2016.
- 9.
- Hernán MA, Robins JM. Using big data to emulate a target trial when a randomized trial is not available. Am J Epidemiol. 2016;183(8):758-764. [PMC free article: PMC4832051] [PubMed: 26994063]
- 10.
- Labrecque JA, Swanson SA. Target trial emulation: teaching epidemiology and beyond. Eur J Epidemiol. 2017;32(6):473-475. [PMC free article: PMC5550532] [PubMed: 28770358]
- 11.
- Schardt C, Adams MB, Owens T, Keitz S, Fontelo P. Utilization of the PICO framework to improve searching PubMed for clinical questions. BMC Med Inform Decis Mak. 2007;7(1):16. [PMC free article: PMC1904193] [PubMed: 17573961]
- 12.
- Dunham R. Nominal group technique: a user's guide. Accessed February 27, 2018. http://instruction.bus.wisc.edu/obdemo/readings/ngt.html [Link no longer works.]
- 13.
- Sample J. Nominal group technique: an alternative to brainstorming. J Ext. 1984;22(2):2IAW2.
- 14.
- Hernán MA, Alonso A, Logan R, et al. Observational studies analyzed like randomized experiments: an application to postmenopausal hormone therapy and coronary heart disease. Epidemiology. 2008;19(6):766. [PMC free article: PMC3731075] [PubMed: 18854702]
- 15.
- Danaei G, Rodríguez LA, Cantero OF, Logan R, Hernán MA. Observational data for comparative effectiveness research: an emulation of randomised trials of statins and primary prevention of coronary heart disease. Stat Methods Med Res. 2013;22(1):70-96. [PMC free article: PMC3613145] [PubMed: 22016461]
- 16.
- Zhang Y, Thamer M, Kaufman J, Cotter D, Hernán M. Comparative effectiveness of two anemia management strategies for complex elderly dialysis patients. Med Care. 2014;52(suppl 3):S132. [PMC free article: PMC3933821] [PubMed: 24561752]
- 17.
- Zhang Y, Young JG, Thamer M, Hernán MA. Comparing the effectiveness of dynamic treatment strategies using electronic health records: an application of the parametric g-formula to anemia management strategies. Health Serv Res. 2018;53(3):1900-1918. [PMC free article: PMC5980367] [PubMed: 28560811]
- 18.
- García-Albéniz X, Hsu J, Bretthauer M, Hernán MA. Effectiveness of screening colonoscopy to prevent colorectal cancer among Medicare beneficiaries aged 70 to 79 years: a prospective observational study. Ann Intern Med. 2017;166(1):18-26. [PMC free article: PMC5417337] [PubMed: 27669524]
- 19.
- Cain LE, Logan R, Robins JM, et al. When to initiate combined antiretroviral therapy to reduce rates of mortality and AIDS in HIV-infected individuals in developed countries. Ann Intern Med. 2011;154(8):509-515. [PMC free article: PMC3610527] [PubMed: 21502648]
- 20.
- Lodi S, Phillips A, Logan R, et al. Comparative effectiveness of immediate antiretroviral therapy versus CD4-based initiation in HIV-positive individuals in high-income countries: observational cohort study. Lancet HIV. 2015;2(8):e335-e343. doi:10.1016/S2352-3018(15)00108-3 [PMC free article: PMC4643831] [PubMed: 26423376] [CrossRef]
- 21.
- Hernán MA. Invited commentary: hypothetical interventions to define causal effects—afterthought or prerequisite? Am J Epidemiol. 2005;162(7):618-620. [PubMed: 16120710]
- 22.
- Thamer M, Hernán MA, Zhang Y, Cotter D, Petri M. Prednisone, lupus activity, and permanent organ damage. J Rheumatol. 2009;36(3):560-564. [PMC free article: PMC3624968] [PubMed: 19208608]
- 23.
- Robins JM, Hernán MA. Estimation of the causal effects of time-varying exposures. In: Fitzmaurice G, Davidian M, Verbeke G, Molenberghs G, eds. Longitudinal Data Analysis. Chapman and Hall/CRC Press; 2008:553-599.
- 24.
- Young JG, Hernán MA, Robins JM. Identification, estimation and approximation of risk under interventions that depend on the natural value of treatment using observational data. Epidemiol Methods. 2014;3(1):1-9. [PMC free article: PMC4387917] [PubMed: 25866704]
- 25.
- Danaei G, Rodríguez LA, Cantero OF, Logan RW, Hernán MA. Electronic medical records can be used to emulate target trials of sustained treatment strategies. J Clin Epidemiol. 2018;96:12-22. [PMC free article: PMC5847447] [PubMed: 29203418]
- 26.
- Hernán MA, Robins JM. Estimating causal effects from epidemiological data. J Epidemiol Community Health. 2006;60(7):578-586. [PMC free article: PMC2652882] [PubMed: 16790829]
- 27.
- Hernán MA, Hernández-Díaz S. Beyond the intention-to-treat in comparative effectiveness research. Clin Trials. 2012;9(1):48-55. [PMC free article: PMC3731071] [PubMed: 21948059]
- 28.
- Murray EJ, Hernán MA. Adherence adjustment in the Coronary Drug Project: a call for better per-protocol effect estimates in randomized trials. Clin Trials. 2016;13(4):372-378. [PMC free article: PMC4942353] [PubMed: 26951361]
- 29.
- Swanson SA, Holme Ø, Løberg M, et al. Bounding the per-protocol effect in randomized trials: an application to colorectal cancer screening. Trials. 2015;16:541. doi:10.1186/s13063-015-1056-8 [PMC free article: PMC4666083] [PubMed: 26620120] [CrossRef]
- 30.
- Jackson JW. Diagnostics for confounding of time-varying and other joint exposures. Epidemiology. 2016;27(6):859-869. [PMC free article: PMC5308856] [PubMed: 27479649]
- 31.
- Cole SR, Hernán MA, Robins JM, et al. Effect of highly active antiretroviral therapy on time to acquired immunodeficiency syndrome or death using marginal structural models. Am J Epidemiol. 2003;158(7):687-694. [PubMed: 14507605]
- 32.
- HIV-Causal Collaboration. When to initiate combined antiretroviral therapy to reduce mortality and AIDS-defining illness in HIV-infected persons in developed countries: an observational study. Ann Intern Med. 2011;154(8):509. [PMC free article: PMC3610527] [PubMed: 21502648]
- 33.
- Naimi AI, Cole SR, Kennedy EH. An introduction to g methods. Int J Epidemiol. 2017;46(2):756-762. [PMC free article: PMC6074945] [PubMed: 28039382]
- 34.
- Funk MJ, Westreich D, Wiesen C, Stürmer T, Brookhart MA, Davidian M. Doubly robust estimation of causal effects. Am J Epidemiol. 2011;173(7):761-767. [PMC free article: PMC3070495] [PubMed: 21385832]
- 35.
- Petersen M, Schwab J, Gruber S, Blaser N, Schomaker M, van der Laan M. Targeted maximum likelihood estimation for dynamic and static longitudinal marginal structural working models. J Causal Inference. 2014;2(2):147-185. [PMC free article: PMC4405134] [PubMed: 25909047]
- 36.
- Swanson SA. Instrumental variable analyses in pharmacoepidemiology: what target trials do we emulate? Curr Epidemiol Rep. 2017;4(4):281-287. [PMC free article: PMC5711965] [PubMed: 29226066]
- 37.
- Greenland S. An introduction to instrumental variables for epidemiologists. Int J Epidemiol. 2000;29(4):722-729. [PubMed: 10922351]
- 38.
- Hernán MA, Robins JM. Instruments for causal inference: an epidemiologist's dream? Epidemiology. 2006;17(4):360-372. [PubMed: 16755261]
- 39.
- Petersen ML. Commentary: applying a causal road map in settings with time-dependent confounding. Epidemiology. 2014;25(6):898-901. [PMC free article: PMC4460577] [PubMed: 25265135]
- 40.
- Cox E, Martin BC, Van Staa T, Garbe E, Siebert U, Johnson ML. Good research practices for comparative effectiveness research: approaches to mitigate bias and confounding in the design of nonrandomized studies of treatment effects using secondary data sources; the International Society for Pharmacoeconomics and Outcomes Research Good Research Practices for Retrospective Database Analysis Task Force Report—Part II. Value Health. 2009;12(8):1053-1061. [PubMed: 19744292]
- 41.
- Petersen ML, Sinisi SE, van der Laan MJ. Estimation of direct causal effects. Epidemiology. 2006;17(3):276-284. [PubMed: 16617276]
- 42.
- Little RJ, D'agostino R, Cohen ML, et al. The prevention and treatment of missing data in clinical trials. N Engl J Med. 2012;367(14):1355-1360. [PMC free article: PMC3771340] [PubMed: 23034025]
- 43.
- Hernán MÁ, Brumback B, Robins JM. Marginal structural models to estimate the causal effect of zidovudine on the survival of HIV-positive men. Epidemiology. 2000;11(5):561-570. [PubMed: 10955409]
- 44.
- Garcia-Albeniz X, Chan JM, Paciorek AT, et al. Immediate versus deferred initiation of androgen deprivation therapy in prostate cancer patients with PSA-only relapse. Eur J Cancer. 2015;51(7):817-824. [PMC free article: PMC4402138] [PubMed: 25794605]
- 45.
- Hernán MA, Lanoy E, Costagliola D, Robins JM. Comparison of dynamic treatment regimes via inverse probability weighting. Basic Clin Pharmacol Toxicol. 2006;98(3):237-242. [PubMed: 16611197]
- 46.
- Klein JP, Rizzo JD, Zhang MJ, Keiding N. Statistical methods for the analysis and presentation of the results of bone marrow transplants. Part 2: regression modeling. Bone Marrow Transplant. 2001;28(11):1001. [PubMed: 11781608]
- 47.
- Baiocchi M, Cheng J, Small DS. Instrumental variable methods for causal inference. Stat Med. 2014;33(13):2297-2340. [PMC free article: PMC4201653] [PubMed: 24599889]
- 48.
- Neugebauer R, van der Laan M. Why prefer double robust estimators in causal inference? J Stat Plan Inference. 2005;129(1-2):405-426.
- 49.
- Zhang Y, Thamer M, Kaufman JS, Cotter DJ, Hernán MA. High doses of epoetin do not lower mortality and cardiovascular risk among elderly hemodialysis patients with diabetes. Kidney Int. 2011;80(6):663-669. [PMC free article: PMC3637948] [PubMed: 21697811]
- 50.
- Daniel RM, De Stavola BL, Vansteelandt S. Commentary: the formal approach to quantitative causal inference in epidemiology: misguided or misrepresented? Int J Epidemiol. 2016;45(6):1817-1829. [PMC free article: PMC5841837] [PubMed: 28130320]
- 51.
- Hernán MA, Sauer BC, Hernández-Díaz S, Platt R, Shrier I. Specifying a target trial prevents immortal time bias and other self-inflicted injuries in observational analyses. J Clin Epidemiol. 2016;79:70-75. [PMC free article: PMC5124536] [PubMed: 27237061]
- 52.
- Ray WA. Evaluating medication effects outside of clinical trials: new-user designs. Am J Epidemiol. 2003;158(9):915-20. [PubMed: 14585769]
- 53.
- Hernán MA. With great data comes great responsibility: publishing comparative effectiveness research in epidemiology. Epidemiology. 2011;22(3):290. [PMC free article: PMC3072432] [PubMed: 21464646]
- 54.
- García-Albéniz X, Hsu J, Hernán MA. The value of explicitly emulating a target trial when using real world evidence: an application to colorectal cancer screening. Eur J Epidemiol. 2017;32(6):495-500. [PMC free article: PMC5759953] [PubMed: 28748498]
- 55.
- Moura LM, Westover MB, Kwasnik D, Cole AJ, Hsu J. Causal inference as an emerging statistical approach in neurology: an example for epilepsy in the elderly. Clin Epidemiol. 2017;9:9. [PMC free article: PMC5221551] [PubMed: 28115873]
- 56.
- Cain LE, Saag MS, Petersen M, et al. Using observational data to emulate a randomized trial of dynamic treatment-switching strategies: an application to antiretroviral therapy. Int J Epidemiol. 2016;45(6):2038-2049. [PMC free article: PMC5841611] [PubMed: 26721599]
- 57.
- Altman DG, Simera I, Hoey J, Moher D, Schulz K. EQUATOR: reporting guidelines for health research. Open Med. 2008;2(2):e49. doi:10.1016/S0140-6736(08)60505-X [PMC free article: PMC3090180] [PubMed: 21602941] [CrossRef]
- 58.
- Gallo V, Egger M, McCormack V, et al. STrengthening the Reporting of OBservational studies in Epidemiology: Molecular Epidemiology STROBE-ME; an extension of the STROBE statement. J Epidemiol Community Health. 2012;66(9):844-854. [PubMed: 22025194]
- 59.
- Des Jarlais DC, Lyles C, Crepaz N; TREND Group. Improving the reporting quality of nonrandomized evaluations of behavioral and public health interventions: the TREND statement. Am J Public Health. 2004;94(3):361-366. [PMC free article: PMC1448256] [PubMed: 14998794]
- 60.
- Dreyer NA, Schneeweiss S, McNeil BJ, et al. GRACE principles: recognizing high-quality observational studies of comparative effectiveness. Am J Manag Care. 2010;16(6):467-471. [PubMed: 20560690]
- 61.
- Berger ML, Mamdani M, Atkins D, Johnson ML. Good research practices for comparative effectiveness research: defining, reporting and interpreting nonrandomized studies of treatment effects using secondary data sources; the ISPOR Good Research Practices for Retrospective Database Analysis Task Force Report—Part I. Value Health. 2009;12(8):1044-1052. [PubMed: 19793072]
- 62.
- Annual meeting of the Society for Epidemiologic Research. Society for Epidemiologic Research. Accessed July 19, 2019. https://epiresearch
.org/annual-meeting/ - 63.
- Conference and workshops on causal inference methods for PCOR using observational data. Patient-Centered Outcomes Research Institute (PCORI). Accessed July 19, 2019. https://www
.pcori.org /research-results/2015 /conference-and-workshops-causal-inference-methods-pcor-using-observational - 64.
- Ahern J, Hubbard A, Galea S. Estimating the effects of potential public health interventions on population disease burden: a step-by-step illustration of causal inference methods. Am J Epidemiol. 2009;169(9):1140-1147. [PMC free article: PMC2732980] [PubMed: 19270051]
- 65.
- Shrier I, Platt RW. Reducing bias through directed acyclic graphs. BMC Med Res Methodol. 2008;8(1):70. [PMC free article: PMC2601045] [PubMed: 18973665]
Acknowledgment
Research reported in this report was [partially] funded through a Patient-Centered Outcomes Research Institute® (PCORI®) Award (#ME-1303-6031) Further information available at: https://www.pcori.org/research-results/2013/developing-interactive-online-guide-support-use-causal-inference-methods
Suggested citation:
Zhang Y, Thamer M, Kshirsagar O, Hernan M. (2019). Developing an Interactive Online Guide to Support the Use of Causal Inference Methods in Comparative Effectiveness Research. Patient-Centered Outcomes Research Institute (PCORI). https://doi.org/10.25302/1.2020.ME.13036031
Disclaimer
The [views, statements, opinions] presented in this report are solely the responsibility of the author(s) and do not necessarily represent the views of the Patient-Centered Outcomes Research Institute® (PCORI®), its Board of Governors or Methodology Committee.
- Developing an Interactive Online Guide to Support the Use of Causal Inference Me...Developing an Interactive Online Guide to Support the Use of Causal Inference Methods in Comparative Effectiveness Research
Your browsing activity is empty.
Activity recording is turned off.
See more...