NCBI Bookshelf. A service of the National Library of Medicine, National Institutes of Health.
A Proof-of-Concept Case Study Integrating Publicly Available Information to Screen Candidates for Chemical Prioritization under TSCA [Internet]. Washington (DC): U.S. Environmental Protection Agency; 2021 Jun.
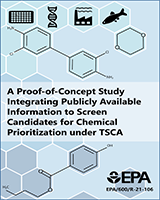
A Proof-of-Concept Case Study Integrating Publicly Available Information to Screen Candidates for Chemical Prioritization under TSCA [Internet].
Show detailsA subset of the TSCA active inventory was selected to test the PICS approach in a proof-of-concept (POC) case study. The subset of chemical substances was designed to evaluate the impact of various workflows across the scientific domains and gauge the impact of different modifying criteria on information availability. The chemical substances (described in further detail below) focused on including a broad range of chemical substances with varying levels of available information and included the initial proposed set of 20 high26- and 20 low priority27 chemical substances. The PICS approach is intended to work broadly across the chemical substance landscape, but this case study was designed for particular application within the TSCA active inventory. Following the development of this approach, the PICS approach can also be applied to the broader TSCA active inventory or adapted to other decision contexts.
The POC case study was also used to develop standard operating procedures (SOPs) for quality control (QC) analysis of the large, Type 1 datasets28 required to apply the approach to the TSCA active inventory. The SOPs are being implemented in an internal software system to assess, track and correct any discrepancies between data used as input and the source documents or files from which these data were obtained. The QC analysis was mainly focused on the data accuracy, and not determining the study or data quality. Further review of data and study quality would be performed by experts outside of the PICS approach prior to chemical substance selection. The QC software system being developed will also accommodate in-depth, expert review of studies for selected chemical substances and/or studies with summary values that trigger QC review.
5.1. Chemical Substance Selection, Curation and Quality Control
Chemical Structure and Identifier Mapping
Chemical substances on the non-confidential TSCA active inventory were downloaded from the EPA website29. Chemical substances without a CAS Registry Number were removed, and the remaining chemical substances mapped to Distributed Structure-Searchable Toxicity (DSSTox) substance identifiers (DTXSIDs) in the ChemReg chemical registration system30, a database underpinning the CompTox Chemicals Dashboard31,32. Any chemical substances with conflicts between the TSCA identifiers and DSSTox records (e.g., discrepant CAS numbers or chemical substance names) were placed in a queue for mapping review by trained chemists. The mapped, non-confidential TSCA active inventory contained ~33,000 chemical substances and 25,275 with structural information visible via the CompTox Chemicals Dashboard33.
Chemical Substance Selection
Chemical substances for the POC case study were selected from the mapped, non-confidential TSCA active inventory by the scientific experts designing the domain specific workflows and included:
- Initial proposed set of 20 high- and 20 low priority candidate chemical substances along with the initial first ten TSCA Work Plan chemical substances selected for evaluation in 2016 (TSCA10)34;
- Chemical substances from the 2014 update to the TSCA Work Plan (TSCA90)35;
- Chemical substances with well-studied effects in each of the scientific domains;
- A subset of chemical substances listed in the Food and Drug Administration’s (FDA) Substances Added to Food inventory (formerly Everything Added to Foods in the United States list) and EPA’s Safer Choice Safer Chemical Ingredients List (SCIL).
From these lists, a total of 238 chemical substances, called the POC238 (listed in Appendix A), was compiled for the development of workflows and metrics for each of the seven scientific domains36. The POC238 contains some chemical substances for which an expected biological response in one or more of the separate domains would serve as a reference for evaluation of how accurately the PICS approach identified potential hazards or environmental concerns. The POC238 was selected to span a range in the degree of potential concern and information availability; however, the overall information availability for the selected chemical substances was generally higher than for the overall TSCA active inventory (see Figure 14).
Chemical Substance Information Extraction and Quality Control
A key component of the PICS approach is the curation of data collected from “Type 1” data sources (as defined in the Working Approach for Potential Candidates). Type 1 data sources are publicly available and readily searchable, enabling data extraction in a structured form. Hazard, exposure, persistence, and bioaccumulation information was extracted from a range of Type 1 sources (listed in Appendix B). Curated traditional and NAM data were compiled and filtered prior to being analyzed for QC. More details on specific filtering of information sources for the individual workflows are described below.
QC analysis was performed on the data for the POC238 chemical substances to ensure the curation accuracy from primary published sources to database repository format, inform the development of formal quality assurance (QA) procedures, and obtain information on the scope and resources needed to perform QC for the entire active TSCA inventory. Specific approaches and considerations for the QC review are provided in Appendix C. The QC analysis focused on a determination of accuracy of extraction of the information from the Type 1 sources and did not examine or evaluate study conclusions. Additionally, no study quality considerations were evaluated during QC review. Reviewers did not perform a critical analysis of experimental design, statistical analyses, or data interpretation. Rather, reviewers compared the aggregated Type 1 data to primary and secondary sources. Reviewers flagged data that could not be confirmed to the primary source, even if the aggregated data matched the secondary source. However, certain secondary sources, such as the ECOTOX knowledgebase, the Integrated Risk Information System (IRIS), or chemical exposure data have existing QC or peer review processes. For these select databases, confirmation to secondary source was sufficient to pass QC review.
Over 25,000 total records were identified, with nearly 17,000 data points (68%) associated with primary sources. For this effort, a data point was deemed ‘reviewed’ if it matched the number in the authoritative secondary source; primary source review was not required. As an example, a point of departure (POD) extracted from the Integrated Risk Information System (IRIS) would have been confirmed against the on-line IRIS database, but not tracked back to the source paper for the POD. POD matching required that the chemical identity, POD value, and relevant metadata (e.g., units, exposure route, species) were consistent. The case study developed methods for data aggregation, curation, and evaluation, as well as QA recommendations to efficiently review Type 1 datasets.
5.2. Scientific Domain Metric Assessment
A comprehensive analysis of the publicly available information for the POC238 chemical substances was performed following data curation and QC. The overall SDM is determined by summing the results from the individual scientific domain workflows described below for the following domains: (1) human health hazard relative to exposure; (2) ecological hazard; (3) carcinogenicity; (4) genotoxicity; (5) susceptible populations; (6) persistence and bioaccumulation; and (7) skin sensitization and skin/eye irritation (Figure 3). Of the seven domains used in the PICS approach, five were included in the previous Working Approach. The additional two domains (carcinogenicity; skin sensitization and skin/eye irritation) were included in the PICS approach based on their use in the 2014 TSCA Work Plan. Each of these workflows represent a mechanism for making a determination of potential concern for a compound in each domain based on publicly available data. These domains were selected based on their importance to understanding human health and ecological hazard, human exposure (including susceptible populations), past use in TSCA prioritization activities37, and/or the statutory language in the Frank R. Lautenberg Chemical Safety for the 21st Century Act (P.L. 114–182)38.
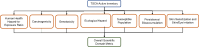
Figure 3
The seven scientific domains used to evaluate the degree of potential concern related to human health and the environment for each chemical substance. The overall SDM is the sum of the individual workflows within each domain.
Human Health Hazard-to-Exposure Ratio Domain
The identification of 2014 TSCA Work Plan chemical substances included consideration of human health hazard as well as information on exposure potential (TSCA 2012)39. As outlined in the Working Approach for Potential Candidates, the workflow described for this domain proposes the use of ratios of hazardous effect dose-response (e.g., point-of-departure) information to exposure predictions. As point-of-departure doses for hazardous effects approach exposure predictions, a greater degree of potential concern may be indicated, whereas doses for hazard and exposure separated by many orders of magnitude may suggest a lower degree of potential concern.
The calculation of the human health hazard-to-exposure ratio (HER) domain metric is based on a workflow that incorporates a tiered selection of hazard information as well as exposure estimates from the EPA ExpoCast (Exposure Forecasting) modeling effort (Figure 4)40. The third generation ExpoCast Systematic Empirical Evaluation of Models (SEEM3) exposure model41 is a meta-model for aggregate population median dose intake rate and incorporates twelve different exposure predictors covering sources that are near42- and far-field43. Four distinct source-based exposure pathways were considered: non-pesticide dietary, consumer products, far-field chemical, and far-field industrial. Chemical substances with other exposure pathways are outside of the domain of the models and are noted with an information gathering (IG) flag. IG flags are used to bring attention to specific aspects of the workflow decisions that may impact the results and may denote whether the data falls within the applicability domain of the model. SEEM3 is calibrated using chemical substance intake rates from biomonitoring data from the Centers for Disease Control and Prevention (CDC) National Health and Nutrition Examination Survey; (NHANES)44; and are used in place of the SEEM3 predictions45. As further described in Ring et al. 201746, NHANES was used as a more comprehensive dataset which allowed for incorporation of interindividual variability, including across different demographics. PODs from dose-response curves from traditional in vivo toxicity studies are divided by the median ExpoCast intake rate estimate to provide a HER. The approach uses only POD values with units of mg/kg-bw/day from repeat dose studies (including multiple specific toxicities, e.g., reproductive toxicity). Therefore, the majority of included studies assessed the oral route of exposure, although other routes of exposure were included if the units had been converted appropriately (e.g., inhalation exposure concentration converted to an equivalent mg/kg-bw/day dose). The POD used for HER calculation was either the minimum of the set, or if a human health-relevant POD estimate from an authoritative regulatory agency was available (ATSDR, EFSA, EPA HEAST, EPA OPP, EPA IRIS or EPA PPRTV), it was used in the analysis. When in vivo studies are not available, in vitro bioactivity estimates from ToxCast are converted into an oral dose equivalent using high-throughput toxicokinetic (HTTK) approaches47,48 (called the in vitro-to-in vivo extrapolation (IVIVE) POD) and divided by the ExpoCast exposure estimate to provide a bioactivity-to-exposure ratio (BER)49,50. Finally, when neither in vivo nor in vitro studies are available, the most relevant threshold of toxicological concern (TTC) value is assigned when appropriate and divided by the ExpoCast exposure estimate to provide a TTC-to-exposure ratio (TER)51. Note that TTC values are in silico NAMs derived using the Toxtree software application (Ideaconsult Ltd)52 by calculating the lower 95th-percentile POD for each of the classes of chemical substances considered, and then applying a safety factor of 100. In the current application, this safety factor is removed because lack of in vivo data is accounted for separately in the IAM. From a practical standpoint, if this safety factor was left in place, a vast majority of chemical substances with only a TTC value would be designated as high concern, regardless of exposure level.
Human Health Hazard-to-Exposure Evaluation
A human HER domain metric is assigned in a tiered fashion based on the magnitude of the HER, BER, or TER value. The order of preference is HER > BER > TER (i.e., if the HER is available, it is used preferentially over BER and TER). For volatile substances, PODs from traditional in vivo repeat dose toxicity studies that have units converted to mg/kg-bw/day are utilized, followed by IVIVE POD estimates using in vitro bioactivity data from ToxCast and toxicokinetic estimates from HTTK. EPA does not initially incorporate TTC values for volatile substances since well-established TTC values for the inhalation route of exposure are not yet available.
For each chemical, each metric was assigned a value in the range from 1 to 4, to allow combining the metrics in a consistent way. This is adapted from the strategy used in the 2012 TSCA Work Plan Methodology53. Most of the metrics naturally fell into discrete categories from low concern=1 to high concern=4. For the HER and ecological hazard domains (see below) we converted the continuous value to this scale using Formula 1:
Note that the maximum and minimum values are taken across all chemicals with HER values so that the domain metric is scaled relative to HER values. The first term (log10 (HER/BER/TER)) uses an HER for a chemical, if available, followed by BER and TER. This sets the domain metric of the chemical with the lowest HER (highest concern) to a value of 4 and sets the domain metric of the chemicals with the highest HER (lowest concern) to 1 (Table 1). The minimum and maximum HER values will be somewhat sensitive to the set of chemicals included, but these values are taken from the 2838 out of the TSCA active inventory with value for either HER, BER or TER. The largest HER is 5.03x1014 (1,2,5,6,9,10-hexabromocyclododecane) and the smallest value is 0.89 (ethenylsilanetriyl triacetate). A value of 0 is given in the absence of information and the substance is flagged for future information gathering. The in vivo hazard data is derived from the EPA ToxValDB database. The in vitro ToxCast data is obtained from the EPA invitroDB database. These datasets as well as the toxicokinetic data parameters are publicly available through the EPA CompTox Chemicals Dashboard54.
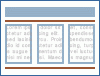
Table 1
Criteria used to calculate the human hazard to exposure ratio domain metric.
Limitations and Longer-term Options
When only data from acute in vivo studies was available, the data were not considered sufficient for calculation of the HER, which uses hazard information from in vivo repeat dose studies, including studies for specific endpoints including reproductive and developmental toxicology. (Note though that the presence or absence of acute data is included in the IAM, described below.) Ongoing research will be needed to determine how to utilize acute toxicity information in the absence of repeat dose toxicity information to estimate a POD for HER calculation. In the current implementation, POD values with typical inhalation units (mg/m3 or ppm) have been excluded. Converting these inhalation values to the oral equivalent dose value requires, at a minimum, knowing whether the chemical substance has local or systemic effects. This information is not typically captured in the current Type 1 information sources and will require either manual curation of the relevant studies, or development of a semi-automated approach to select the appropriate exposure effect class. Similarly, conversion of dermal exposure was not addressed for this case study. Future efforts could incorporate data from these additional routes of exposure.
Calculation of the BER is influenced by selection of a minimum in vitro potency value from high-throughput bioactivity screening data and the HTTK approaches used to derive an administered equivalent dose. Although this is an area of ongoing research, current evidence supports that this global bioactivity approach is conservative55 and that further efforts to refine may provide additional pathway specific PODs (increase relevance). In the POC, features of the concentration-response curves fit to the ToxCast high-throughput bioactivity data have been used to identify a minimum potency value showing bioactivity. In future iterations, we propose leveraging ongoing research on how to best identify the minimum credible in vitro potency values from ToxCast and other sources of high-throughput bioactivity data (e.g. high-throughput transcriptomics), as well as ongoing and iterative improvements in HTTK modeling. Selected choices in HTTK modeling approaches can also include a consideration of interindividual toxicokinetic variability, or not, depending on the scenario; in a conservative approach to an initial screening of substances, use of estimated parameters for toxicokinetically-susceptible individuals to derive administered equivalent doses may be informative.
Additionally, QSAR models were considered to estimate in vivo PODs as a fourth level in the hazard estimation process (in vivo>IVIVE>QSAR>TTC), but at the time of development, a valid QSAR model was not available. Finally, the current TTC values are limited to oral exposures. We are reviewing the latest research efforts related to the use of TTC for other routes of exposure, and any future improvements to this approach may expand the domain of applicability of the TTC to incorporate these updates such as in Nelms and Patlewicz (2020)56. This may also help to address limitations of this approach related to potential screening of compounds for which in vitro assays (the basis of BER) or TTC (the basis of TER) do not perform well.
Carcinogenicity Domain
The probable or known carcinogenicity of a chemical substance was considered in selecting the 2014 TSCA Work Plan chemical substances57. Carcinogenicity was not included as a separate domain in the previous Working Approach for Potential Candidates, due to limited availability of Type 1 carcinogenicity data sources. In the Working Approach for Potential Candidates, the genotoxicity domain was considered as a surrogate for carcinogenicity. In the PICS approach, carcinogenicity and genotoxicity are included as separate domains due to the fact that carcinogenicity may be associated with non-genotoxic as well as genotoxic mechanisms.
The ability of an agent to cause cancer in humans is typically assessed using a weight-of-evidence approach, considering exposure, epidemiology, animal cancer data, and mechanistic data, including genotoxicity and pharmacokinetic/pharmacodynamic information. Major national and international organizations convene expert panels to perform these evaluations, resulting in authoritative assessments of the potential of agents to induce cancer in humans (e.g., IARC, IRIS). EPA has its own guidelines for cancer that consider mechanistic data as an important component of carcinogenicity58. In the absence of such evaluations for human cancer, the ability of the agent to cause cancer in in vivo rodent models is an indication of the potential of an agent to be carcinogenic to humans. Rodent chronic bioassays include the standard protocols established by organizations such as the National Toxicology Program (NTP)59, as well as more generalized guidance from institutions such as the Organization for Economic Cooperation and Development (OECD)60. These data have been compiled in ToxValDB. The presence of lesions believed to have resulted from carcinogenesis were used in a binary fashion in the scoring process. That is, the potency of the carcinogen is not reflected in the evaluation process, just the evidence of carcinogenicity (yes or no) (Figure 5).
Carcinogenicity Evaluation
The carcinogenicity domain metric is determined from a two-tiered evaluation workflow of the available carcinogenicity data in humans and/or animals. Chemical substances that have not been evaluated for their ability to cause cancer in humans, or where there are no available human data, are evaluated for the ability to cause cancer in animals. This is a binary result, not based on the dose required to produce carcinogenicity, but based on the presence or absence of carcinogenicity in a study (Figure 5/Table 2). Chemical substances with evidence of known human carcinogenicity as determined by an authoritative source are given a value of 4; chemical substances that have been determined to have evidence as possible or probable human carcinogens are given a value of 3; chemical substances that have been shown to cause cancer in animals but have not otherwise been assessed for their ability to cause cancer in humans are given a value of 2; and chemical substances with evidence indicating a low likelihood of carcinogenicity in either humans or rodents based on negative data (e.g., a negative rodent cancer bioassay) are given a value of 1. This category may also be termed as having inadequate or insufficient evidence of carcinogenicity in an authoritative carcinogenesis assessment. A value of 0 is given in the absence of data and an information gathering flag is included.
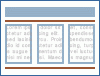
Table 2
Criteria used to calculate the carcinogenicity domain metric.
Limitations and Longer-term Options
There are limited data available for carcinogenicity of chemical substances. One limitation of this approach is the lack of a published peer-reviewed automated predictive model for the determination of carcinogenicity. OncoLogic™ 61, a computer system that evaluates the carcinogenic potential of chemical substances, has not yet been modified to analyze large numbers of chemical substances in a manner that can be readily incorporated into this approach. In the future, the OncoLogic system could be adapted to meet this need and could be incorporated into this workflow in a tiered manner. Indeed, there is an activity within the OECD Toolbox Management Group which is investigating the feasibility of implementing the decision logic of selected OncoLogic chemical classes into the OECD Toolbox. Currently, OncoLogic is used as part of the expert review of compounds and incorporated into the weight-of-evidence assessment of specific compounds.
Genotoxicity Domain
Genotoxicity is an important component of understanding chemical substance hazards. Genotoxicity refers to the ability of agents to induce DNA damage, such as DNA strand breaks or DNA adducts, as well as the ability to induce mutations, i.e., heritable changes in DNA sequence. In the absence of carcinogenicity data, genotoxicity is often used as a surrogate. This document evaluates chemical substances for genotoxicity by considering data from assays that collectively detect mutations in bacteria or mammalian cells, as well as DNA damage in mammalian cells or rodents. For the PICS approach, some consideration was given to including genotoxicity within the same domain as carcinogenicity. However, it was determined that these should be considered separately to incorporate not only the impact of nongenotoxic carcinogens, but also capture genotoxic chemical exposures that may not have been assessed for cancer.
Since the initial EPA implementation of TSCA in 1976, many studies have assessed which combinations of genotoxicity tests are the most predictive62, resulting in testing schemes recommended by the OECD Genetic Toxicology Test Guidelines63, the International Conference on Harmonization64, and the NTP65. Additional consideration has been given to entirely new testing approaches, which do not rely on traditional assays66,67.
Most regulatory bodies in the U.S., such as the EPA and FDA, recommend the OECD genetic toxicology guidelines. This testing includes a set of bacterial assays for gene mutation using strains of Salmonella (the Ames strains) and strains of Escherichia coli WP2 and assays for chromosomal mutation (in vitro chromosome aberration assay, mouse bone-marrow micronucleus assay, and the mouse lymphoma Tk+/- assay). The combination of assays identifies genotoxic agents that produce primarily gene mutations, chromosomal mutations or both gene and chromosomal mutations. A smaller number of chemical substances produce aneuploidy (chromosome gain or loss), which is also detected by the chromosome aberration (CA) or mouse bone-marrow micronucleus assays (MNT).
In the PICS approach, we considered that the genotoxicity of an agent can be sufficiently assessed by evaluating data in the standard bacterial mutation assays (the Ames Salmonella and E. coli WP2 strains) and the three principal assays for chromosomal mutation (in vitro chromosome aberration assay, mouse bone-marrow micronucleus assay, and the mouse lymphoma Tk+/- assay). The selection of a subset of genotoxicity assays used in this approach was based on recent work by Williams et al. 201968 which demonstrated that this subset of assays is sufficient to identify 99% of mutagens tested.
In the absence of experimental data, genotoxicity may be predicted using in silico Quantitative Structure-Activity Relationship (QSAR) models for Ames mutagenicity or in silico structural alerts for clastogenicity. This evaluation is similar for measured data but is tagged with an IG flag for predicted data. The EPA Toxicity Estimation Software Tool (TEST) was used to predict Ames mutagenicity, along with the OECD Toolbox, which includes DNA alerts for Ames, CA and MNT; protein binding alerts for CA; in vitro mutagenicity (Ames test) alerts by Instituto Superiore di Sanita (ISS), and in vivo mutagenicity (micronucleus) alerts by ISS. The Ames mutagenicity module within the TEST software is based on a dataset of 6,512 chemical substances that was compiled from several different sources as described in Hansen et al.69. After removal of salts, mixtures, ambiguous compounds, and compounds without CAS numbers, the final dataset consisted of 5,743 chemical substances. Several different approaches were used to derive TEST predictions, including a hierarchical-clustering approach70, a nearest-neighbor approach, a Food and Drug Administration approach, and a single-model approach. The profilers within the OECD Toolbox are collections of structural alerts that have been compiled and developed by various researchers and organizations. Most of the profilers incorporate the alerts devised by Ashby and Tennant (1991)71, but additional alerts are included depending on the experimental data available. DNA_OASIS profilers include alerts derived from the training sets used for the TIMES expert system, whereas the ISS alerts rely on the ISSCAN72 database. A consensus outcome from the individual models culminates in the overall prediction conclusion generated for a given chemical substance.
Genotoxicity Evaluation
The process for screening chemical substances for genotoxicity is shown in Figure 6/Table 3. Chemical substances with evidence of genotoxicity are evaluated based on results for gene mutation in bacterial mutagenicity assays and any of the three assays for chromosomal mutations (clastogenicity as described above). Chemical substances that have been determined to be genotoxic experimentally (either as a gene or chromosomal mutagen) are given a value of 4; chemical substances that are predicted to be genotoxic are given a value of 3; chemical substances with inconclusive data are given a value of 2; chemical substances with data showing that the chemical substance is not likely to be genotoxic are given a value of 1; and chemical substances with no data are given a value of 0. Chemical substances with inconclusive results are also tagged with an IG flag. If there are multiple data sources for a chemical substance, the highest metric is selected, e.g., if a chemical substance is a predicted clastogen (metric of 3) and a mutagen based on measured data (metric of 4), an overall metric of 4 was used. No attempts were made to evaluate the quality and design of the studies, and the IG flag gives the end-user an opportunity to evaluate the weight-of-evidence in situations that are deemed inconclusive. Combinations of the Ames or clastogenicity information was used to determine the genotoxicity, which was converted to a numeric scale as described in Table 3.

Figure 6
Tiered evaluation process associated with the genotoxicity domain. The process evaluates the potential mutagenicity and DNA damaging potential of a chemical substance as well as the potential clastogenicity. IG, information gathering.
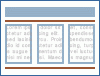
Table 3
Criteria used to calculate the genotoxicity domain metric.
Limitations and Longer-term Options
A limitation of the genotoxicity analysis was the reliance on secondary source data for specific genotoxicity endpoints. The sources included authoritative assessments, data compilation summaries, and publicly available review papers. For the purposes of the automated PICS approach, secondary data sources were deemed acceptable but given an IG flag so that the expert reviewer would have an awareness of the potential limitations of the data sources. Although weight-of-evidence results from authoritative sources may be considered, this approach does not perform a weight-of-evidence analysis for genotoxicity. For many of the chemical substances in the database, there were genotoxicity data available from a variety of data sources. This screening approach does not explicitly address the absolute number of positive or negative results or contradictory results. These issues should be considered during the downstream expert review of any candidate chemical substances (Figure 2). Similarly, a recent effort to develop a genotoxicity hazard assessment framework using in silico tools was published73. This method was developed to rapidly assess chemical hazard but is not designed as an automated approach for analysis of a large number of chemicals at one time. While the published approach goes further to incorporate expert review, many of the underlying assumptions on incorporating specific genotoxicity assays support decisions made in the PICS approach. Longer-term options should also include recent advances in the development of a consensus model using combinations of QSAR models and structural alert predictions to strengthen the use of predictive results in the genotoxicity assessment of compounds74.
Ecological Hazard Domain
The ecological hazard domain is intended to account for potential toxicity to a broad diversity of wildlife and plants. Under typical approaches for ecological hazard classification of chemical substances, for example under the Globally Harmonized System (GHS)75, only aquatic toxicity is considered. However, it is common to consider data from at least three trophic levels of organisms, generally a fish, an invertebrate (crustacean), and at least one plant or algae species76. In cases where data for all three trophic levels are unavailable, additional uncertainty factors are often applied to account for the fact that potentially sensitive classes of organisms with highly distinct life histories and physiology have not been considered. Consistent with GHS, experimentally-derived test data are preferred for derivation of the ecological hazard metric. However, in cases where no experimentally derived PODs are available, QSAR models are used. This well-established method for evaluating ecological hazards is the basis of the workflow developed for the ecological hazard domain of the PICS approach.
The ecological hazard domain is a hazard-only approach based on measured or estimated chronic and acute aquatic toxicity. In vivo aquatic toxicity data are collected from US EPA’s ECOTOX Knowledgebase77, eChemPortal78, and EFSA79. In cases where in vivo data are absent, QSAR-based predictions of aquatic acute toxicity are derived from EcoSAR80 or TEST81. All data are compiled into ToxValDB.
In the PICS approach (Figure 7), additional uncertainty factors are not applied when the three trophic levels typically considered in GHS classification82 are not represented in the dataset. However, there is a computational evaluation of the presence/absence of data for each trophic level. In cases where one or more trophic levels of organisms are not represented, an alert is provided in the form of an information gathering flag. This alert provides an indication that at least one major group of potentially sensitive taxa are not currently considered as part of the ecological hazard domain metric.
Ecological Hazard Evaluation
An ecological domain metric is based on the lowest POD value derived from available in vivo data (acute and chronic; any endpoint or life stage) or QSAR predictions when in vivo POD estimates are unavailable (Figure 7). Using the same rationale as developed for GHS classification, in vivo acute values (expressed as LC50 or LD50) are divided by a factor of 10 to derive a final in vivo POD estimate (PODin vivo). Chronic toxicity data (e.g., reported as NOEC, LOEC, NOEL, LOEL, NOAEL, LOAEL) and QSAR-based estimates are used unadjusted. The minimum PODQSAR value is the minimum across all EcoSAR and TEST predictions (including both acute and chronic models). In general, the chronic values are lower than the acute values, so chronic QSAR values are most often used. The resulting minimum PODin vivo across all three categories are then compared with the ecological hazard domain metric table (Table 4) to assign the final value on a scale of 1–4 (low hazard to very high hazard). A value of 1 is also given when the POD is greater than the water solubility of the chemical84. In the absence of in vivo data, the minimum PODQSAR is selected. The domain metric was calculated in the same way as for human HER, i.e. by scaling the continuous chemical level POD values onto the 1–4 scale, using Formula 2:
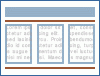
Table 4
Criteria used to calculate the ecological hazard domain metric.
Here the PODmin and PODmax are the minimum and maximum PODs across all chemicals. As with HER, this assigns a metric value of 4 to the chemical with lowest POD and a metric value of 1 to the chemical with the largest POD. The minimum and maximum POD values will be somewhat sensitive to the set of chemicals included, but these values are taken from the 5031 out of the TSCA active inventory with in vivo PODs for either acute or chronic aquatic studies. The largest POD is 163,709 mg/L (phosphonic acid, [1,6-hexanediylbis [nitrilobis(methylene)]]tetrakis) and the smallest value is 1.6x10-19 (propanoic acid, 3-(dodecylthio)-, 2,2-bis[[3-(dodecylthio)-1-oxopropoxy]methyl]-1,3-propanediyl ester).
Limitations and Longer-term Options
One of the limitations of the ecological hazard domain metric is that, unlike the human hazard-to-exposure ratio metric, the ecological hazard domain metric is solely based on hazard, without consideration of exposure. This is primarily due to limited peer-reviewed, automated ecological exposure estimation tools. While procedures to derive ecological exposure estimates are routinely implemented, those approaches cannot currently be automated for thousands of chemical substances. Consequently, the development of an appropriate automated framework for ecological exposure estimation is viewed as a high priority for long-term improvement of the ecological domain metric. If appropriate ecological exposure estimates could be generated or obtained in an automated fashion, the ecological hazard domain metric could be adapted to use a hazard-to-exposure ratio approach that parallels that used in the human health domain described earlier.
A second limitation of the current ecological hazard domain metric is that it only considers aquatic ecotoxicity data. Terrestrial ecotoxicity data for mammals is considered in the human health hazard domain. However, ecotoxicity data for other terrestrial organisms (e.g., amphibians, birds, reptiles, earthworms, insects, terrestrial plants) are not considered which is consistent with the GHS approach85. The 2012 TSCA Work Plan Chemical substances methods86 only considered aquatic ecotoxicity data in deriving a hazard metric. The greater reliance on aquatic ecotoxicity data over terrestrial ecotoxicity data is based in part on the assumption that the aquatic compartment is maximally vulnerable as a final receiving environment for many chemical substances. Aquatic organisms are continuously immersed in the aqueous exposure media and tend to have high exposure levels87. The focus on aquatic species is also based in part on the greater availability of aquatic ecotoxicity data and the availability of well-established QSARs for predicting aquatic toxicity.
Finally, consistent with the GHS approach, experimentally-derived PODs are preferred over QSAR predictions. This is expected to be robust when a modest number of experimentally-derived POD values are available. However, in cases where the experimental data are sparse, one or a few poorly designed studies could lead to underestimation of hazard. Given that the PICS approach is intended to assist in efficiently selecting chemical substances for subsequent expert review, it is assumed that study quality and data sufficiency would be evaluated in the expert-driven part of the process.
Susceptible Human Population Domain
The modernization of TSCA included a requirement for increased attention to susceptible subpopulations, such as infants, children, pregnant women, workers, or the elderly. In the context of TSCA, a “potentially exposed or susceptible subpopulation” is defined as a group of individuals within the general population who, due to either greater susceptibility or greater exposure, may be at greater risk than the general population of adverse health effects from exposure to a chemical substance or mixture. Currently, children are the only susceptible subpopulation given separate consideration in the PICS approach, although additional subpopulations (e.g., pregnant women, elderly) could be incorporated in the future if appropriate to the decision context.
Children may have higher exposure levels to environmental chemical substances than adults, and life-stage dependent exposure sources and pathways can contribute to this differential88. Infants and young toddlers have unique exposure sources such as breast milk and formula. Children play close to the ground, and thus increased contact with the floor and a lower height of the breathing zone results in increased exposure to chemical substances in dust and to chemical substances emitted from flooring or from products applied to floors. Children display increased hand and object mouthing behaviors, and thus can be more highly exposed to chemical substances in consumer products applied to the body, residential surfaces, or in articles such as toys. Children can also be more heavily exposed to environmental pollutants than adults due to physiological factors; they consume more food and water and have higher inhalation rates per pound of body weight than adults89.
Susceptible Population Evaluation
A susceptible population domain metric was developed that characterizes the potential for differential exposure between children and the general population (Figure 8/Table 5). The proposed susceptible subpopulation domain metric incorporates exposure from multiple sources that contribute to an increased exposure of children relative to the general population (Figure 8). Each exposure source is given an exposure differential score to semi-quantitatively represent the magnitude of potential exposure differential between children and adults. Each chemical is assessed using available data to determine whether it occurs in each exposure source and is evaluated accordingly. The exposure source definitions, the data sources used to identify associated chemicals, and the mechanism(s) by which the exposure source contributes to increased exposure for children are summarized in Appendix D. These definitions are consistent with the pathways used by ORD in high-throughput models of exposure90. Information in EPA’s Chemical and Product (CPDat)91 and Chemical Product Category (CPCat)92 databases or in EPA Chemical Data Reporting (CDR) is used to determine which exposure sources are relevant for each chemical substance. CPDat and CPCat contain use information from hundreds of data sources; CPDat contains reported chemical substance data on thousands of consumer products (obtained from product Safety Data Sheets or ingredient lists), while CPCat contains general chemical substance use information for over 75,000 chemical substances from public manufacturer, government, or industry chemical substance lists. Two exposure sources, breast milk and residential dust, are characterized here via a recently published meta-analyses93,94.
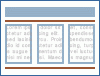
Table 5
Criteria used to evaluate the susceptible population exposure domain metric.
Once it is determined that a chemical substance has available exposure source information, exposure sources are evaluated for the chemical substance. If the exposure source is determined for a substance, the value assigned to the source (represented in Figure 8 to the right of each potential source) is added to the total for that chemical substance. The value associated with each exposure source represents the relative magnitude of potential exposure differential between children and adults. The total for each chemical substance can range from 1 – 18 and is used to assign the susceptible population domain metric based on the ranges specified in Table 5.
Limitations and Longer-Term Options
A limitation of the susceptible population exposure domain is that only children’s exposure was included. Future updates may expand this component to include other susceptible populations (e.g., workers, elderly). Further, the metric developed herein was designed to capture information about exposure sources relevant to children, using publicly available exposure-relevant data that has been compiled and curated by ORD. Collection and curation of product and monitoring data by ORD is ongoing, and new data or data streams can be incorporated into this workflow when they reach an acceptable level of quality review. It is likely that the available product and monitoring data used to develop the susceptible population metric may not be representative of the total chemical substance landscape to which children are exposed. For example, the children’s products for which data are available in CPDat may not be representative of all products used by children, and the chemical substances reported on safety data sheet for these products are not necessarily representative of all the chemical substances in those products. Therefore, the lack of a positive for a chemical substance data source does not necessarily imply a global negative (only a negative for these data sources). Additionally, this domain could be further expanded through incorporation of any future ExpoCast models as they are developed, including those that generated consensus exposure predictions for individual cohorts (e.g., children, the elderly).
Persistence and Bioaccumulation Domain
Persistence refers to the tendency of a chemical substance to remain in the environment in its original form, potentially resulting in exposures that last for a long period of time. Bioaccumulation refers to the tendency of a chemical substance to accumulate in biota. Although generally considered separately, these properties overlap to a substantial degree because molecular features that tend to increase chemical persistence, such halogenation and/or steric features that limit microbial degradation, often promote increased bioaccumulation. Chemical bioaccumulation may occur in both terrestrial and aquatic environments; however, bioaccumulation assessments are often focused on the potential for chemical accumulation in fish. This focus reflects the well-known tendency of hydrophobic substances to partition out of the water column and into aquatic biota. Fish may accumulate chemicals directly from water and by consuming contaminated food items.
Persistence Evaluation
The workflow for evaluating persistence is based on ultimate biodegradation, which is defined as complete mineralization resulting in the formation of carbon dioxide, water, mineral salts and biomass and is measured in weeks (Figure 9). If measured biodegradation half-lives are available, the persistence domain metric is derived based on this data. In the absence of measured data, calculated ratings for ultimate biodegradability are obtained from BIOWIN3 (Ultimate Survey Model) in EPI Suite95.

Figure 9
Tiered evaluation process associated with the persistence domain metric. Persistence evaluation is performed by comparing a half-life value for ultimate biodegradation. IG = information gathering.
Persistence evaluation is performed by comparing a half-life value from the workflow for overall persistence to the criteria recommended in the 2012 TSCA Work Plan Chemicals: Methods Document96 (Table 6).
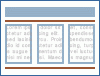
Table 6
Criteria used to calculate the persistence domain metric.
Bioaccumulation Evaluation
Bioaccumulation is typically evaluated using a steady-state fish bioaccumulation factor (BAF) or bioconcentration factor (BCF). The BAF (chemical substance concentration in fish/chemical substance concentration in water; L/kg) quantifies chemical substance accumulation occurring in fish by all possible routes of exposure and is generally determined in field-collected animals. The BCF (chemical substance concentration in fish/chemical substance concentration in water; L/kg) quantifies accumulation occurring in a water-only exposure and is usually measured in controlled laboratory studies. Because BCFs can be determined experimentally, they are often used as a surrogate measure of bioaccumulation potential when measured BAFs are unavailable. However, a measured BAF better represents the potential for chemical substance bioaccumulation in a real-world setting.
A workflow for evaluating chemical substance bioaccumulation potential in fish is shown in Figure 10. In most cases, this workflow leads to a BAF or BCF that can be evaluated against previously defined criteria from the 2012 TSCA Work Plan Chemical Methods Document97 (Table 7). Possible exceptions include chemical substances that are outside the applicability domain of predictive models (see below). Relative confidence in these BAF and BCF values is represented by flags indicating “high,” “intermediate,” or “low” confidence. The structure of the workflow represents several general considerations. First, preference is given to chemical substances for which measured BAFs are available. If a measured BAF is not available, chemical substances for which measured BCFs are available receive preference. A database of measured fish BAFs and BCFs was published by Arnot and Gobas98. The BCFs assembled by these authors were evaluated for data quality based on aspects of study design and the collection of supporting analytical information. This database did not provide criteria for evaluating fish BAFs; however, methods used to measure reported BAFs were compared to existing guidance for analysis of environmental samples. For the purposes of the bioaccumulation domain metric, a “high quality BCF” refers to a BCF that was scored 1 or 2 in the Arnot-Gobas database across the entire set of evaluation criteria (labeled “acceptable confidence” by the authors), while a “high quality BAF” refers to a BAF that was judged by the same authors to be of “acceptable quality.” Either of these bioaccumulation metrics can be used as the basis for evaluating bioaccumulation potential, and the resulting values are flagged as “high confidence.”
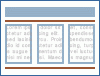
Table 7
Criteria for bioaccumulation domain metric.
If a measured BAF or BCF is not available, the chemical substance is passed on to predictive BCF modeling. The workflow assumes that all structures are neutral at environmental pH values. In most cases, this represents a conservative assumption; that is, BCFs predicted under this assumption are likely to be higher than the actual BCF values associated with these compounds. However, exceptions to this general rule are known to exist.
The two models used to predict bioconcentration of neutral chemical substances are the Arnot-Gobas QSAR model99 (henceforth “Arnot-Gobas model” without citation) included in the BCFBAF module of EPI Suite ver. 4.11, and the OPERA BCF QSAR model3 (henceforth “OPERA BCF model” without citation) developed by the EPA ORD. The Arnot-Gobas model is a one-compartment mass-balance description that predicts bioconcentration from competing rates of uptake and elimination, while the OPERA BCF model employs a k-nearest neighbor approach to calculate a BCF from measured values for chemical substances that exhibit molecular similarity to the chemical substance. Before using either model, the chemical substance is evaluated using the KOWWIN model in EPI Suite to determine whether it possesses a predicted log KOW value > 9. If the predicted log KOW is > 9, the chemical substance is flagged as “low confidence.” This designation reflects uncertainty in both the log KOW estimate and the modeled bioconcentration prediction.
The workflow is structured so that the Arnot-Gobas model is implemented first. This model provides several BCF metrics. For this workflow, we focused on BCF prediction for lower trophic level fish, assuming biotransformation. This focus reflects the fact that most standardized in vivo BCF tests are performed using small fish species or juveniles of larger species. If the Arnot-Gobas returns a value, the chemical substance is passed on for evaluation of bioaccumulation potential. If not, the chemical substance is passed to the OPERA BCF model.
If the OPERA model does not return a value, the process terminates with an IG flag, which indicates that there are no data (measured or predicted) available. This outcome is anticipated for some inorganic chemical substances, metals, organometallic chemical substances and mixtures. If OPERA returns a value, a determination is made whether the chemical substance falls within the model’s applicability domain (AD). A chemical substance that falls within the AD is passed on for evaluation. A chemical substance that falls outside the AD is flagged as “low confidence” and then passed on for evaluation. Any chemical substance for which the evaluation is based on a predicted BCF is flagged “medium confidence” unless it has been flagged “low confidence” at some earlier step (e.g., because it possesses a log KOW value > 9). This designation reflects the fact that BCF prediction models have been developed and calibrated using data for a relatively small number of industrial chemical substances (< 1000). In addition, predicted BCFs do not account for potential food web effects (i.e., biomagnification).
Limitations and Longer-Term Options
EPA is currently in the process of adopting a new approach for evaluating persistence based on the potential half-life in air, water, soil, and sediment. The approach factors the partitioning characteristics of the chemical substances and potential removal pathways based on standard physical-chemical substance properties and environmental fate parameters. Once adopted, this approach may be included in the persistence component of this workflow.
Measured BAFs for some poorly metabolized compounds may exceed measured BCFs by an order of magnitude or more due to biomagnification of chemical residues (i.e., higher concentrations at successively higher trophic levels)100. These differences are also apparent in modeled BAF and BCF values. Given this fact, as well as the preference of measured BAFs over measured BCFs noted above, it is reasonable to ask whether preference should be given to predicted BAFs over predicted BCFs. Because predicted BAFs may exceed modeled BCFs, particularly for chemicals of special concern from a bioaccumulation perspective, they represent a more conservative metric of bioaccumulation. Predicted BAFs are well suited, therefore, to performing risk assessments for individual chemicals. The current focus on predicted BCFs was motivated by the fact that the number of published BCFs for fish greatly exceeds the number of published BAFs. The use of a BCF prediction model therefore results in comparable modeled and measured values, which is important if both data sources are used to perform a relative evaluation of bioaccumulation potential for many chemicals. To evaluate this question further, Costanza et al.101 predicted BCFs and BAFs for 6,034 chemicals using the Arnot-Gobas model, and then compared these values to criteria used by EPA to screen chemicals for bioaccumulation potential (“not bioaccumulative” = BCF or BAF < 1000; “bioaccumulative” = 1000 < log BCF or log BAF < 5000; “highly bioaccumulative” = BCF or BAF > 5000). The results showed that for 86% of chemicals there was no change in bioaccumulation rating when using the BAF rather than the BCF. This finding suggests that for screening-level assessments, modeled BCFs and BAFs generally lead to the same conclusion.
The inability of current modeling approaches to adequately predict bioaccumulation of ionizable compounds represents a well-recognized research need. Process-based models that describe uptake and accumulation of ionizable compounds in fish have been described by several authors102,103. Additional models have been developed specifically for per- and polyfluorinated alkylated substances (PFAS), many of which are > 99% ionized at environmental pH values104. Ionizable chemicals represent a particularly difficult challenge in the field of predictive bioaccumulation modeling. Guidance provided in EPI Suite (BCFBAF Help menu, 7.1 Bioconcentration Factor (BCF), 7.1.1 Estimation Methodology) indicates that the Arnot-Gobas model is not intended for use with ionizable compounds. Instead, the guidance recommends using an alternative model which bins chemicals based on their estimated log KOW values (neutral form). BCFs predicted by this model for such compounds tend to be relatively low (maximum log BCF of 1.75). Additional guidance indicates, however, that this model should not be used for compounds possessing specific molecular features. These features include the presence of an aromatic azo group (a structural component of many pigments and dyes), a charged metal species (esp., mercury or tin), or a long chain alkyl group (e.g., many cationic and anionic surfactants). Not given on this list of molecular features is the presence of a fluorine group. Nevertheless, this model appears to be poorly suited for ionizable PFAS compounds, several of which have been shown to accumulate in fish (log BCFs > 3)105.
The BCF dataset used to train the OPERA BCF model contains a small (< 70) number of ionizable compounds. In principal, these measured BCFs reflect the net result of all processes responsible for bioconcentration including chemical speciation in the water and fish, and uptake and accumulation of all relevant chemical species. Given the small number of chemicals in the training set, however, there is a relatively high likelihood that a given ionizable chemical will fall outside the applicability domain of the QSAR. Moreover, as indicated by the exclusionary criteria given in EPI Suite, some ionizable compounds may require special attention.
Anticipating future acceptance of a process-based model for ionizable compounds, we may consider possible modifications to the decision tree used to evaluate chemical bioaccumulation potential. The first step in a revised tree would be to determine whether a chemical is substantially ionized at environmental (5 − 9) pH values. In a recent review, it was suggested that pH effects on uptake and accumulation of ionizable chemicals by fish are likely to be minor unless the extent of ionization in bulk water exceeds 90%106. Of special concern are weak acids and bases for which an accurate estimate of pKa would be required. Ideally, this estimate would be obtained using an open-source software tool that has been evaluated against measured data as well as existing proprietary software. An OPERA pKa model may provide such a tool107. If exclusionary criteria are still required, they would be applied at an early stage in the decision process. For some chemical classes (e.g., PFAS), the decision tree may direct the user to employ a model specifically designed for such compounds. BCFs predicted by a general model for ionizable compounds could be handled in a manner analogous to that for BCFs presently generated by the Arnot-Gobas model. Assuming further that the OPERA BCF model will be updated and trained using newly available data for ionized chemicals, we may anticipate a situation wherein BCFs can be predicted by a process-based model and by the OPERA BCF model. This would require some type of process for averaging these predictions or choosing one in preference to the other. Presently, due to a lack of data for model calibration and evaluation, these models cannot be applied with confidence to the broad range of ionizable structures contained on the TSCA inventory108. It is anticipated that these or similar models can be incorporated into future bioaccumulation evaluation efforts.
Combined Persistence and Bioaccumulation Domain Metric
The combined persistence and bioaccumulation domain metric (Table 8) is obtained by adding the separate metrics from the persistence and bioaccumulation workflows (Figures 9 and 10) and described in Tables 6 and 7. This process is consistent with the method recommended in the 2012 TSCA Work Plan Chemical Methods document109.
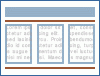
Table 8
Combined persistence/bioaccumulation domain metric.
Skin Sensitization and Skin/Eye Irritation Domain
Skin sensitization and skin/eye irritation are important potential hazards of chemical substances that are of concern for human health. These are addressed here in a separate domain due to different routes of exposure and different data sources for these endpoints. Ocular and dermal exposures can occur through a variety of sources, particularly occupational exposures as well as consumer exposures
Local effects are changes at the site of contact (skin, eye, mucous membrane/gastrointestinal tract, or mucous membrane/respiratory tract) as a result of exposure to a chemical substance. Such changes after a single exposure may be categorized as irritant or corrosive, depending on the severity and reversibility of the outcomes. Corrosive substances are those which may destroy living tissues with which they come into contact. Irritant substances are non-corrosive substances which, through immediate contact with the tissue may cause inflammation.
Skin sensitization denotes the immune-mediated hazards associated with human allergic contact dermatitis and/or rodent contact hypersensitivity. Allergic contact dermatitis is the clinical term that indicates the presence of skin erythema and edema that result from delayed type IV cell-mediated skin hypersensitivity.
Skin Sensitization and Skin/Eye Irritation Evaluation
The proposed skin sensitization and skin/eye irritation domain metric incorporates GHS hazard codes or hazard categories from the ECHA Classification, Labelling and Packaging (CLP) Regulation and agencies of several countries (e.g., Canada, Japan, Denmark), as well as study-level REACH registration data from ECHA via OECD’s eChemPortal. GHS classification and labeling information was extracted from websites of the environmental or occupational health agencies of individual countries. The study-level data in eChemPortal were obtained by searching for the endpoints of eye irritation (in vivo and in vitro), skin corrosion (in vitro), skin irritation (in vivo), and skin sensitization (in vivo local lymph node assay (LLNA), in vivo non-LLNA and in vitro).
The GHS classifications were converted to a 4-level ranking of Low (L), Moderate (M), High (H), and Very High (VH), which was then converted to a numerical scale of 1–4 (L=1, VH=4). The ECHA experimental data were converted to the same scale using a mapping from the result summary sentences provided by each study. An expert-derived dictionary was created that mapped each unique result sentence to a scoring level. The criteria for determining metrics are shown in Table 9 below. These criteria were based on the EPA’s Design for the Environment Program (DfE) Alternatives Assessment Criteria for Hazard Evaluation110,111.
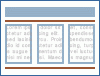
Table 9a
Criteria for Skin Sensitization Sub-Domain Metric.
For each associated sub-domain (skin sensitization, skin irritation, eye irritation) a chemical substance could have one or more hazard determinations from different sources. The evaluation method used for combining these values into an overall metric for each sub-domain is shown in Figure 11. Briefly, the evaluation was based on the source of the information. Similar to the approach used by the Clean Production Action’s GreenScreen List Translator112, this evaluation method selects the highest value (highest hazard level) from the most authoritative source as the final output. In the method that was presently implemented (Figure 11), authoritative sources take precedence over screening sources, which take precedence over QSAR models. Within each of those three levels, the source that produces the highest value takes precedence. Using this method, an overall value across all sources was determined for each sub-domain. Then, the most conservative of the three sub-domain values was used as the final value for the skin/eye domain.

Figure 11
Tiered evaluation process associated with the skin sensitization, skin/eye irritation domain metric. IG = information gathering flag; L = low; M = medium; H = high; VH = very high.
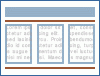
Table 9b
Criteria for Skin Irritation Sub-Domain Metric.
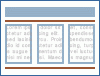
Table 9c
Criteria for Eye Irritation Sub-Domain Metric.
Limitations and Longer-term Options
A limitation of the skin sensitization and skin/eye irritation evaluation was the availability of data sources, particularly a lack of large databases of validated non-animal data. Thus, the data sources that were used have some limitations. While there are non-animal test methods to assess both skin sensitization and skin/eye irritation and corrosion, the data for large numbers of chemical substances remains limited. Moreover, the ability of alternative eye irritation assessment methods to discriminate between GHS categories remains a limitation. The source of the ECHA data in eChemPortal is industry-submitted REACH registration dossiers. REACH requires that at least 5% of the registration dossiers of each tonnage band of chemical substances must be checked for compliance with legal requirements for chemical substance identity descriptions and safety information113. Because up to 95% of REACH registration dossiers may not be checked for compliance, the quality assurance of the data from these dossiers is limited. The majority of chemical substances (60–80%) had information from only the ECHA REACH dossiers or a GHS source but not both. For chemical substances that had data available from the REACH dossiers and another source, the results from the REACH dossiers often were consistent with the results from the other source. However, there were instances in which a chemical substance had a value of VH (4) based on the GHS classification data, but the ECHA data indicated a value of L (1). The GHS classifications were generally more severe on average than those from ECHA, which might be partially attributable to GHS classification being more likely to be conducted when there is prior cause for concern. The GHS categorization of “Not Classified”, which indicates that a chemical substance does not meet the requirements to be classified as hazardous under the GHS, was reported by Japan’s National Institute of Technology and Evaluation (NITE) but was not reported by the other sources of GHS data. An additional limitation relates to the lack of reporting of GHS classifications for chemicals which do not meet the classification criteria. For this reason, lack of a classification is ambiguous, meaning either no data or not meeting criteria. For the purpose of the PICS approach, "not classifiable" or "classification not possible" were interpreted as no data (or insufficient data or ambiguous). In contrast, we interpreted "not classified" as not meeting GHS criteria for being classified as hazardous. Further in-depth review would be considered part of the expert review for compounds of interest that may follow the application of the PICS approach, which would involve evaluating the mapping of summary sentences from REACH dossiers. The method of determining an overall value for each endpoint by selecting the most hazardous value from the most authoritative source reduces the influence of differences in data quality between different data sources, but the inability to check all primary sources for data quality remains a limitation. Potential longer-term improvements include additional quality assurance checks as well as the inclusion of additional data sources if such sources become available. Furthermore, new QSAR models are being developed to fill in missing data for these endpoints.
5.3. Scientific Domain Metric Calculation
The overall scientific domain output is calculated by summing the metrics from each of the 7 domains. Any individual domain metric of zero is given a value of 1, which helps to normalize the values across chemical substances and domains. The lack of data is captured in the IAM and is visualized in Figure 14 based on the size of the point representing the chemical. The maximum possible output is 27 and the minimum output is 7. The summed results are scaled to values from 0 to 100 to match the IAM. Therefore, the minimum value of 7 is converted to zero, with the maximum value of 27 equal to 100.
5.4. Information Availability Metric
The second dimension of the PICS approach is a metric that represents the information available for use in any future chemical substance risk evaluation. Under TSCA, there is no minimum data requirement necessary to perform a chemical substance risk evaluation, as decisions about what would be considered a sufficient amount of hazard or exposure data are typically context specific and would require expert judgment to determine. While this would be possible during the formal prioritization and risk evaluation processes, expert judgment is not part of the automated approach described here. The IAM is designed to automatically evaluate chemical substances based on the number and type of studies available to inform this analysis. To partially address the context-specific aspect of the data, this metric includes a relatively simple set of four modifying criteria of potentially relevant exposure, human health and ecological toxicity information. The criteria include a combination of primary use as a chemical substance intermediate, environmental half-life, water solubility, molecular weight, whether the chemical substance is an exempt polymer and whether the chemical substance has been assessed for human risk by an authoritative source (Figure 12). Following application of the criteria, the IAM is calculated as a function of information in the associated lists. Missing information is flagged for potential future information gathering, but these IG flags do not directly impact the IAM and only identify specific information gaps. The IAM is calculated by giving a chemical substance one point for having experimental data in each of the domains corresponding to the appropriate box in Figure 12. This metric does not take into account the quality or quantity of studies for each chemical. Predicted data is also not incorporated into this metric with the exception of the SEEM3 exposure model.
If the chemical substance has an authoritative human risk assessment from one of these specific sources (IRIS, EFSA, ATSDR, SIDs, OPPT, OPP), it is given a point for each of the 8 human information availability study types (mammalian values plus carcinogenicity, genotoxicity and skin and eye). The output is then calculated by dividing by the total number of domains for the appropriate box and multiplying by 100. A detailed scheme for the calculation is given in Appendix G, Figure G-1.
5.5. Results of the Proof-of-Concept Analysis
Overall Evaluation
The number of chemical substances in the current non-confidential TSCA active inventory is 33,364; however, only 14,017 of these are unique organic chemical substances with defined structures (Table 10). The majority of chemical substances in the inventory are mixtures of varying complexity. Chemical substances that have some in vivo mammalian and ecotoxicological data constitute 11–13% of the overall inventory and 3% have experimental cancer data. The data included in the PICS approach is public and excludes industry submitted CBI studies. The PICS approach also does not include data extracted from the literature beyond what is included in the Type 1 data sources currently being utilized.
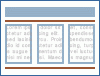
Table 10
Number of chemical substances in the non-confidential active TSCA inventory with specific types of experimental data.
As noted earlier, the POC238 was also selected to test the PICS approach using a subset of the TSCA active inventory and spanned a range of potential concern and information availability (Fig. 13).

Figure 13
Distributions of the scaled SDM and IAM for the POC238 subset. The x-axis displays the scaled value and the y-axis shows the frequency of that value in the subset. Similar histograms for the TSCA active inventory can be found in Appendix F.
The two-dimensional representation of the SDM and IAMs can be summarized for the POC238 (Figure 14; results used to inform this figure can be found in Appendix E). There is an association between IAM and SDM (i.e., more information tends to produce a higher value). This may be a result of potential testing or publication bias. Chemical substances that are expected to show or have previously shown indications of potential hazard will lead to more data being generated, while those that are not expected to show high hazard are less likely to be tested. Additionally, there is a publication bias towards positive results as most peer-reviewed publications do not describe negative results. Further, a lack of available data does not indicate a lack of toxicity.
Using the recently released TSCA high and low priority chemical substance candidates selected by EPA114, the high priority candidates generally having higher metrics than the low priority candidates when analyzed by the PICS approach (Fig 14). In part, these candidates were selected by expert reviewers examining both publicly available and CBI data for each chemical substance using a systematic review process115, which takes into consideration study quality and consistency in the database. Further, this review would take into consideration various policy aspects and scientific judgment that are not part of the automated PICS approach. Discrepancies between the conclusions of expert reviewers and the results of the PICS approach may be related to the different data sources used, but also may be related to the more in-depth review of the studies used as a basis for the candidate selection. For example, in some cases, the conservative decisions used in the genotoxicity domain of the PICS approach (i.e., assigning a positive genotoxicity score in the presence of one positive study regardless of the results of other studies) may give a chemical substance a higher domain metric than a weight of evidence analysis as the latter would take into account the full dataset.
In addition to the TSCA high and low priority candidates, the POC238 also includes a selection of chemical substances from other lists that had a prior expectation of higher or lower potential concern. For instance, the chemical substances from the 2014 TSCA Work Plan116 are generally expected to be of higher than average concern with an existing authoritative hazard assessment. The chemical substances on the SCIL list or chemical substances that are intentional food ingredients are expected to be of lower than average concern. Figure 16 summarizes the metric distributions for selected chemical substance lists from across the full TSCA active inventory. From this plot we can see that the SDM values are largely consistent with expectations. The TSCA high priority candidates and the 2014 TSCA Work Plan chemical substances are relatively high while the TSCA low priority candidates, SCIL, and food ingredients are relatively low. The median scientific domain metr6ic for the full TSCA active inventory is very low, but this generally reflects the overall low information availability. Comparatively low information availability is also seen in the SCIL and food ingredient lists. The POC238 list is enriched in the high priority regulatory chemical substances, and the remaining chemical substances were largely selected because of knowledge of some toxicological concern. As a result, the POC238 has a distribution similar to the high concern lists and is not reflective of the overall TSCA active inventory.
To illustrate how the process works for individual chemical substances, we show information for two chemical substances, one with a relatively high value for the SDM (benzene, 68) and one with a relatively low value (3-methoxybutyl acetate, 14.7). Both chemical substances have relatively high values for the IAM (benzene = 93% and 3-methoxybutyl acetate = 67%). Table 11 shows values for individual domains. Following the overall metric scores are the information gathering flags that indicate the types of in vivo data that are lacking and information about the BAF values used. There were no IG flags for the other domains. Next are the seven individual domain metrics, whose sum could range from 7 to 27. As described above, the overall score is equal to 100 x (sum-7)/(27–7). Benzene has a 2.5 (out of 4) for the human hazard-to-exposure ratio metric, based on the HER value. 3-Methoxybutyl acetate has a 2.3 of out 4 for the human hazard-to-exposure ratio metric.
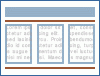
Table 11
Results for benzene and 3-methoxybutyl acetate.
The human health repeat dose POD for benzene is 0.015 mg/kg-bw/day, which is the chronic NOAEL from an authoritative human hazard assessment (ATSDR / CDC). The corresponding value for 3-methoxybutyl acetate is 100 mg/kg-bw/day, which is a NOAEL from an ECHA repeat dose study in guinea pigs. For the ecological hazard metric, the minimum PODs for the two chemical substances are very similar (0.71 and 0.49 mg/L), which leads to the same ecological hazard metric value. The minimum ecological POD for benzene is 0.49 mg/L, derived from an acute study (96 hours) with POD of a 4.9 mg/L (divided by 10 in our process) in sockeye salmon [ECHA / eChemPortal117. For 3-methoxybutyl acetate, the minimum ecological POD is 7.1 mg/L in an acute zebrafish study from ECHA / eChemPortal. This POD was then divided by 10 using the acute-to-repeat dose factor. In the OCSPP evaluation of this chemical, the zebrafish study was disregarded because it did not meet the minimum quality criteria. OCSPP identified a repeat-dose study with a NOAEL of 74 mg/L from a source not included in the current Type 1 data sources.
Benzene has a maximum value for the cancer metric because it is classified as a Group 1 human carcinogen by IARC, while 3-methoxybutyl acetate had no cancer-related information. For genotoxicity, benzene is classified as genotoxic and 3-methoxybutyl acetate is classified as non-genotoxic, based on a single negative Ames assay. Benzene has 27 total assays in the genotoxicity database, including 2 positive Ames tests, leading to the positive overall classification. However, there are also 3 negative and 2 ambiguous Ames results. Both chemical substances had elevated values for the susceptible population metric (4 for benzene, 3 for 3-methoxybutyl acetate), indicating that there is a high probability that children may be exposed to these chemical substances. Benzene has a moderate metric for persistence / bioaccumulation based on a persistence score from EpiSuite of 2.4, while 3-methoxylbutyl acetate has a low metric for persistence/bioaccumulation based on low persistence and bioaccumulation values. Benzene has a sensitization / irritation score of 3 based on High scores for both skin and eye irritation. These are based on GHS classifications, which were consistent between ECHA, New Zealand, Canada, Malaysia and Japan. 3-Methoxybutyl acetate has low scores for skin and eye irritation.
Each of the workflows also includes the use of IG flags to identify missing experimental information, even in the case where predicted values could be used (Figure 15). For example, there are a large number of study types that may be used in calculating the human hazard-to-exposure domain metric. However, even if one or more such values are available, the schema depicted in Figure 12 includes flags for study types that are missing. For instance, Figure 15 shows that a large fraction (0.85) of POC238 chemical substances are missing neurotoxicity data, although most had at least one other acceptable mammalian study to be used in calculating an HER value. Similarly, for the ecological hazard domain, this figure shows that that most of the POC were lacking an acute plant study, although in most cases there was still at least one in vivo study that could be used to provide an ecotoxicological POD.

Figure 15
Plot of the frequency distribution of 1G flags for each SDMs for the POC238 set of chemical substances. IG flags are designed to highlight data types used in specific SDMs as well as possible data gaps. IG = information gathering.
5.6. Overall Limitations and Long-term Options
The PICS approach described here was designed under consideration for use in support of TSCA. However, this approach was designed to be adaptable to other decision contexts. The main limitations in adapting the PICS approach is the availability of state of the science methods and access to curated datasets. Addressing these areas would allow for the incorporation of other specific endpoints (e.g., reproductive or developmental toxicity), pathways (e.g., estrogenic), and additional data sources. As the science progresses, changes to this approach could also address applicability to data poor compounds by increasing focus on the use of NAMs to help fill specific data gaps. Future advances across the scientific domains, including the development and incorporation of additional NAMs (e.g., in chemico and alternative species models) which could also aid in incorporating specific chemical classes that are not easily addressed with the methods in the current PICS approach (e.g., volatile chemicals). The adaptability of the approach also applies to how the impact of specific domains may be adjusted. Depending on the decision context, the user may want to weight some scientific domains or data sources differently than we have done in this proof-of-concept case study in order to focus on scientific endpoints of specific concern for that decision context. Alternatively, the decisionmaker may want to incorporate additional IG flags to highlight aspects important to that decision context (e.g., IG flag for GHS classification for carcinogenicity). Longer term efforts could also help to address how this work could be applied to mixtures, although more research is needed to determine how best to address this issue. As noted earlier in the document, a limitation for this case study is the focus of the susceptible exposure domain only on children’s exposure. However, if data sources are available, they could be incorporated to include additional populations (e.g., workers, elderly) as appropriate for future applications. As with the hazard domains, as the research and data evolve, additional populations can be incorporated as appropriate for the decision context.
Footnotes
- 26
This list can be found at https://comptox
.epa.gov /dashboard/chemical_lists/TSCAHIGHPRI - 27
This list can be found at https://comptox
.epa.gov /dashboard/chemical_lists/TSCALOWPRI - 28
Type 1 sources were defined as data sources storing reasonably available and relevant information that could be readily queried and extracted in a structured manner. This includes existing databases (and dashboards) that allow the user to sift through information using a graphical user-interface, a direct query such as Structured Query Language (SQL), or webservice Application Programming Interfaces (APIs). (https://www
.epa.gov/sites /production/files /2018-09/documents /preprioritization_white_paper_9272018 .pdf) - 29
- 30
Grulke CM, Williams AJ, Thillanadarajah I, Richard AM. (2019). EPA’s DSSTox database: History of development of a curated chemistry resource supporting computational toxicology research. Computational Toxicology 12:100096.
- 31
Richard, AM (2004). DSSTOX website launch: Improving public access to databases for building structure-toxicity prediction models. Preclinica 2(2):103–108.
- 32
Williams AJ, Grulke CM, Edwards J, McEachran AD, Mansouri K, Baker NC, Patlewicz G, Shah I, Wambaugh JF, Judson RS, et al. (2017). The CompTox Chemistry Dashboard: a community data resource for environmental chemistry. Journal of Cheminformatics 9(1):61.
- 33
Curated list of non-confidential substances on the active TSCA inventory (https://comptox
.epa.gov /dashboard/chemical_lists /TSCA_ACTIVE_NCTI_0320). The list contains 33,364 chemical substances as of March 2020. - 34
TSCA10 represents the first ten TSCA Work Plan chemical substances selected for risk evaluation in 2016.
- 35
TSCA90 represents the TSCA Work Plan chemical substances from the 2014 update.
- 36
The scientific domains include human hazard relative to exposure, ecological hazard, carcinogenicity, genotoxicity, exposure to susceptible populations, persistence and bioaccumulation, skin sensitization and skin/eye irritation
- 37
TSCA Work Plan Methods Document 2012 (https://www
.epa.gov/sites /production/files /2014-03/documents /work_plan_methods_document_web_final .pdf). - 38
- 39
TSCA Work Plan Methods Document 2012 (https://www
.epa.gov/sites /production/files /2014-03/documents /work_plan_methods_document_web_final .pdf). - 40
- 41
Ring CL, Arnot JA, Bennett DH, Egeghy PP, Fantke P, Huang L, Isaacs KK, Jolliet O, Phillips KA, Price PS, Shin HM. (2018). Consensus modeling of median chemical intake for the US population based on predictions of exposure pathways. Environmental Science & Technology 53(2):719–32.
- 42
Near-field represents exposures occurring proximal to use-field (e.g., sources inside the home, for example from consumer products).
- 43
Far-field represents exposures occurring far from use or as a result of environmental emission (e.g., ambient sources outside the home, for example from industrial releases).
- 44
Centers for Disease Control and Prevention. "Fourth report on human exposure to environmental chemical substances, updated tables." US Department of Health and Human Services, Centers for Disease Control and Prevention (2017).
- 45
Ring CL, Arnot J, Bennett DH, Egeghy P, Fantke P, Huang L, Isaacs KK, Jolliet O, Phillips K, Price PS, Shin HM, Westgate JN, Setzer RW, Wambaugh JF. (2019). Consensus Modeling of Median Chemical Intake for the U.S. Population Based on Predictions of Exposure Pathways. Environmental Science and Technology 53(2):719–732.
- 46
Ring CL, Pearce RG, Setzer RW, Wetmore BA, Wambaugh JF. (2017). Identifying populations sensitive to environmental chemical substances by simulating toxicokinetic variability. Environment International 106:105–118.
- 47
Pearce RG, Setzer RW, Davis JL, Wambaugh JF. (2017). Evaluation and calibration of high-throughput predictions of chemical distribution to tissues. Journal of Pharmacokinetics and Pharmacodynamics 44(6):549–565.
- 48
Pearce RG, Setzer RW, Strope CL, Wambaugh JF, Sipes NS. (2017). httk: R package for high-throughput toxicokinetics. Journal of Statistical Software 79(4):1.
- 49
Paul-Friedman K, Gagne M, Loo LH, Karamertzanis P, Netzeva T, Sobanski T, Franzosa JA, Richard AM, Lougee RR, Gissi A, Lee JJ, Angrish M, Dorne JL, Foster S, Raffaele K, Bahadori T, Gwinn MR, Lambert J, Whelan M, Rasenberg M, Barton-Maclaren T, Thomas RS. (2020). Utility of In Vitro Bioactivity as a Lower Bound Estimate of In Vivo Adverse Effect Levels and in Risk-Based Prioritization. Toxicological Sciences 173(1):202–225.
- 50
Wetmore BA, Wambaugh JF, Allen B, Ferguson SS, Sochaski MA, Setzer RW, Houck KA, Strope CL, Cantwell K, Judson RS, LeCluyse E. (2015). Incorporating high-throughput exposure predictions with dosimetry-adjusted in vitro bioactivity to inform chemical toxicity testing. Toxicological Sciences 148(1):121–36.
- 51
Patlewicz G, Wambaugh JF, Felter SP, Simon TW, Becker RA. (2018). Utilizing Threshold of Toxicological Concern (TTC) with high throughput exposure predictions (HTE) as a risk-based prioritization approach for thousands of chemicals. Computational Toxicology 7:58–79.
- 52
Patlewicz G, Jeliazkova N, Safford RJ, Worth AP, Aleksiev B. (2008). An evaluation of the implementation of the Cramer classification scheme in the Toxtree software. SAR and QSAR in Environmental Research 19(5–6):495–524.
- 53
TSCA Work Plan Chemicals: Methods Document, https://www
.epa.gov/sites /production/files /2014-03/documents /work_plan_methods_document_web_final .pdf - 54
- 55
Paul Friedman K, Gagne M, Loo LH, Karamertzanis P, Netzeva T, Sobanski T, Franzosa JA, Richard AM, Lougee RR, Gissi A, Lee JJ, Angrish M, Dorne JL, Foster S, Raffaele K, Bahadori T, Gwinn MR, Lambert J, Whelan M, Rasenberg M, Barton-Maclaren T, Thomas RS. (2020). Utility of In Vitro Bioactivity as a Lower Bound Estimate of In Vivo Adverse Effect Levels and in Risk-Based Prioritization. Toxicological Sciences 173(1):202–225.
- 56
Nelms MD, Patlewicz G. (2020) Derivation of new Thresholds of Toxicological Concern values for exposure via inhalation for environmentally-relevant chemicals. Frontiers in Toxicology 2:5.
- 57
- 58
- 59
NTP Toxicology/Carcinogenicity Study Overview. https://ntp
.niehs.nih .gov/testing/types/cartox/index.html - 60
OECD Draft guidance http://www
.oecd.org/chemicalsafety /testing/44960015.pdf - 61
- 62
Eastmond DA, Hartwig A, Anderson D, Anwar WA, Cimino MC, Dobrev I, Douglas GR, Nohmi T, Phillips DH, Vickers C. (2009). Mutagenicity testing for chemical risk assessment: update of the WHO/IPCS Harmonized Scheme. Mutagenesis 24:341–349.
- 63
Guidance Document on Revisions to OECD Genetic Toxicology Test Guidelines (2015) http://www
.oecd.org/chemicalsafety /testing /Genetic%20Toxicology %20Guidance%20Document %20Aug%2031%202015.pdf - 64
International Conference on Harmonisation. (2012). Genotoxicity Testing and Data Interpretation for Pharmaceuticals Intended for Human Use. S2(R1). http://www
.ich.org/fileadmin /Public_Web_Site /ICH_Products/Guidelines /Safety/S2_R1/Step4/S2R1_Step4 .pdf - 65
NTP Genetic Toxicology. https://ntp
.niehs.nih .gov/testing/types/genetic/index.html - 66
Thomas RS, Philbert MA, Auerbach SS, Wetmore BA, Devito MJ, Cote I, Rowlands JC, Whelan MP, Hays SM, Andersen ME, Meek ME, Reiter LW, Lambert JC, Clewell HJ 3rd, Stephens ML, Zhao QJ, Wesselkamper SC, Flowers L, Carney EW, Pastoor TP, Petersen DD, Yauk CL, Nong A. (2013). Incorporating new technologies into toxicity testing and risk assessment: Moving from 21st century vision to a data-driven framework. Toxicological Sciences. 136:4–18.
- 67
Dearfield KL, Gollapudi BB, Bemis JC, Benz RD, Douglas GR, Elespuru RK, Johnson GE, Kirkland DJ, LeBaron MJ, Li AP, Marchetti F, Pottenger LH, Rorije E, Tanir JY, Thybaud V, van Benthem J, Yauk CL, Zeiger E, Luijten M. (2017). Next generation testing strategy for assessment of genomic damage: A conceptual framework and considerations. Environmental and Molecular Mutagenesis 58:264–283.
- 68
Williams RV, DM DeMarini, LF Stankowski Jr, PA Escobar, E Zeiger, J Howe, R Elespuru, KP Cross. (2019). Are all bacterial strains required by OECD mutagenicity test guideline TG471 needed? Mutation Research 848:503081.
- 69
Hansen K, Mika S, Schroeter T, Sutter A, ter Laak A, Steger-Hartmann T, Heinrich N, Müller KR. (2009). Benchmark data set for in silico prediction of Ames mutagenicity. Journal of Chemical Information and Modelling 49(9):2077–81.
- 70
More details can be found at https://www
.epa.gov/chemical-research /toxicity-estimation-software-tool-test - 71
Ashby J, Tennant RW. (1991). Definitive relationships among chemical structure, carcinogenicity and mutagenicity for 301 chemicals tested by the U.S. NTP. Mutation Research 257(3):229–306.
- 72
Carcinogens database developed by ISS. http://old
.iss.it/publ /anna/2008/1/44148.pdf - 73
Hasselgren C, Ahlberg E, et al. (2019). Genetic toxicology in silico protocol. Regulatory Toxicology and Pharmacology 107:104403.
- 74
Pradeep P, Judson R, DeMarini, DM, Keshava N, Martin TM, Dean J, Gibbons CF, Sima A, Warren SH, Gwinn MR, Patlewicz, G. (2021). Evaluation of existing QSAR models and structural alerts and development of new ensemble models for genotoxicity using a newly compiled experimental dataset. Computational Toxicology 18:100167.
- 75
United Nations. (2017). Globally harmonized system of classification and labeling of chemical substances. Seventh revised edition. United Nations, New York, NY, USA. ST/SG/AC.10/30/Rev.7
- 76
United Nations. (2017). Globally harmonized system of classification and labeling of chemical substances. Seventh revised edition. United Nations, New York, NY, USA. ST/SG/AC.10/30/Rev.7
- 77
- 78
- 79
- 80
- 81
- 82
United Nations. (2017). Globally harmonized system of classification and labeling of chemical substances. Seventh revised edition. United Nations, New York, NY, USA. ST/SG/AC.10/30/Rev.7
- 83
United Nations. (2017). Globally harmonized system of classification and labeling of chemical substances. Seventh revised edition. United Nations, New York, NY, USA. ST/SG/AC.10/30/Rev.7
- 84
Water solubility is predicted by OPERA (Mansouri et al., 2018; https://www
.ncbi.nlm .nih.gov/pmc/articles/PMC5843579/) - 85
United Nations. (2017). Globally harmonized system of classification and labeling of chemical substances. Seventh revised edition. United Nations, New York, NY, USA. ST/SG/AC.10/30/Rev.7
- 86
- 87
United Nations. (2017). Globally harmonized system of classification and labeling of chemical substances. Seventh revised edition. United Nations, New York, NY, USA. ST/SG/AC.10/30/Rev.7
- 88
Environmental Protection Agency (2006). A Framework for Assessing Health Risks of Environmental Exposures to Children, EPA/600/R-05/093F.
- 89
Environmental Protection Agency (2002). Child Specific Exposure Factors Handbook, EPA-600-P-00–002B.
- 90
Ring CL, Arnot JA, Bennett DH, Egeghy PP, Fantke P, Huang L, Isaacs KK, Jolliet O, Phillips KA, Price PS, Shin HM, Westgate JN, Setzer RW, Wambaugh JF. (2019). Consensus modeling of median chemical intake for the U.S. population based on predictions of exposure pathways. Environmental Science and Technology 53(2):719–732.
- 91
Dionisio KL, Phillips K, Price PS, Grulke CM, Williams A, Biryol D, Hong T, Isaacs KK. (2018). The Chemical and Products Database, a resource for exposure-relevant data on chemicals in consumer products, Scientific Data, 5: 1–9.
- 92
Dionisio KL, Frame AM, Goldsmith MR, Wambaugh JF, Liddell A, Cathey T, Smith D, Vail J, Ernstoff AS, Fantke P, Jolliet O, Judson RS. (2015). Exploring consumer exposure pathways and patterns of use for chemicals in the environment. Toxicology Reports 2:228–37.
- 93
Lehmann GM, LaKind JS, Davis MH, Hines EP, Marchitti SA, Alcala C, Lorber C. (2018). Environmental chemicals in breast milk and formula: Exposure and risk assessment implications. Environmental Health Perspectives 126:96001.
- 94
Mitro SD, Dodson RE, Singla V, Adamkiewicz G, Elmi AF, Tilly MK, Zota AR. (2016). Consumer product chemicals in indoor dust: A quantitative meta-analysis of U.S. studies. Environmental Science and Technology 50: 13611–11.
- 95
US EPA. (2019). Estimation Programs Interface Suite™ for Microsoft® Windows, v 4.11. United States Environmental Protection Agency, Washington, DC, USA.
- 96
U.S EPA. (2012). TSCA Work Plan Chemicals: Methods Document. U.S. Environmental Protection Agency, Office of Pollution Prevention and Toxics. https://www
.epa.gov/sites /production/files /2014-03/documents /work_plan_methods_document_web_final .pdf - 97
U.S EPA. (2012). TSCA Work Plan Chemical Methods Document. U.S. Environmental Protection Agency, Office of Pollution Prevention and Toxics. https://www
.epa.gov/sites /production/files /2014-03/documents /work_plan_methods_document_web_final .pdf - 98
Arnot JA, Gobas, FA. (2006). A review of bioconcentration factor (BCF) and bioaccumulation factor (BAF) assessments for organic chemicals in aquatic organisms. Environmental Reviews 14:257–297.
- 99
Arnot JA, Gobas F. (2003). A generic QSAR for assessing the bioaccumulation potential of organic chemical substances in aquatic food webs. QSAR and Combinatorial Science. 22:337–345.
- 100
Arnot JA, Gobas, FA. (2006). A review of bioconcentration factor (BCF) and bioaccumulation factor (BAF) assessments for organic chemicals in aquatic organisms. Environmental Reviews 14:257–297.
- 101
Costanza J, Lynch DG, Boethling RS, Arnot JA. (2012). Use of the bioaccumulation factor to screen chemicals for bioaccumulation potential. Environmental Toxicology and Chemistry 31:2261–2268.
- 102
Erickson RJ, McKim JM, Lien GJ, Hoffman AD, Batterman, SL. (2006) Uptake and elimination of ionizable organic chemicals at fish gills. II. Observed and predicted effects of pH, alkalinity, and chemical properties. Environmental Toxicology and Chemistry 25:1522–1532.
- 103
Armitage JM, Arnot JA, Wania F, Mackay D. (2013). Development and evaluation of a mechanistic bioconcentration model for ionogenic organic chemicals in fish. Environmental Toxicology and Chemistry 32:115–128.
- 104
Ng CA, Hungerbuhler K. (2013). Bioconcentration of perfluorinated alkyl acids: How important is specific binding? Environmental Science and Technology 47:7214–7223.
- 105
Martin JW, Mabury SA, Solomon KR, Muir DCG. (2003). Bioconcentration and tissue distribution of perfluorinated acids in rainbow trout (Oncorhynchus mykiss). Environmental Toxicology and Chemistry 22:196–204.
- 106
Armitage JM, Erickson RJ, Luckenbach T, Ng CA, Prosser RS, Arnot JA, Schirmer K, Nichols JW. (2017). Assessing the bioaccumulation potential of ionizable organic compounds: current knowledge and research priorities. Environmental Toxicology and Chemistry 36(4):882–897.
- 107
Mansouri K, Cariello, NF, Korotcov A, Tkachenko V, Grulke CM, Sprankle CS, Allen D, Casey WM, Kleinstreuer NC, Williams AJ. (2019) Open-source QSAR models for pKa prediction using multiple machine learning approaches. Journal of Cheminformatics 11:60.
- 108
Franco A, Ferranti A, Davidsen C, Trapp S. (2010) An unexpected challenge: Ionizable compounds in the REACH chemical space. International Journal of Life Cycle Assessment. 15:321–325.
- 109
U.S EPA. (2012) TSCA Work Plan Chemicals: Methods Document. U.S. Environmental Protection Agency, Office of Pollution Prevention and Toxics. https://www
.epa.gov/sites /production/files /2014-03/documents /work_plan_methods_document_web_final .pdf - 110
US EPA (2011) Design for the Environment Program Alternatives Assessment Criteria for Hazard Evaluation Version 2.0. https://www
.epa.gov/saferchoice /alternatives-assessment-criteria-hazard-evaluation. Accessed 09/24/18 - 111
Vegosen L, Martin TM. (2020). An Automated Framework for Compiling and Integrating Chemical Hazard Data. Clean Technologies and Environmental Policy 22(2):441–58.
- 112
Clean Production Action (2018). Greenscreen for Safer Chemicals Hazard Assessment Guidance version 1.4. https:
//greenscreenchemicals .org/method/full-greenscreen-method - 113
ECHA, REACH compliance checks: https://echa
.europa.eu /regulations/reach /evaluation/compliance-checks - 114
- 115
- 116
- 117
Black JA, Birge WJ, McDonnell WE, Westerman AG, Ramey BA, Bruser DM. (1982). The Aquatic Toxicity of Organic Compounds to Embryo-Larval Stages of Fish and Amphibians. Research Report No.133, Water Resources Research Institute, University of Kentucky, Lexington, KY.
- A Proof-of-Concept Case Study - A Proof-of-Concept Case Study Integrating Public...A Proof-of-Concept Case Study - A Proof-of-Concept Case Study Integrating Publicly Available Information to Screen Candidates for Chemical Prioritization under TSCA
Your browsing activity is empty.
Activity recording is turned off.
See more...