Included under terms of UK Non-commercial Government License.
NCBI Bookshelf. A service of the National Library of Medicine, National Institutes of Health.
Harrison DA, Ferrando-Vivas P, Shahin J, et al. Ensuring comparisons of health-care providers are fair: development and validation of risk prediction models for critically ill patients. Southampton (UK): NIHR Journals Library; 2015 Oct. (Health Services and Delivery Research, No. 3.41.)
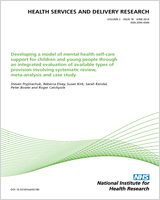
Ensuring comparisons of health-care providers are fair: development and validation of risk prediction models for critically ill patients.
Show detailsIntroduction
With the development of advanced circulatory support technologies and strategies, cardiothoracic critical care has developed into its own distinct specialty. In the UK, cardiothoracic critical care units are the principal areas where both complicated surgical and medical cardiac and thoracic admissions are managed.
The traditional risk prediction models used for adult general critical care may not be applicable to cardiothoracic critical care units for the following reasons. First, the majority of admissions to cardiothoracic critical care units are cardiac surgery cases, which were predominantly excluded from earlier versions of commonly used risk prediction models.34 Although admissions following cardiac surgery were not excluded from the development of the ICNARC model,14 the model was developed using data from adult, general critical care units only and, therefore, any cardiac surgery cases included would probably be atypical. Second, the pathophysiological mechanisms underlying organ failure in the cardiac surgery population are inherently different from those seen in the adult, general critical care population, where admissions are most commonly due to sepsis or respiratory failure.35 As such, the physiological variables that constitute the risk scores may not be calibrated for a population consisting mainly of cardiac surgery patients.
The majority of critical care units participating in the CMP are adult general units; however, an increasing number of cardiothoracic critical care units are now joining. Although we ultimately aim to produce a single risk prediction model that performs well across all types of critical care units, we believe that a model specifically focused on this unique group of patients would complement this output. This chapter therefore reports on the development and validation of a risk prediction model to predict acute hospital mortality for admissions to cardiothoracic critical care units.
Methods
Inclusion and exclusion criteria
For the development data set, data were extracted from the CMP database for all admissions to cardiothoracic critical care units between 1 January 2010 and 31 December 2012. Patients aged less than 16 years and readmissions to the critical care unit within the same acute hospital stay were excluded.
The validation data set consisted of admissions to cardiothoracic critical care units between 1 January 2013 and 30 June 2014. The same exclusion criteria were applied.
Outcome and candidate predictors
The outcome for the risk prediction model was acute hospital mortality, defined as death before final discharge from acute hospital and including deaths after direct transfer to another acute hospital from the hospital housing the critical care unit.
Candidate predictors were chosen based on expert clinical opinion and availability in the CMP database. The candidate predictors included were as follows: age; sex; severe conditions in the past medical history; dependency prior to admission to acute hospital; CPR within 24 hours prior to admission to the critical care unit; location prior to admission to the critical care unit; highest heart rate; mean arterial pressure (from the blood pressure measurement with the lowest SBP); highest temperature (central measurement or, if none available, non-central + 0.5 °C); ratio of PaO2 to fraction of inspired oxygen (FiO2) (from the arterial blood gas with the lowest PaO2); lowest arterial pH; partial pressure of carbon dioxide in arterial blood (PaCO2) (from the arterial blood gas with the lowest pH); highest blood lactate concentration; highest urea value; highest creatinine value; lowest sodium value; highest potassium value; lowest haemoglobin value; lowest white blood cell (WBC) count; lowest platelet count; lowest total GCS score; and mechanical ventilation status.
Severe conditions in the past medical history were defined according to the APACHE II method9 and categorised as liver disease, renal disease, cardiovascular disease, respiratory disease, metastatic disease, haematological malignancy and immunocompromisation. Conditions must have been evident in the 6 months prior to admission to the critical care unit. Dependency prior to admission to acute hospital was assessed according to the ability to complete activities of daily living, categorised as the ability to live without assistance in daily activities, with some (minor or major) assistance with daily activities or with total assistance with all daily activities. Location prior to admission was categorised as theatre, ward (including intermediate care areas), other critical care unit or emergency department. Admissions from theatre were further categorised as following elective/scheduled or emergency/urgent surgery. Where indicated among candidate predictors as lowest or highest, physiological predictors were the lowest or highest value from the first 24 hours following admission to the critical care unit.
Handling of missing data
Although moderate (from 0.1% to 3.2%), missing data were imputed to address potential bias and loss of precision. Fully conditional specification (FCS)36 was used as the multiple imputation method. All the candidate predictors (with or without missing values)37 and the outcome,38 as well as auxiliary variables related to missingness,39 were entered into the imputation model. When required, simple or zero-skewness log-transformation for non-normality was used. Unless the rate of missing information is unusually high, there tends to be little or no practical benefit to using more than 10 imputations40 and so, in the following analysis, 10 repeat imputations were performed. The examination of the imputed data showed the distribution to be broadly similar to that of the observed data, indicating no obvious problems with the imputation process.
Model development
The distributions of all candidate predictors were explored in patients with and without the primary outcome.
For modelling continuous predictors, different approaches were considered, including fractional polynomials, restricted cubic splines and generalised additive models. The best functional form for each predictor was selected based on fit, plausibility, accuracy and clinical knowledge.
After appropriate functional forms were decided in the univariable setting, a full multivariable model, with all continuous and non-continuous predictors, was fitted to determine the association between the predictors and the outcome. This model was redefined by removing predictors with no significant global effect. To test predictors’ global significance and individual linearity, Wald tests (based on Wald statistics for pooled estimates) were applied. Predictors that were non-significant at a cut-off p-value of 0.1 were discarded. The model was refitted and the remaining predictors were retested. The process continued until all the predictors in the model were significant. Using the resultant model as a starting point, a parsimonious model was developed using a backward elimination strategy. At each step one predictor was dropped from the model while comparing the c-index22 and Brier score.28 The 10 performance estimates (from the 10 multiply imputed data sets) were averaged and their variances pooled according to Rubin’s rules.41 The least significant predictor was removed and the process continued until no predictors remained in the model. The final model was chosen to balance parsimony and model performance.
As the majority of patients were admitted to the cardiothoracic critical care unit directly from theatre following cardiac surgery, two expanded models with additional predictors and interactions were tested to improve model performance. The first expanded model included an additional predictor for the pathological or physiological process of the primary reason for admission to the critical care unit (e.g. congenital or acquired deformity, degeneration, dissection or aneurysm, obstruction) from the hierarchical ICNARC coding method among admissions following cardiac surgery.20 The second expanded model tested interactions between the physiological predictors and cardiac surgery as the primary reason for admission. A nominal p-value of 0.001 was used to retain interaction terms in the model. The enhanced models were tested for improvements in discrimination and calibration.
In order to further evaluate the expanded models, net reclassification improvement (NRI) was determined. Reclassification has been proposed as a measure of utility or improvement in a risk prediction model.42 The proportions of patients with and without the outcome reclassified into lower- or higher-risk categories are compared. The NRI is defined as the proportion of non-survivors moving to a higher-risk category minus the proportion moving to a lower-risk category plus the proportion of survivors moving to a lower-risk category minus the proportion moving to a higher-risk category.
The final model coefficients were estimated using Rubin’s rules, to give a single estimate and standard error.
Model validation
The risk prediction model was then further validated in the temporally distinct validation data set. The 10 performance estimates of the final model were averaged and their variances pooled according to Rubin’s rules. The predictive performance of the model was estimated by bootstrapping the c-index and Brier score.43 Calibration was assessed by Cox calibration regression27 and graphically using calibration plots, with 20 equal-sized risk groups. Using Rubin’s rules, 10 linear predictions were calculated and averaged from the new model equation for each admission. The predicted probability of acute hospital mortality was calculated from this pooled result.
Statistical analyses were performed using Stata/SE, version 13.0.
Results
Available data
Between 1 January 2010 and 31 December 2012 there were 17,002 eligible admissions to five cardiothoracic critical care units participating in the CMP, which formed the development data set, and between 1 January 2013 and 30 June 2014 there were a further 10,238 eligible admissions to six cardiothoracic critical care units (one additional unit having joined the CMP), which formed the validation data set (Table 5).
TABLE 5
Characteristics of admissions included in the development and validation data sets for the risk prediction model for cardiothoracic critical care units
In the development data set, the majority of admitted patients were male (69%) with a median age of 66 years. Only 14.9% of admitted patients had any previous severe conditions in the past medical history, with the majority of those present being due to severe cardiovascular disease (8.6% of admissions). Most patients were fully functional prior to hospital admission (82.3%) and reported as needing no assistance with their activities of daily living. Over three-quarters of all admissions were surgical, of which 97% followed cardiothoracic surgery. Most surgery was elective or scheduled and most patients were ventilated during the first 24 hours following admission to the unit. The median length of critical care unit stay was 1.2 days, while the median total length of stay in acute hospital was 11 days. Critical care unit mortality was 7.4% and acute hospital mortality was 11.1%.
The validation data set had similar characteristics, although a lower proportion of admissions had one or more severe conditions in the past medical history (9.9%), particularly severe cardiovascular disease (4.8%), and a correspondingly higher proportion were fully functional prior to hospital admission (88.1%). Mortality was also lower, both in the critical care unit (6.4%) and in acute hospital (9.7%).
Model development
The best functional form for the relationship between each of the 15 continuous predictors and the outcome of acute hospital mortality was explored (see Table 6). All 15 predictors showed significant non-linearity (p < 0.001). Restricted cubic splines were chosen for the final modelling as they showed the best combination of flexibility and precision. To avoid overfitting spurious dips and unrealistic features of the curve, four knots were chosen to model the continuous predictors. When functional form was reassessed in the full multivariable model, the evidence for non-linearity in the relationship for blood lactate concentration was weak and so this predictor was finally analysed as linear. The final functional form for each predictor, including the position of the knots for restricted cubic splines, is shown in Table 6.
TABLE 6
Functional form of continuous predictors in the risk prediction model for cardiothoracic critical care units
The full multivariable model, including the 22 predictors, had a c-index and Brier score of 0.902 and 0.0635, respectively (Table 7). Of the initial 22 predictors, 18 were found to be associated with acute hospital mortality on multivariable analysis (p < 0.1): age; one or more severe conditions in the past medical history; dependency; CPR within 24 hours prior to admission; location prior to admission; heart rate; mean arterial pressure; temperature; PaO2/FiO2; arterial pH; PaCO2; blood lactate concentration; urea level; creatinine level; sodium level; WBC count; platelet count; and GCS score. Removal of the non-significant predictors resulted in minimal change to c-index and Brier score (see Table 7).
TABLE 7
Model selection process for the risk prediction model for cardiothoracic critical care units
The 18 significant predictors were entered into a stepwise model selection (see Table 7). The model which best balanced parsimony with precision consisted of 10 predictors: age; dependency; location prior to admission; mean arterial pressure; arterial pH; blood lactate concentration; creatinine level; WBC count; platelet count; and GCS score. The c-index and Brier score were 0.895 and 0.0656 respectively.
The first expanded model, incorporating reason for admission, performed moderately better than the baseline parsimonious model, with a c-index of 0.899 and Brier score of 0.0652 (see Table 7). The second expanded model, incorporating interactions between admission following cardiac surgery and blood lactate concentration, creatinine level and platelet count, demonstrated a c-index of 0.899 and Brier score of 0.0649 (see Table 7).
After comparing the reclassification of the two expanded models using risk categories defined by thresholds of 0%, 2%, 5%, 10%, 20% and 50% (Tables 8 and 9), the model with interaction terms was superior (Table 10). With this model, a total of 3677 (23%) admissions were reclassified and 2382 of those (65%) were placed in more appropriate categories. The total NRI for the expanded model with interaction terms was 11.1% (standard error 1.1%; p < 0.0001) compared with 6.5% (1.0%; p < 0.0001) for the expanded model with reasons for admission. The calibration regression for the expanded model with interaction terms demonstrated a slope of 0.98 and an intercept of −0.07, indicating a well-calibrated model. This was therefore taken as the final model. The coefficients for the final model are shown in Table 11.
TABLE 8
Reclassification table for the expanded model with reasons for admission compared with the parsimonious model for cardiothoracic critical care units
TABLE 9
Reclassification table for the expanded model with interaction terms compared with the parsimonious model for cardiothoracic critical care units
TABLE 10
Net reclassification improvement for the expanded models for cardiothoracic critical care units
TABLE 11
Final risk prediction model for acute hospital mortality among admissions to cardiothoracic critical care units
Model validation
The performance in the validation data set of 10,238 admissions from January 2013 to June 2014 was excellent: a c-index of 0.904 (95% CI 0.893 to 0.915) and Brier score of 0.055. The calibration of the model was satisfactory (Figure 4), with a calibration slope of 0.961 and a calibration intercept of –0.183.
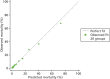
FIGURE 4
Calibration in the validation data set of the final risk prediction model for admissions to cardiothoracic critical care units.
Discussion
The case mix of admissions to cardiothoracic critical care units is different from that of admissions to adult general critical care units. Specific risk prediction models may aid benchmarking, performance improvement and resource allocation. Using a combination of baseline clinical and physiological predictors, collected in the first 24 hours following admission to a cardiothoracic critical care unit, a parsimonious model with good discrimination was developed and validated. The best-performing model combined age, dependency, prior location and seven physiological predictors, of which three had interactions with cardiothoracic surgery as the primary reason for admission.
There are several strengths to this study. First, this study is one of the few to examine cardiothoracic critical care units rather than isolated cohorts of cardiac or thoracic surgery admissions. Second, cardiothoracic critical care admissions were from five distinct cardiothoracic critical care units and included a large sample of admissions. To our knowledge, this is the largest study in terms of sample size from which a model has been developed. Finally, the data on risk factors are of high fidelity and the CMP database from which the data derive has been previously assessed to be of high quality.7 The main limitation of the study is the available data. Specifically, risk factor analysis and model development were limited by the data that have already been collected for the CMP.
Previous literature on risk prediction models in the cardiothoracic critical care unit setting is limited. There is a substantial body of literature on risk prediction for patients undergoing cardiac surgery with emphasis on preoperative risk prediction.44,45 By definition, preoperative risk prediction fails to account for intraoperative events and will therefore not necessarily be adequate for critical care risk prediction. There are also several studies examining postoperative risk factors in cardiac and thoracic surgery admissions, but these are limited in that they are mostly single-centre studies, with risk prediction models that have not been validated in a separate cohort.46,47 Badreldin et al. evaluated the Sequential Organ Failure Assessment (SOFA) score and Cardiac Surgery Score (CASUS) in a single-centre study and found high c-indices associated with both scores, but greater with CASUS.46 CASUS is promising in that it consistently demonstrates a high c-index, but has yet to be validated in a large multicentre cohort. Tamayo et al. developed the Post Cardiac Surgery (POCAS) score in a single-centre study which used four postoperative risk factors (mean arterial pressure, bicarbonate level, blood lactate concentration and the international normalised ratio) to model in hospital mortality and which demonstrated a c-index of 0.89.48 Again, the main limitation of this study is the bias inherent in a single-centre study design.
Several multicentre studies have been conducted in an attempt to predict outcomes using postoperative risk factors. Becker et al. evaluated the APACHE III model in admissions to a critical care unit after coronary artery bypass surgery in a multicentre cohort study.49 Using a model that included the acute physiology score of the APACHE III model and baseline clinical risk factors, a c-index of 0.85 was demonstrated for acute hospital mortality. Similarly, Simchen et al.50 tested multiple models, which included pre-, intra- and postoperative risk factors, to predict 30-day mortality in patients with coronary artery bypass surgery admitted to 14 units. The best model that emerged was the one which included postoperative risk factors with a c-index of 0.92. Gomes et al.51 developed a model in a multicentre cohort, which included PaO2/FiO2, vasopressor and inotrope use, mechanical ventilation and pre- and intraoperative risk factors, to predict acute hospital mortality in cardiac surgery admissions with a c-index of 0.84. Multiple other studies have examined the association between mortality and postoperative risk factors in multicentre cohort studies in both cardiac and thoracic surgery admissions. Significant postoperative risk factors included creatinine level, serum glucose concentration, number of blood transfusions received, low cardiac output, stroke, reoperation, intra-aortic balloon pump use, organ failure, mechanical ventilation time and serum transaminase levels.52–58 All these studies examined postoperative risk factors in cardiac or thoracic surgery admissions. To our knowledge, this is the first study to examine postoperative risk factors in a cohort of cardiothoracic critical care admissions to multiple cardiothoracic critical care units.
In addition to using postoperative physiological predictors in our model, we attempted to augment its predictive ability by adding interaction terms to it that would account for the unique physiology of the post cardiac surgery patient. The injury in cardiac surgery is usually a result of the cardiopulmonary bypass and, in comparison with patients with sepsis, for example, it is transient and often reversible. To account for these differences we interacted physiological predictors with cardiac surgery as the primary reason for admission to the critical care unit. This expanded model was better able to predict and classify patient outcomes in cardiothoracic critical care units.
Models specifically designed for cardiothoracic critical care units may be warranted given the differences in case mix of cardiothoracic critical care units compared to that of adult, general critical care units. A parsimonious model using a combination of clinical and physiological variables collected in the first 24 hours following admission was shown to have good discrimination and calibration, and will serve as a benchmark for establishing whether or not use of a single risk prediction model across all types of adult critical care units is appropriate in these highly specialised units.
- Development and validation of a risk prediction model for admissions to cardioth...Development and validation of a risk prediction model for admissions to cardiothoracic critical care units - Ensuring comparisons of health-care providers are fair: development and validation of risk prediction models for critically ill patients
Your browsing activity is empty.
Activity recording is turned off.
See more...