NCBI Bookshelf. A service of the National Library of Medicine, National Institutes of Health.
Bion J, Aldridge C, Beet C, et al. Increasing specialist intensity at weekends to improve outcomes for patients undergoing emergency hospital admission: the HiSLAC two-phase mixed-methods study. Southampton (UK): NIHR Journals Library; 2021 Jul. (Health Services and Delivery Research, No. 9.13.)

Increasing specialist intensity at weekends to improve outcomes for patients undergoing emergency hospital admission: the HiSLAC two-phase mixed-methods study.
Show detailsIntroduction
In 2013, NHS England published The NHS Belongs to the People: A Call to Action,130 in which it called for a public debate on how to develop new ways of working in the health service to respond to the financial crisis, given a funding gap of around £30B between projected spending requirements and resources available between 2013/14 and 2020/21. In the event, the government’s 2015 spending review indicated that additional funding for the NHS, part of which was intended to facilitate the introduction of 7-day services, would have to be accompanied by savings of £22B, suggesting that trusts were likely to be expected to find at least some of the funding for 7-day services from existing resources.131 However, the true cost (including opportunity costs) of implementing 7-day services was unknown, and the potential benefits are uncertain and unquantified. The cost of training a doctor to the level of consultant was estimated to be £510,411 in 2016, with an annual basic salary of £89,046 and cost per hour of £105.132 Given the emphasis in the 7-day service standards on expanding consultant input at weekends, it was clear that a major cost component for individual trusts to consider would be doctors’ salaries. The HiSLAC study provided an opportunity to examine the cost-effectiveness of this element of 7-day services on the quality of emergency care.
Economic evaluation of medical products and technologies is standard practice in developed health-care systems,133,134 generally extrapolating effectiveness estimates from randomised controlled trials to generic health end points, such as QALYs. Historically, less attention has been paid to service delivery interventions affecting staffing and system organisation, although this is now starting to change.135 Service delivery interventions are complex, act further upstream of the patient than drugs or devices, and may have diffuse ‘downstream’ effects. They therefore require large sample sizes for accurate estimation and pose potential difficulties for causal interpretation.136
The level of specialist intensity is a good example of a complex service delivery intervention acting through multiple causal pathways. It has both direct and indirect effects, that is acting through a mediating variable or not, respectively.137 Specialist presence affects, for example, both the acute phase of stabilisation, diagnosis and treatment at admission, and the subsequent risk of experiencing preventable harm. Indeed, it was this mechanism that motivated the policy. As discussed in Chapter 1, the observed increased risk of mortality among weekend admissions was most frequently assumed to be a consequence of a reduction in care quality. However, the level of specialist presence is also likely to be correlated with the health of the patient population.106 A single regression model of mortality on day of admission, as much of the weekend effect literature is based on, cannot separate these two effects, a problem discussed widely at the time.138 However, a structural equation modelling approach can be used to specify models based on causal hypotheses of the relationships between observed and latent variables.139–141
In this chapter, we present results from a cost–benefit analysis of a policy of changing the specialist-to-patient ratio, based on a structural equation modelling method. Our objective in using this approach was to develop, compare and estimate models that accounted for the different possible pathways through which specialists might affect the relevant outcomes. By jointly estimating models from across the causal pathway we could separate out the effects of unobserved patient health from those needed for cost–benefit analysis, subject to some key assumptions. We took a Bayesian approach to model specification, estimation and interpretation, which permits the incorporation of prior information and knowledge, typically affords the estimation of more complex joint and hierarchical models, and naturally fits into a decision-making framework.
Aim
The aim was to determine whether or not implementing a policy of increasing specialist intensity at weekends to the level observed in ‘high-intensity’ hospitals is cost-effective.
Objectives
- Develop candidate qualitative causal models.
- Estimate and compare structural equation models derived from the causal models.
- Estimate the expected net benefits of increasing specialist intensity.
- Determine the probability that net benefits are positive at different cost-effectiveness thresholds.
Methods
Data
Quality of care was assessed from the case record review (see Chapter 6, Results). For each case record, a binary indicator was derived for whether or not each patient experienced each of the nine different types of error, and whether or not they were judged to have experienced an adverse event. Age, sex and mode of arrival at the hospital were also extracted. Mode of arrival has been previously shown to be an important indicator of patient severity, particularly in studies of the weekend effect.142 Where case records were reviewed twice, we randomly selected one review for inclusion. Overall, 4000 patients were included in the data set (as opposed to all 4800 reviews in Chapter 6). Mortality and length of stay for each patient were extracted from the HES database for each patient. Information on specialist intensity and change over time was taken from the HiSLAC annual PPSs (2013/14 to 2017/18) (see Chapter 4). The level of specialist intensity for the appropriate epoch, day of admission and hospital were matched to each patient.
Cost–benefit analysis
The ultimate outcome for this study was the expected net benefits of shifting from a low level of specialist intensity to a high level (‘high’ and ‘low’ were defined as the weekday and weekend averages, respectively). The expected net benefits are the change in benefits to patients resulting from this shift, measured in QALYs and valued at the societal willingness to pay per QALY, minus the change in costs. If the expected net benefits are positive, then this indicates that the policy generates more benefit in monetary terms than it costs and would be considered cost-effective.
Patient QALYs were not captured by our data, so we specify a distribution of possible QALY losses associated with the outcomes we do observe, namely adverse events and mortality, following the approach of Yao et al.143 Adverse events were divided into different severity categories based on a common classification.144 We used published data identified by systematic review to specify a distribution for the proportion of adverse events falling within each category, as well as for their duration and severity.145–147 The results of this exercise are shown in Table 16.
TABLE 16
Estimated proportion and impact of adverse events across four severity categories
Counterfactual life expectancies were required to estimate the duration of life lost associated with a fatal adverse event. One study was identified that provided estimates of these counterfactuals.148 The same distribution was used for permanent disability as the effects would be expected to last for the remainder of an individual’s life. For temporary disability, in the absence of further information, we specified a distribution with a median of 3 months and with 95% of patients recovered by 1 year. For minimal harm, we specified that the duration is uniformly distributed between 1 day and 1 month. The quality-of-life value associated with each year lost associated with mortality was equivalent to reported quality-of-life values at the age the patient would have otherwise attained, which we estimated from Ham.149 A sample weighted by these age groups was taken from the Health Survey for England150 for the years in which the survey reported EQ-5D-derived health-related quality-of-life weights (1996, 2003–6, and 2008). The mean (SD) quality-of-life weight was 0.64 (0.25). Quality-of-life weights for non-fatal adverse events were based on evidence from studies of representative conditions.
For the cost side of the equation, there were both direct and indirect costs to consider. The direct cost is the additional expenditure required to employ specialists at a ‘high’ level. To specify a distribution for this, we considered the pay scales (approximately £80,000–110,000), clinical excellence award levels (approximately £3000–80,000) and superannuation costs (21%) for specialists in NHS England.151 There is no information in the public domain about the distribution of specialists across pay scales or clinical excellence awards. We therefore take the top end of the pay scale (with superannuation costs) as a mean point, and allow variation to cover the range of possible pay and awards. Based on a whole-time equivalent of 40 hours per week for 46 weeks per year, we specified that an additional hour of specialist presence has a mean cost of £77 and SD of £10. The indirect cost is the change in the cost of treatment due to an adverse event. We based this on any estimated change in length of stay due to an adverse event multiplied by the mean cost of a non-elective excess bed-day reported in the most recent publication of the NHS 2017/18 Reference Costs,152 which was £373.
Model development
To support the development of the model, an expert workshop and focus group was convened initially to discuss the mechanisms by which increasing the intensity of specialist input at weekends might affect patient outcomes. Based on this discussion and on previous literature, a candidate set of three models of increasing complexity was developed (Figure 23). All of the models included the causal effect of specialists on the risk of experiencing each of the nine types of error and a direct effect on length of stay due to early or delayed discharge. Model 1 has these two pathways only, so that the only mechanism by which specialists could influence adverse events and mortality is by affecting the risk of an error. Unobserved patient health was also included, which had an effect on the risk of mortality and overall length of stay only. However, model 1 may be unrealistic, sicker patients may be taken to hospitals with greater specialist presence or, conversely, less severely ill patients may not be admitted when specialist levels are lower. Model 2, therefore, extends model 1 by allowing for a relationship between the specialist-to-patient ratio and patient health. So, for example, an increase in specialist presence could affect length of stay through three mechanisms: changing the risk of errors, changing the risk of delayed discharge or correlating with the health of the patient population. Model 3 adds an additional extension by allowing for a relationship between patient health and the risk of each type of error and the risk of experiencing an adverse event. More severely ill patients may be at higher risk of experiencing an error as they are exposed to a greater number of interventions and have more complex conditions.

FIGURE 23
Three candidate models.
Structural equation model
We used a Bayesian approach to model design, specification and estimation. A Bayesian statistical model has two components: a model for the data – the likelihood – and prior distributions of the model parameters. The posterior distribution of the model parameters, which are used for inference, is directly proportional to the product of the likelihood and prior distribution. The likelihood here is a structural equation model and the qualitative causal models can be used to specify the structural equation model. A structural equation model can be considered a joint regression model for a number of different outcomes with some shared parameters or latent variables. For example, in the models above, the same latent health term, α, would appear in models for outcomes including mortality and length of stay. For each outcome, we can specify what goes into its constituent part of the joint model by examining all of the other variables whose arrows point into it. In model 1, for example, the model for mortality has an indicator for adverse events and patient health.
For dichotomous outcomes (mortality, adverse event, error of each type), we specified binomial-logistic models; we used a Poisson regression model for length of stay and a linear model for specialist intensity. Where health appears in a model, we included patient sex, age, age squared and an indicator of whether or not the mode of arrival was by ambulance. We also included the hospital- and individual-level random effects (i.e. latent health effects). To allow for differing effects of the latent health variables in each model, these were multiplied by a model-specific parameter, typically known as ‘factor loadings’. In addition, as the hospital-level effects may change over time, we allowed for the random effect to be different but correlated in each epoch; this was specified as an auto-regressive relationship (of order 1).
The relationship between specialist intensity and either the risk of the various errors or length of stay was unlikely to be linear. For example, it is possible that there is a diminishing marginal benefit to additional specialists. However, there was little prior evidence on the ‘shape’ of this relationship. A flexible, non-parametric approach was therefore used for specialist intensity, which let the data dictate the shape of the function relating specialist intensity to each outcome. In particular, we used natural cubic splines. To ensure that this function was smooth (i.e. that it did not vary dramatically for small changes in intensity), we limited the degrees of freedom to 3. Internal knots were placed at the upper and lower thirds of the range of intensity.
Prior distributions
Prior distributions are used to capture knowledge about model parameters and quantify what values are plausible. For example, we know that it is completely implausible for the risk of death to be 10-fold higher among women than among men or vice versa. It is highly likely that any difference in risk between men and women will be less than 20 percentage points. The more precise the prior distributions, the more influence they have over the posterior distributions and, hence, our inferences about parameter values. We wanted to specify prior distributions that ruled out extreme, implausible values but had little influence on the posterior distributions within the plausible range to let the data ‘do the talking’. These are sometimes referred to as ‘weakly informative’ prior distributions.153 They also facilitate computation and model estimation, compared with so-called non-informative prior distributions that allow for extreme and implausible values.
The splines used to flexibly model the effect of specialist intensity have two parameters that dictate their shape. However, little prior information exists on the effect of specialist presence on patient safety outcomes, although recent evidence suggests that it may be negligible.154 Our priors for the effect of specialist intensity are partially informed by an expert elicitation exercise that we conducted on 20 September 2018 with 19 specialists in acute medicine to ascertain expert beliefs about the magnitude of the effect of shifting specialist intensity. The elicited beliefs do not map directly onto model parameters for the effect of specialist intensity as the parameters of the spline function do not have any ‘natural’ interpretation. However, the elicited information did inform us that any changes in specialist intensity are unlikely to be associated with large changes in the risk of errors or changes to length of stay. For example, the expert panel expected the effect of doubling specialist intensity from weekend to weekday averages to lead to a 25% (95% CrI 10% to 40%) reduction in the risk of a diagnostic error and a 5% (95% CrI –5% to 15%) reduction in length of stay. On this basis, we specify standard normal priors for the spline parameters, which rule out large shifts in risk for small changes in specialist intensity but allow for a range of functional shapes, including increases, decreases and no change in risk. For the remaining model parameters, we adopted weakly informative N(0, 5) prior distributions and, for the SDs of random-effect terms, we specified an inverse-gamma distribution with shape and rate parameters of 1.
Model comparisons
Three candidate structural equation models were specified from the qualitative models. Although the more complex model might be more realistic, it might be too complex and have too many parameters for the data to tell us anything meaningful about their values. A very complex model with a large number of parameters may be able to successfully replicate the data used to estimate it, given the flexibility afforded by the sheer number of parameters, but be very poor at predicting new data. This problem is sometimes referred to as ‘overfitting’. We therefore compare the models using two statistics. First, the widely applicable information criterion assesses how closely predictions from a fitted model align with the data, but adds a penalty term for the number of parameters to avoid overfitting. Second, the leave-one-out cross-validation score examines the out-of-sample predictive ability of the model by determining how well the model would predict each data point if it were estimated with all of the remaining data (hence ‘leave-one-out’). In the protocol we stated that we would use posterior predictive model checks; however, these checks are poor at detecting overfitting and so were considered to be a bad choice for the required model comparison.
Expected net benefits
From the best-fitting model, we determined the estimated change in adverse events, mortality and length of stay associated with a change in specialist intensity, while adjusting for unobserved patient health. On this basis, we calculated the expected net benefits. The expected net benefits should be interpreted as being for a fixed patient population, that is what might happen if the level of specialist intensity were changed but there was no change in the patients admitted to a hospital. We considered the expected net benefits of, first, increasing mean weekend specialist intensity levels to the weekday level and, second, increasing specialist intensity incrementally to identify potential minimum and optimum levels.
Results
Summary statistics
Using data from the PPS, Figure 24 shows the levels of specialist intensity at the participating sites on Sundays and Wednesdays in the two epochs. Although the majority of sites were estimated to have increased their level of specialist presence at the weekend, most also reduced their specialist intensity on a weekday. Across the 20 trusts, the mean level of specialist intensity at the weekend increased from 25.1 hours per 10 EAs to 27.7 hours, but on weekdays intensity declined (52.7 and 49.8 hours per 10 EAs, respectively). As we have shown (see Chapter 4), the reduction in weekday intensity is explained partly by a disproportionately greater increase in Wednesday EAs than in Sunday admissions between the two epochs, but it is likely that specialists have transferred a proportion of their weekday work to weekends.

FIGURE 24
Mean specialist intensity by trust and epoch, hours per 10 EAs.
Summary statistics of the sample of 4000 admissions by epoch and day of admission are shown in Table 17. There were some changes in the patient population over the two epochs. The proportion of patients arriving by ambulance declined at weekends and on weekdays over time, although it remained relatively larger at the weekend. The proportion of patients experiencing almost all types of error also declined over time; there was no clear pattern showing that the number of errors or adverse events were consistently larger for weekend or weekday admissions. Mortality among weekend admissions was higher than that among weekday admissions in both epochs.
TABLE 17
Summary statistics of the sample by epoch and day of admission
We can examine the relationship between specialist intensity and markers of patient health to support or refute different models. Figure 25 shows the association between specialist intensity and patient age and the proportion of patients arriving by ambulance. There is evidently a relationship, which would initially refute model 1, as it provides evidence that patient health is associated with level of specialist intensity.
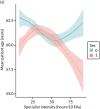
FIGURE 25
(a) Relationship between specialist intensity and patient age (b) and the proportion of patients arriving by ambulance.
Model comparisons
Model 3 was the preferred model under both comparison statistics. Model 2 was a large improvement over model 1, and model 3 was a (smaller) improvement over model 2. Figure 25 shows the estimated adjusted relationships between specialist intensity and the risk of each of the nine types of error from the three models. For models 1 and 2, which do not allow for patient health to affect the risk of error, there is little evidence of a relationship between specialist intensity and the risk of each type of error (see Appendix 19). For model 3, higher levels of specialist intensity are associated with a reduced risk of diagnostic, communication, medication, monitoring and management errors. For example, an increase in specialist intensity from 25 to 50 hours per 10 EAs is associated with a reduction in the odds of a diagnostic error of 26% (95% CrI 2% to 45%). These results suggest that there is a degree of confounding in models 1 and 2 as we expected; higher levels of specialist intensity are associated with both a lower risk of error and poorer health among patients, and poorer health is associated with a higher risk of error.
Little evidence of a relationship was seen between specialist intensity and the risk of experiencing an adverse event, and there was a large degree of uncertainty about any effects. The risk of an adverse event was very low (1.35%; see Table 17). Diagnostic errors were the most likely to lead to harm; the risk of an adverse event was approximately 10% with a diagnostic error, compared with the next most common error, treatment/management errors, which had a risk of < 1%. As a result, the doubling of specialist intensity from 25 to 50 hours per EAs was associated with a reduction in the number of patients experiencing adverse events only by approximately 2 in 1000. For the same reason, there was little evidence of a relationship between specialist intensity and mortality. Although experiencing an adverse event was associated with an increased risk of mortality in all models, given the relatively low risk of both mortality and adverse events, the effects of specialist intensity are very small. For the same doubling of specialists described above, we would expect five fewer deaths per 10,000 EAs on average.
Figure 26 shows the posterior predictive distribution of length of stay compared with specialist intensity. Both models 2 and 3, which allowed for latent health to be correlated with specialist intensity, mortality and length of stay, showed a shorter expected length of stay with higher levels of specialists. Indeed, the effects were, qualitatively, highly similar, although they were associated with wide CrIs. Most of the observed effect was from the direct effect of specialists rather than from a reduced risk of adverse events.

FIGURE 26
Posterior predictive distribution of length of stay and specialist intensity: all models.
Cost–benefit analysis
Model 3 was selected as the best-fitting model and was therefore used for the cost–benefit analysis. As Figure 24 and Table 17 show, the 7-day services policy had little discernible effect on the level of specialist intensity in these 20 trusts. However, we can examine the expected net benefits of the policy, had it been implemented with greater fidelity, by predicting the outcomes from model 3 at a level of specialist intensity of 26.9 hours per 10 EAs (the weekend average) and 48.4 hours per 10 EAs (the weekday average). The posterior mean expected net benefit was £177, indicating that the policy is cost-effective at a willingness to pay per QALY of £20,000.
On average, the policy would cost £167 per EA, generate £5 per EA in QALYs from the reduced risk of an adverse event, and save £349 as a result of a reduced length of stay. Model 3 predicted that the length of stay would be reduced by an average of 0.9 days under this policy. Given that QALY gains made a relatively small contribution to the overall expected net benefits, the model was not affected by the choice of cost-effectiveness threshold.
To consider more generally the cost–benefit of increasing specialist intensity from this model, we next examined the effects of increasing specialist intensity incrementally from its lowest to highest observed values. Figure 27 shows the expected net benefits of increasing the level of specialists by 10 hours per 10 EAs. The expected net benefits were greater than zero across the range of specialist intensity. However, they were flat and close to zero above approximately 35 hours per 10 EAs, and the 95% CrI was fairly wide, with a substantial area below zero. Below 35 hours per 10 EAs, there is much stronger evidence that increasing specialist intensity is cost-effective.

FIGURE 27
Posterior predictive distribution of expected net benefits of raising specialist intensity by 10 hours per 10 EAs with 95% CrI.
Discussion
As described above, one of the main aims of the 7-day services policy was to improve care quality for patients admitted to hospital at the weekend. However, the confounding of the relationship between specialist intensity and care quality by patient health status complicates the task of identifying the effect of the policy from observational data. Indeed, this is a general problem in the evaluation of service delivery interventions, which, by definition, have effects that are mediated by other services and have clinical processes that are diffuse but affect a large proportion of the patient population.136 The policy was not implemented as designed, and so the expected within-hospital variation in specialist intensity that we planned to use to identify the effects of the policy was not present. However, significant variation in specialist intensity levels between hospitals and by day of admission was present, and this enabled us to examine the relationship of this variation and patient outcomes. Implicit, however, in these analyses is the assumption that any other changes in care quality by day of admission were constant across hospitals in each epoch, which may be a strong assumption.
For this study, we developed structural equation models for this type of evaluation, which enabled us to disentangle the different causal pathways. In doing so, our evidence supports the hypothesis that an increase in the level of specialist intensity is associated with reductions in the risk of errors and adverse events, which is confounded by patient health. However, the effect is small and certainly not large enough to justify the potential outlay required to implement the policy. This agrees with a number of previous studies and discussions that have been published based on cruder analyses.29 A more significant benefit is estimated in the reduced length of stay associated with increases in specialist intensity, which is potentially large enough to make the policy cost-effective, particularly when specialist intensity is low.
We estimated that increasing specialist intensity at the weekend to weekday levels would reduce the average length of stay by almost a day, thereby significantly reducing the cost of patient care. The qualitative analysis (see Chapter 6) also identified delayed discharge as a key issue at the weekend that results from a lack of specialists. However, the system-level implications of reducing length of stay have not been explored in detail. Overall patient volume and bed occupancy would be unlikely to reduce, but throughput would increase. This would imply an improved efficiency of the health service and potentially improved patient experience as a result of less unnecessary time spent in the hospital, although we did not account for patient experience in our analysis here. Any improvement in care quality would also affect the patient experience, although we reiterate that significant adverse events were exceedingly rare. The types of error that the analysis suggested would be impacted by specialist presence also aligned with those identified in our focus group exercise, further validating the evidence.
There has been growing interest in the evaluation of service delivery interventions.155,156 Watson and Lilford,156 Lilford et al.136 and Watson et al.157 have discussed the necessity of capturing and integrating data from across the causal chain to estimate policy relevant effects and conduct economic evaluations. The analyses in this chapter exemplify how this might be done in practice using a structural equation modelling approach. However, we caution that, in the absence of an experimental methodology, the results cannot necessarily be interpreted as causal effects. Even the more complex models are simplifications of the health system and may miss issues of confounding or reverse causality. However, as we discussed, the data become less and less informative about model parameters as complexity increases. A method of principled Bayesian analysis using qualitative model development and comparison aims to deal with this problem.158 We believe that our analysis provides the most reliable evidence to date of the cost–benefit of increasing specialist intensity.
We reiterate the issues with the data discussed in previous chapters. Response rates to the PPS were variable and often low. The consequences of this could be significant in terms of bias if the response rate were systematically dependent on other key variables, such as patient health or specialist intensity. However, we do not have reason to believe that this is the case and the results have strong face validity. Although the inter-reviewer reliability of subjective case note review was low at the individual level (see Chapter 6, Case record review: results), it was high when aggregated at trust level and by epoch. By the same reasoning, we expect the ‘noise’ to cancel out in the aggregate. Our results here focus on average effects, and the effect of the high variation in case note review is to increase our uncertainty around these effects rather than to introduce any systematic bias.
Had the 7-day services policy been implemented as designed, the evidence presented in this chapter suggests a high probability that it would have been cost-effective. This may seem to contradict previous findings and discussions (e.g. Godlee,138 Meacock et al.29), which determined that improvements in quality and reductions in mortality would be unlikely to justify the policy on cost-effectiveness grounds. We would agree with this assessment, but disagree with the overall conclusions, as through reductions in length of stay the policy would likely be justifiable. Thus, we would tentatively conclude that the 7-day services policy was right, but for the wrong reasons.
- Health economics evaluation of increasing the weekend-to-weekday specialist inte...Health economics evaluation of increasing the weekend-to-weekday specialist intensity ratio in hospitals in England - Increasing specialist intensity at weekends to improve outcomes for patients undergoing emergency hospital admission: the HiSLAC two-phase mixed-methods study
Your browsing activity is empty.
Activity recording is turned off.
See more...