This work was produced by Guthrie et al. under the terms of a commissioning contract issued by the Secretary of State for Health and Social Care. This is an Open Access publication distributed under the terms of the Creative Commons Attribution CC BY 4.0 licence, which permits unrestricted use, distribution, reproduction and adaptation in any medium and for any purpose provided that it is properly attributed. See: https://creativecommons.org/licenses/by/4.0/. For attribution the title, original author(s), the publication source – NIHR Journals Library, and the DOI of the publication must be cited.
NCBI Bookshelf. A service of the National Library of Medicine, National Institutes of Health.
Guthrie B, Rogers G, Livingstone S, et al. The implications of competing risks and direct treatment disutility in cardiovascular disease and osteoporotic fracture: risk prediction and cost effectiveness analysis. Southampton (UK): National Institute for Health and Care Research; 2024 Feb. (Health and Social Care Delivery Research, No. 12.04.)
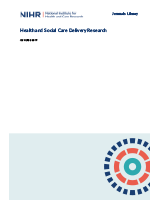
The implications of competing risks and direct treatment disutility in cardiovascular disease and osteoporotic fracture: risk prediction and cost effectiveness analysis.
Show detailsType of first cardiovascular event
We fitted a multinomial logistic regression model to the CPRD data set (which excluded people who have previously experienced cardiovascular events or received statins) to estimate the relative probabilities of a cardiovascular event being of each type, according to sex, age at event and baseline QRISK3-predicted risk. Multinomial logistic regression provides a way of predicting the likelihood of each of a number of possible events in a single statistical model. In logistic regression, the probabilities of each event are output as log-transformed odds of the events against a reference event. Accordingly, for each included risk factor, the model estimates a separate coefficient for each event, which can be interpreted as a log-transformed OR. Exponentiating these coefficients gives the ratios by which a unit change of the corresponding risk factors changes the odds of the event against the reference.
Table 47 shows the output from the multinomial regression model that we constructed, for which cardiovascular death was the reference event. Applying the coefficient values for an event type to a set of covariate values reflecting a cohort’s risk factors gives the log-odds between that event and cardiovascular death for the cohort.
TABLE 47
Multinomial regression estimating type of first cardiovascular event as a function of age at event, sex and cardiovascular risk
To give a worked example, consider 50-year-old women with a 10-year QRISK3-predicted CVD risk of 10%. For women in this subgroup who experience a cardiovascular event, we can calculate the log-odds that this event will be a MI compared with cardiovascular death as follows. For each unit of age, one unit of the corresponding coefficient for MI (set at its mean value) is added (not multiplied because, before exponentiation, we are working on a logarithmic scale) to the intercept: 4.2883 + 50 × −0.1052. For women, we do not apply the ‘male’ coefficient. The transformations and interactions described in Table 47 must be applied to age, sex and QRISK3-predicted risk before applying all other coefficients in the same way. Let I be the set of cohort characteristics (i.e. women, 50 years old, 10% QRISK3). Therefore, the final equation is:
We exponentiate to give the odds of MI against non-cardiovascular death for covariates I:
Once we have calculated the odds of each event against the reference, we may calculate the absolute probabilities of each event as follows. First, we express the absolute probability of the event being a MI as a multiple of the absolute probability of the event being cardiovascular death:
Repeating steps 1–3 for each other event gives:
People who had a cardiovascular event recorded in the CPRD data set must have had one of these types of events or be classified as a cardiovascular death. Therefore, the sum of these absolute probabilities, along with that of cardiovascular death, must equal 1:
Now, we can substitute the values from Equation 7 into Equation 8 and use the result to calculate the probability of the reference event: cardiovascular death:
Finally, we compute the absolute probabilities of each event from Equation 7 using the value we calculated for P(CV death)I:
Figure 36 illustrates the results of the model for men and women at a range of ages and underlying levels of cardiovascular risk. For comparison, Figure 36 also shows the raw distribution of first events in the CPRD data to which we fitted the model. Strokes and cardiovascular deaths become more common first events as people get older. In contrast, the probability that the first sign of CVD will be an acute coronary event (MI or UA) is highest in younger people. Predicted cardiovascular risk at baseline and sex have relatively little influence on the distribution of events.

FIGURE 36
First CVD event stratified by age, sex and CVD risk: predictions from multinomial model compared with observed data from CPRD.
The events available in the CPRD data set comprise only events used by QRISK3 as predictors (i.e. SA, UA, MI, stroke, TIA and cardiovascular death). Therefore, we had to replicate the approach used in CG181 of combining predicted events within each cohort with external evidence estimating age- and sex-specific rates of PAD169 and heart failure.170
To extend our example of a 50-year-old woman, this evidence suggests that, for women in the age bracket of 45–55 years, there are 6.3% as many cases of heart failure and 62.5% as many cases of PAD as QRISK3 events. This means that the QRISK3 events accounted for: 1/[P(PAD)I + P(HF)I + 1] = 0.593. Multiplying this number by our calculations from Equation 10 we arrive at our final proportions:
Health-related quality of life: underlying
We created the data set we used from a pooled sample of respondents from the HSE.133 The HSE is a nationally representative cross-sectional sample of people residing privately in England. Each year, respondents complete a set of core questions about their general health (including the EQ-5D-3L), their lifestyle choices and behaviour, as well as their personal characteristics. From year to year, additional modules are integrated within the HSE and respondents are asked detailed questions about particular topics. We pooled HSE data sets from 2003, 2006 and 2011, as these surveys focused on CVD, with respondents being asked whether or not they had ever been specifically diagnosed with CVD by a doctor. After pooling the data, we dropped individuals with missing data for age and EQ-5D-3L. The remaining sample (n = 26,400) included only people who reported having no doctor-diagnosed CVD.
To account for non-linearity in the relationship between age and quality of life, we explored a range of polynomial forms. We introduced sex as a covariate and tested various degrees of interaction with the age terms. The model that best described the data (i.e. the most parsimonious model with AIC within 3 points of the lowest observed) had a quintic form with full interaction with sex (equivalent to fitting separate models for men and women). Table 48 details the final model.
TABLE 48
Polynomial regression estimating quality of life in people aged 16–89 years with no history of CVD from HSE data
For people aged 90–100 years, we did not use modelled quality-of-life estimates and, instead, we relied on a simple average of values for men and women in that age group. We did this because data for nonagenarians are very scanty in the HSE data set (with only 52 women and 26 men represented) and, although it is clear that quality of life in the 10th decade is lower than in preceding years, there is no indication of a within-decade decline in this subpopulation of people with no cardiovascular history. Contrary to this, a continuous function fitted to all ages results in a dramatic drop-off in quality of life between 90 and 100 years (as the continuous function extrapolates the decline that is seen in the more plentiful data for previous decades). This can be solved by incorporating higher-order polynomial terms for age, but this results in implausible tail effects, where quality of life suddenly and markedly improves among the oldest people. We concluded that there are simply not enough data to assume any trends in within-decade quality of life for nonagenarians, and used a simple average instead (Table 49).
TABLE 49
Quality of life in people aged ≥ 90 years with no history of CVD from HSE data
Figure 37 provides a visualisation of the new fitted model and alternative approaches compared with the underlying data. It is clear that the linear decline assumed in CG181 provides a poor approximation, as it overestimates quality of life in the oldest and youngest people and underestimates it for people aged 40–85 years. The quadratic function suggested by Ara and Brazier156 provides a better fit, especially for women; however, it is less well suited to men, overestimating quality of life in the first three decades of adulthood and underestimating quality of life from age 60 to 90 years.

FIGURE 37
Underlying quality of life of people with no history of CVD: new polynomial model compared with linear assumption from CG181 and quadratic model from Ara and Brazier. (a) Women; and (b) men.
For similar reasons, for women up until age 85 years, our new quintic model mirrors Ara and Brazier’s156 quadratic model fairly closely, but differences are more apparent in men. In particular, the raw data suggest that – in this subgroup of men with no cardiovascular history (which will become an increasingly atypical group as age rises) – quality of life is fairly well preserved throughout middle age, and only begins to tail off appreciably in their 80s and 90s. This suggests that a good proportion of the expected decline in the quality of life of men as they age can be attributed to CVD itself (or other morbidities that are strongly correlated with cardiovascular events). The remaining men with no cardiovascular history – the population of interest for our primary prevention decision problem – appear to escape without much deterioration in their underlying utility, and our new model captures this where other alternatives do not.
Health-related quality of life: cardiovascular events
To quality adjust expected survival for cost–utility analysis of a state-transition models, we require estimates of the quality of life associated with each of the model states. We updated the health state utility values from the CG181 model using a systematic review and in accordance with the Centre for Reviews and Dissemination guidelines.171 To identify estimates for each model state, we adopted a pragmatic approach following recommendations in the NICE Decision Support Unit’s technical support document for utility values.134
Table 50 reports the focus [i.e. population, intervention, comparator, outcome, study type (PICOS)] of this review. Two reviewers screened titles and abstracts for inclusion, resolving conflicts by consensus. Following this, one reviewer screened full texts for inclusion. A second reviewer checked a sample of full texts. One reviewer extracted data from the included studies. The protocol was published (PROSPERO CRD42021249959).172
TABLE 50
Focus of the review
We searched MEDLINE and EMBASE databases via Ovid. The search strategy combined cardiovascular terms from CG181 with the York precision-maximising filter for utility values.173 We excluded studies if they were unavailable in English or used only condition-specific measures of quality of life.
The search returned 5080 distinct studies, of which 4132 were excluded on abstract screening (Figure 38). A further 526 studies were excluded on full-text screening for the following reasons: 165 studies had no primary data; 128 studies did not consider any of our specified health states; 32 studies considered unrepresentative subgroups of specified states only; 2 studies collected only condition-specific measures of health-related quality of life; 157 studies did not report overall health-related quality-of-life estimate; and 44 studies were unavailable to download or were unavailable in English.
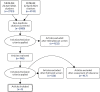
FIGURE 38
A PRISMA (Preferred Reporting Items for Systematic Reviews and Meta-Analyses) flow chart for systematic review of health state utility values.
The review results included studies that collected EQ-5D-3L responses from British populations with each of the specified conditions, with the exception of PAD. Estimates based on European EQ-5D-3L responses, evaluated according to the British tariff, were available for PAD. For each of the events that had an associated post-event state, it was possible to source HSUVs for post-event states from the same source as first year.
We filtered the results of the review to show studies that evaluated the EQ-5D-3L responses of British, or similar, populations according to a UK tariff. We assessed the relevance of these studies to our purposes using high-level characteristics, such as sample size and the number of conditions of interest considered. We identified the studies judged to be most suitable as candidates from which to source disutility multipliers for the model. We used a self-developed quality assessment tool to compare candidate studies according to risk of bias and applicability. There were nine candidate studies in total.174–182
A set of disutilities for the health states of interest was chosen according to their critical appraisal performance and clinical face validity when compared with one another. Two clinicians assessed the face validity of the chosen set of disutilities. The two clinicians confirmed that each estimate, as well as the rank order between them, was plausible.
We selected a study recruiting people experiencing their first TIA (n = 314) or stroke (n = 445) across five general practices.174 Heart failure estimates were sourced from cases (n = 260) detected from clinics/acute care/GPs at two UK sites in 2006–8.176 For SA, we pooled results across three management strategies for people aged ≥ 30 years with suspected SA who were suitable for revascularisation.182 For PAD, we used data from 204 consecutive cases of PAD diagnosed by ankle-brachial index of ≤ 0.9 in the Netherlands, evaluated using UK tariff.180
If we were to choose our preferred sources for each individual state independently, there is a risk that we would be left with an implausible ranking of multipliers. For MI, it appeared that a study covering all UK cases in a 5-year period181 was the best source based on critical appraisal and recruitment policy. However, when chosen together with our preferred source for SA,182 the study suggested that angina decreases health-related quality of life to a greater extent than MI. To avoid an implausible ordering of these states, we chose a third study (which considered multiple states of interest, but considered only three sites rather than the whole UK) for both UA (n = 898) and MI (n = 1176).179 People who were aged ≥ 18 years and who had not been revascularised or received a coronary artery bypass graft within the previous 6 months were eligible for inclusion in this study. We did not use separate values for any comorbidities of the conditions modelled. We did not use three of the candidate studies175,177,178 identified by the review.
Three of the source studies174,179,182 had the advantage of being longitudinal. We calculated average annual utility from the time points given using the AUROC approach. Otherwise, the single estimates provided were used.176,180 The studies used for stroke, TIA and MI provided estimates of quality of life 1 month after the event in question, but did not give a baseline value.174,179 For stroke and TIA, our area under the curve calculations assumed that baseline values were equal to those at 1 month. For MI, we applied the ratio of baseline to 1-month estimates from an alternative source181 to the 1-month estimates from the study used to derive a baseline estimate.
We applied utility values to baseline quality of life using a multiplicative approach. For stroke and TIA, the study reported health-related quality of life of controls without CVD174 and so we used this directly to estimate the relative impact of the events. For other states, we calculated disutility multipliers by comparing reported EQ-5D-3L values with our prediction of baseline utility for people with the same mean age and proportion of men and women but no CVD (see above). The model multiplies the age-and sex-specific baseline utility by the relevant disutility multiplier to adjust people’s quality of life accordingly (Table 51).
TABLE 51
Disutility multipliers used in the model (base case)
Resource use and costs: cardiovascular events
We performed a rapid review using pearl-growing from two previously known sources: one for stroke/TIA140 and one for cardiovascular events.141 Table 52 reports the focus (PICOS) of this review. One researcher conducted the pearl-growing and checked citations of included studies. For the rapid review, the researcher used the medical subject heading terms for the original pearl papers to find similar papers in both MEDLINE and EMBASE using Ovid Online. These papers were combined with the papers found using the ‘Find Similar’ tool within Ovid Online. Finally, all references within the pearls were extracted and combined with any papers citing the pearls. The researcher then removed duplicates and screened titles and abstracts of studies identified for inclusion based on PICOS. Following this, two researchers screened full texts for inclusion based on PICOS. One researcher extracted data from the included studies and a second researcher checked the extraction.
TABLE 52
Focus of the review
We included five studies140,141,182–184 and used the studies to select a set of base-case values (Table 53).
TABLE 53
Studies included in the rapid review of health-state costs
We required annual costs in both the year of the event and following years (i.e. post event). When possible, we chose studies reporting values in a format that most closely reflected first-year and post-event costs for the base case. We sought to source the post-event costs from the same studies as the year 1 costs.
Furthermore, we required incremental costs compared with healthcare costs for somebody in the primary prevention population and, therefore, we chose studies reporting incremental costs for the base case where possible. When we deemed estimates to be equally relevant, we chose those with more recent cost-years for the base case. All cost-years were projected to 2019–20, which was the year for which the most recent inflators163 and Personal Social Services Research Unit costs of health and social care159 were available at the time of analysis.
We discounted estimated social care costs of stroke and post-stroke by 50% to reflect the proportion of this cost that we assume is paid out-of-pocket by individuals (this assumption has precedent in NICE guidelines185–187). In the source study,140 disaggregated health and social care costs were available for only all strokes and not for ischaemic stroke, which we preferred for our decision context. Therefore, we approximated the required numbers by applying the ratio of overall ischaemic stroke costs to overall all stroke costs to disaggregated health and social care costs for all strokes.
One study184 reported a regression model to calculate costs depending on characteristics, such as age and sex. Therefore, we adjusted costs from this study for start age and sex of each cohort modelled. The average cost reported in each other study was applied to all cohorts.
Two studies141,184 included reported estimates for multiple model states. In the base case, we prioritised choosing the most relevant estimates for each state over consistently sourcing from the same paper, and this meant that we used a combination of estimates from the two studies.141,184 We did, however, model scenarios that relied on each of the two studies wherever possible and used base-case values otherwise. In one of these scenarios, reported model coefficients were applied to costs for SA to calculate costs for other states because people with SA were the reference population for the source study.184 In a final two scenarios, we used the values from CG181 in its original cost-year and then inflated to 2019–20.
Formal specification of relative survival model
We used the multiplicative version of the relative survival model from formula 6 of Andersen et al.142 to predict the effect of cardiovascular risk on time of non-cardiovascular death. Let the adjusted hazard of non-cardiovascular death be given by λunadj. Therefore, as in Equation 12 of Pohar and Stare,188 the adjusted hazard, λadj., for covariate values [x1,..., xk]d and coefficients [β0, β1,..., βk], is given by:
We can express λunadj. as the exponent of its own natural logarithm. Therefore, the above is equivalent to:
Then, applying the laws of multiplication for exponents, we obtain:
This can be interpreted in the same way as a Cox model with a log-transformed unadjusted hazard of survival included as a covariate and with coefficient βf fixed at 1:
Accounting for competing risk of non-cardiovascular death
As explained in the main text, the critical covariate for our relative survival models is Δlogit(Q), that is the difference between an individual’s predicted 10-year QRISK3 score and the average score for a person of the same age and sex. We estimate the latter using a regression on the CPRD data set. Table 54 shows the model coefficients and Figure 39 shows the model fitted for men and women as a function of age. We found that the relationship between age and logit(QRISK3) was best described by a quintic function. Although still-higher-order polynomials reduced measures of model fit (AIC) further, we observed that their introduction caused artefacts at high ages (e.g. a sudden reduction in expected risk) that strongly suggested overfitting. Therefore, we preferred the quintic model.
TABLE 54
Regression estimating population average logit(QRISK3 10-year cardiovascular risk) as a function of sex and age
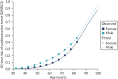
FIGURE 39
Average 10-year risk of cardiovascular event (QRISK3) in people with no history of CVD as a function of age and sex.
- Supplementary methods for model assessing cost-effectiveness of statins for the ...Supplementary methods for model assessing cost-effectiveness of statins for the primary prevention of cardiovascular disease - The implications of competing risks and direct treatment disutility in cardiovascular disease and osteoporotic fracture: risk prediction and cost effectiveness analysis
Your browsing activity is empty.
Activity recording is turned off.
See more...