This work was produced by Guthrie et al. under the terms of a commissioning contract issued by the Secretary of State for Health and Social Care. This is an Open Access publication distributed under the terms of the Creative Commons Attribution CC BY 4.0 licence, which permits unrestricted use, distribution, reproduction and adaptation in any medium and for any purpose provided that it is properly attributed. See: https://creativecommons.org/licenses/by/4.0/. For attribution the title, original author(s), the publication source – NIHR Journals Library, and the DOI of the publication must be cited.
NCBI Bookshelf. A service of the National Library of Medicine, National Institutes of Health.
Guthrie B, Rogers G, Livingstone S, et al. The implications of competing risks and direct treatment disutility in cardiovascular disease and osteoporotic fracture: risk prediction and cost effectiveness analysis. Southampton (UK): National Institute for Health and Care Research; 2024 Feb. (Health and Social Care Delivery Research, No. 12.04.)
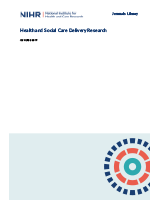
The implications of competing risks and direct treatment disutility in cardiovascular disease and osteoporotic fracture: risk prediction and cost effectiveness analysis.
Show detailsBackground
Although the age-specific incidence of CVD has fallen steadily in most developed countries for several decades, ageing populations mean that CVD remains a major cause of morbidity and mortality worldwide. UK guidelines for the primary prevention of CVD recommend that clinicians use a risk prediction tool to target treatment with statins at people whose predicted risk exceeds a specified threshold. The recommended threshold has been progressively reduced, with NICE changing its recommendation for England and Wales from a 10-year CVD risk of > 20% to > 10% in 2014.10 US guidelines recommend a 7.5% threshold, although the included events are not identical.53 Risk thresholds have been reduced because of increasing evidence of statin effectiveness for primary prevention, and the increasing cost-effectiveness of statins at lower thresholds of baseline risk because statin prices have fallen as they come off patent.
Such risk-stratified guideline recommendations are reliant on the availability of prediction tools for CVD risk. The risk prediction tools recommended in different countries and guidelines vary, reflecting variation in CVD risk factors and incidence, and reflecting that locally derived and validated tools are more likely to be appropriate to local contexts. NICE recommends the use of QRISK2 to predict CVD risk.10 QRISK2 has been externally validated in UK primary-care data sets, and has excellent discrimination and calibration at whole-population level when evaluated on its own terms (ignoring competing mortality risk).16 QRISK3 is a new version of the same tool that includes additional morbidities in prediction. QRISK3 has been derived and internally validated using the same methodology as for QRISK2,25 and in internal validation has excellent model discrimination in the overall population and among younger people, but only good discrimination among older people (defined as those aged ≥ 60 years).25 External validation is required before recommending any prediction tool for routine use.20,21,54,55
However, as with its predecessors, QRISK3 does not account for competing mortality risk in its derivation. The effects of competing mortality risk are obvious in the extreme: taking a statin is clearly futile for someone receiving end-of-life care for terminal cancer. However, across a 10-year prediction time horizon, less dramatic levels of competing mortality risk can lead to systematic and clinically significant overprediction of CVD risk among people at higher risk of dying from another cause, which will particularly apply to older people and those with multimorbidity.34,35
In addition, because age dominates CVD risk, a risk prediction tool that covers a wide range of ages (25–84 years in the case of QRISK3) will always have good discrimination at overall population level. However, discrimination and calibration in subgroups may be poor. This is observed in reported discrimination for QRISK3 in internal validation, where, for example, discrimination is better among younger than older people, and is better among those with type 1 diabetes than those with type 2 diabetes (see the supplementary appendix of the derivation and internal validation paper25), although calibration in different groups is not reported.
In terms of NICE’s surveillance review of the lipid modification guideline, two particular subgroups of interest are people with type 1 diabetes and people with CKD.13 There is some evidence that models derived in people with diabetes have somewhat better discrimination in diabetic populations than models derived in the general population, although the evidence is primarily for people with type 2 diabetes.56 Discrimination of CVD risk prediction models in people with type 2 diabetes is generally poor,57 particularly in older adults.58 QRISK3 does include a type 1 diabetes variable and so, in principle, provides a type 1 diabetes-specific prediction in a model derived from a whole population,25 and the Steno Type 1 Risk Engine (Steno Diabetes Center, Copenhagen, Denmark) provides an alternative derived from a population of people with type 1 diabetes;59 however, at the time this study was carried out, neither tool had been externally validated (an external validation60 published after we completed this element of the study is discussed in Summary). In people with CKD, CVD risk prediction tools that do not account for CKD substantially underpredict CVD risk,61–63 although adding a detailed indicator of estimated glomerular filtration rate (eGFR) and albuminuria to models calibrated to the CKD population leads to only small improvements in discrimination.64
This study, therefore, externally evaluates the performance of QRISK3 both in its own terms (ignoring competing mortality risk) and accounting for competing risk, and examines model performance in subgroups of the population defined by age and by levels of comorbidity [a modified Charlson Comorbidity Index65 (mCCI)], for whom competing mortality risk is likely to vary, and in people with type 1 diabetes and CKD.
We then derive a new CVD prediction model based on QRISK3 that accounts for competing mortality risks (i.e. the CRISK), internally validate the model in the same data set and examine reclassification from using CRISK compared with using QRISK3 to identify people with predicted 10-year CVD risk of > 10%.
This chapter reports methods and findings for CVD risk prediction models in relation to objectives 1 and 2, as follows:
- To externally validate the recommended risk prediction tools for primary prevention of CVD (QRISK3), including performance in important subgroups, and for osteoporotic fracture (QFracture-2012).
- To derive and internally validate new-incident CVD (and osteoporotic fracture) risk prediction models, accounting for competing risks of death, and compare performance with existing risk prediction models.
Methods
Data sources
Data used in this study were taken from CPRD GOLD,66 which derives data from general practices using INPS Vision electronic health records and is distinct from the derivation data set, which is derived from practices using the EMIS system. Identical to QRISK3 derivation and internal validation, patients were eligible for inclusion if they:
- were permanently registered with a general practice, contributing up-to-standard data for at least 1 year and with consent to link GP data to hospital discharge (Hospital Episodes Statistics Admitted Patient Care) and mortality [Office for National Statistics (ONS) mortality registration] data
- were aged ≥ 25 years and < 85 years
- had no prior history of any CVD
- had no prior history of statin prescription.
Cohort entry was defined as the latest date of an individual’s date of registration plus 1 year, the individual’s 25th birthday or 1 January 2004. Cohort exit was defined as the earliest of:
- the first non-fatal or fatal cardiovascular event
- receipt of a statin prescription
- deregistration from their participating general practice
- date of last data collection from their participating general practice
- end-of-study follow-up on 31 March 2016.
Of note, the QRISK3 derivation and internal validation did not censor on statin prescription, but we chose to, as the primary purpose of the tool is to inform decisions on statin initiation.
Sample size
The sample size is fixed by the size of the CPRD GOLD data set. Therefore, no formal power calculation was carried out, as it could not alter study design and the available sample size was considered sufficient for the purpose.54
Outcome definition
The outcome was the first CVD event experienced by an individual, defined as the earliest GP, hospital or mortality record of non-fatal coronary heart disease, ischaemic stroke or transient ischaemic attack (TIA). Outcomes were defined using Read codes (for GP data) and International Statistical Classification of Diseases and Related Health Problems, Tenth Revision (ICD-10) codes (for hospital discharge and mortality data). ICD-10 codes are those listed in the published QRISK3 derivation paper;25 however, there are no published Read codesets available. We, therefore, derived our own Read codeset, and this and the ICD-10 codes are listed in appendices to the published paper67 reporting our external validation of QRISK3.
Other variable definitions
At the time the analysis was done, there were no published Read codesets for other variables included in the QRISK3 model, which we defined using Read codes in GP data which we created for this study and values [e.g. systolic blood pressure (SBP), cholesterol] in GP data (listed in our published paper).67 There were several data-handling variations compared with the original QRISK3 derivation:
- We chose a later cohort entry date (1 January 2004 vs. 1 January 1998).
- Where no cholesterol value was available at baseline, then QRISK3 allowed cholesterol values after the cohort entry date to be used provided that they were before any CVD event. In contrast, we used values from before the cohort entry date only to avoid using future information in prediction.
- CPRD makes only group Townsend deprivation scores available as vigintile (equal 20th) of Townsend score. Therefore, we estimated the median Townsend score of national vigintiles and used that in prediction.
In addition, we calculated a mCCI based on Read codes in the GP data, using a published codeset.65 The mCCI was modified in that the original Charlson Comorbidity Index (CCI) includes several CVDs that are, by definition, excluded at baseline in this study because participants are CVD free at baseline. The mCCI was not used in prediction but was used to examine discrimination and calibration in subgroup analysis (categorised as 0, 1, 2 and ≥ 3), along with age group (categorised as 25–44 years, 45–64 years, 65–74 years and 75–84 years).
Missing data
Missing data handling and the proportion of each variable with missing data are shown in Appendix 1, Table 24. As with QRISK3 derivation, patients were excluded if the Townsend deprivation score was missing, patients with missing data on ethnicity were assumed to be white and patients with no record of a condition were assumed not to have the condition. For continuous variables [i.e. body mass index (BMI), total cholesterol : high-density lipoprotein ratio (TC : HDL), SBP, SBP variability] and for smoking status, multivariate imputation via chained equations68 was used to generate five imputed data sets. Analyses of these imputed data sets were combined using Rubin’s rules to account for the uncertainty association with imputation.69
Analytical methods: external validation
The published QRISK3 2017 prediction model was implemented (under GNU Lesser General Public Licence v3), and the predicted 10-year risk of experiencing a CVD event was calculated for each patient without recalibration of baseline risk. Model performance was evaluated by examining discrimination and calibration.
Discrimination evaluates how well the risk score differentiates between patients who experience a CVD event (or more generally, the event of interest) during the study and patients who do not. We primarily examined discrimination using the truncated version of Harrell’s c-statistic to include only pairs where the earliest survival time was no later than 10 years after entry. A c-statistic of 0.5 indicates that the risk score performs no better than chance, whereas a c-statistic of 1 indicates perfect discrimination. Evaluating how good discrimination is for values between 0.5 and 1 is arbitrary and involves judgement. We considered c-statistic values of 0.5–0.599 poor, values of 0.6–0.699 moderate, values of 0.7–0.799 good and values > 0.8 excellent.
Two additional measures of discrimination were calculated. First, we calculated Royston and Sauerbrei’s D-statistic (based on the separation in event-free survival between patients with predicted risk scores above and below the median, where higher values indicate greater discrimination and a difference of ≥ 0.1 is suggested as indicating a meaningful difference in discrimination).70 Second, we calculated a related R2-statistic designed for estimating explained variation in censored survival data.71
Models may have good discrimination but imperfectly predict risk, for example by systematically overpredicting or underpredicting. Examination of calibration is, therefore, important, particularly where predicted risk is used to determine offers of treatment, as it is for primary prevention of CVD.10 Calibration refers to how closely the predicted and observed probabilities agree at group level, and for this purpose participants were grouped into 10 equal-sized groups (deciles) of predicted risk. Calibration of the risk score predictions was assessed by plotting observed proportions against predicted probabilities. For both men and women separately, plots were generated for all patients and for prespecified subgroups of age, mCCI, diabetes type and CKD, based on summary statistics pooled across the imputed data sets. Subgroups were defined to ensure that there were enough events in each subgroup to ensure stable estimates of observed risk (and for diabetes and CKD, analysis was, therefore, in the whole subgroup without further stratification for age group or mCCI). CKD was defined in two ways: (1) only using Read codes,67 as per QRISK3 derivation25 and (2) using the same set of Read codes or the last recorded eGFR or eGFR based on last recorded serum creatinine, where an eGFR < 60 ml per minute defined CKD.
The following summary statistics and their standard errors (SEs) were obtained by decile of predicted risk score and for each imputed data set, in turn: non-parametric measures of observed risk or proportions of patients with a CVD event, the Kaplan–Meier estimator (the conventional measure ignoring competing risks), the Aalen–Johansen estimator (an extension to allow for competing events, non-CVD death in this case)16 and the mean predicted risk score. All models were fitted in R 4.0.0 (The R Foundation for Statistical Computing, Vienna, Austria) and Stata® 11.2 (StataCorp LP, College Station, TX, USA).
Analytical methods: competing risk model derivation and internal validation
Competing risk model derivation and internal validation was carried out in the same data set as QRISK3 external validation. For this purpose, participants were randomly allocated to distinct derivation and test data sets in a 2 : 1 ratio, with allocation balanced in terms of age and final event status. The derivation data set was used to derive CRISK, that is a Fine–Gray model to predict the 10-year risk of experiencing a CVD event, accounting for the competing risk of non-CVD death. Separate models were estimated for men and women. Reflecting the overall aim of the project, where we wished to explicitly compare prediction in models accounting for competing risk compared with ignoring competing risk, we included all of the same main effects (i.e. predictors) and age interactions as QRISK3, modified as follows. First, we accounted for non-CVD death as a second (competing) outcome using the Fine–Gray model, and we re-estimated fractional polynomial terms for continuous variables, including in QRISK3, selecting terms based on those performing best (as measured by the c-statistic) in balanced 10-fold cross-validation and showing consistency of model fit [i.e. Akaike information criterion (AIC)] across folds of the derivation data set (this model is called CRISK). Second, as QRISK3 predictors are focused on CVD events, we derived a further model [i.e. the competing mortality risk model with Charlson Comorbidity Index (CRISK-CCI)], which additionally included the mCCI score in the model (categorised as 0, 1, 2, ≥ 3), as CCI is a well-validated predictor of total mortality.12 Fine–Gray models allow the cumulative incidence function or probability of a CVD event occurring over time to be directly predicted; however, the subdistribution hazard ratios (HRs) in the Fine–Gray models do not have a straightforward interpretation, as they describe the direction but not the magnitude of the effect of predictors on the cumulative incidence function. The use of fractional polynomials and the inclusion of complex interactions with age further complicate direct interpretation of model coefficients. Model coefficients are, therefore, not straightforwardly interpretable, but the derived model is provided in Appendix 1, Tables 25 and 26, to allow replication.
The performance of all three models (i.e. CRISK, CRISK-CCI and QRISK3) was evaluated in the independent validation data set by examining discrimination and calibration, as described above. R 4.0.0 was used for all analyses.
Results 1: external validation of QRISK3 in the whole population
The external validation data set had 1,648,746 women aged 25–84 years with linkage to Hospital Episode Statistics (HES) and ONS. Of these women, 164,129 (10.0%) were excluded because of missing deprivation score (0.2%), prior CVD (4.7%) or prior statin prescribing (5.1%). The external validation data set had 1,621,535 men aged 25–84 years with linkage HES and ONS. Of these men, 201,359 (12.4%) were excluded because of missing deprivation score (0.2%), prior CVD (6.9%) or prior statin prescribing (5.3%). Therefore, analysis used data for 1,484,597 women and 1,420,176 men.
The baseline characteristics of participants compared with the QRISK3 internal validation cohort25 are shown in Appendix 1, Table 27. The two cohorts were similar, although there was a higher prevalence of treated hypertension in this study, and a lower recorded prevalence of family history of premature CVD. Appendix 1, Table 24, shows that ethnicity data were less frequently missing in this study, but that TC : HDL, SBP variability and smoking status were more commonly missing (which may reflect the use of data after study entry date in QRISK3 derivation).
In women, during 8,594,620 years of follow-up, there were 42,451 incident cases of CVD observed {4.94 [95% confidence interval (CI) 4.89 to 4.99] per 1000 person-years}. In men, during 7,896,704 years of follow-up, there were 53,066 incident cases [6.72 (95% CI 6.66 to 6.78) per 1000 person-years]. Incidence progressively rose with age, from 0.3 cases per 1000 person-years in both men and women aged 25–29 years, to 44.1 cases in women aged 80–84 years and to 52.6 cases in men aged 80–84 years. CVD incidence was moderately lower than that observed in QRISK3 derivation (see Appendix 1, Table 28).4
In the whole population, discrimination was excellent in both women (Harrell’s c-statistic 0.865, 95% CI 0.861 to 0.868) and men (Harrell’s c-statistic 0.834, 95% CI 0.831 to 0.837), and very similar to QRISK3 internal validation (women, Harrell’s c-statistic 0.880; men, Harrell’s c-statistic 0.858)25 (Table 1). The D-statistic was 2.43 in women (similar to the internal validation study’s D-statistic of 2.49) and 2.10 in men (somewhat lower than the internal validation study’s D-statistic of 2.26). Explained variation (R2) was 58.5% in women and 51.3% in men, compared with 59.6% and 55.0%, respectively, in the internal validation study. In all strata of age group, discrimination was worse in both men and women, varying from good in younger people (age 25–44 years Harrell’s c-statistic: women, 0.865; men, 0.757) to poor to moderate in older people (age 75–84 years Harrell’s c-statistic: women, 0.611; men, 0.585), with low levels of explained variation in older people (age 75–84 years R2: women, 8.1%; men, 4.9%). Stratified by mCCI, discrimination was excellent in people with low comorbidity, but progressively less good in people with higher comorbidity, but with less change than for age group (mCCI ≥ 3 Harrell’s c-statistic: women, 0.744; men, 0.695).
TABLE 1
Discrimination of QRISK3 in the whole population and stratified by age group and mCCI
Ignoring competing mortality risk in the estimation of observed risk (Figures 1 and 2, parts a, c and e), calibration in the whole population was very good, with only minor overprediction in people at higher predicted risk (see Figures 1 and 2, part a). Stratified by age, overprediction was larger in older people (see Figures 1 and 2, part b). Stratified by mCCI, there was some overprediction in people with no baseline comorbidity, but underprediction in those with comorbidity (see Figures 1 and 2, part c). Accounting for competing mortality risk in the estimation of observed risk (see Figures 1 and 2, parts b, d and f), overprediction was larger in the whole population and in all age groups apart from the youngest (i.e. people aged 25–44 years) (see Figures 1 and 2, parts d and e). Stratified by mCCI, underprediction was still observed in people with higher comorbidity at lower levels of predicted risk, but there was large overprediction in people with higher comorbidity at higher levels of predicted risk (see Figures 1 and 2, part f).
Results 2: external validation of QRISK3 in people with diabetes
Type 1 diabetes
There were 6025 women with type 1 diabetes potentially eligible for inclusion, of whom 646 (10.7%) and 1627 (27.0%) were excluded because of prior CVD or prior statin prescribing for primary prevention, respectively. There were 8260 men with type 1 diabetes potentially eligible for inclusion, of whom 953 (11.5%) and 2464 (40.9%) were excluded because of prior CVD or prior statin prescribing for primary prevention, respectively. Therefore, 3752 women (62.3% of potentially eligible women with type 1 diabetes) and 4843 men (48.6% of potentially eligible men with type 1 diabetes) were included in analysis of type 1 diabetes.
During follow-up of the type 1 diabetes cohort, there were 108 CVD events in 13,098 person-years’ follow-up in women [8.25 (95% CI 6.83 to 9.94) events per 1000 person-years] and 172 CVD events in 15,824 person-years’ follow-up in men [10.90 (95% CI 9.40 to 12.60) events per 1000 person-years].
Discrimination in people with type 1 diabetes was excellent [Harrell’s c-statistic: women, 0.830 [95% CI 0.768 to 0.891; men, 0.853 (95% CI 0.803 to 0.902)] and explained variance in the model was 51.6% in women and 48.0% in men (see Appendix 1, Table 29).
Ignoring competing risks (see Appendix 1, Figure 28, parts a and c), calibration in women with type 1 diabetes was good (allowing for small number of events and, therefore, a relatively noisy plot), but there was some overprediction in men at higher predicted risk. Accounting for competing risks (see Appendix 1, Figure 28, parts b and d), there was overprediction in women at higher predicted risk and greater overprediction in men at higher predicted risk.
Type 2 diabetes
There were 53,284 women with type 2 diabetes potentially eligible for inclusion, of whom 12,068 (22.6%) and 24,194 (45.4%) were excluded because of prior CVD or prior statin prescribing for primary prevention, respectively. There were 68,236 men with type 2 diabetes potentially eligible for inclusion, of whom 19,777 (28.9%) and 27,382 (40.1%) were excluded because of prior statin prescribing for primary prevention, respectively. Therefore, 24,194 women (i.e. 31.9% of the potentially eligible women with type 2 diabetes) and 21,077 men (i.e. 30.9% of the potentially eligible men with type 2 diabetes) were, therefore, included in analysis of type 2 diabetes.
During follow-up of the type 2 diabetes cohort, there were 1167 CVD events in 44,678 person-years’ follow-up in women [26.12 (95% CI 24.68 to 27.64) events per 1000 person-years] and 1682 CVD events in 57,160 person-years’ follow-up in men [29.40 (95% CI 28.10 to 30.80) events per 1000 person-years].
Discrimination in people with type 2 diabetes was moderate to good [Harrell’s c-statistic: women, 0.741 (95% CI 0.722 to 0.760); men, 0.695 (95% CI 0.679 to 0.712)] and explained variance in the model was lower than for type 1 diabetes (i.e. 29.2% in women and 22.0% in men) (see Appendix 1, Table 29).
Ignoring competing risks (see Appendix 1, Figure 29, parts a and c), calibration in women with type 2 diabetes was good, but there was some overprediction in men at the highest predicted risk. Accounting for competing risks (see Appendix 1, Figure 29, parts b and d), there was progressively increasing overprediction in women with moderate to high predicted risk and in men in all but the lowest deciles of predicted.
Results 3: external validation of QRISK3 in people with chronic kidney disease
Chronic kidney disease defined by Read code alone
There were 16,048 women with CKD defined by Read code alone potentially eligible for inclusion, of whom 4223 (26.3%) and 4897 (30.5%) were excluded because of prior CVD or prior statin prescribing for primary prevention, respectively. There were 15,784 men with CKD defined by Read code alone potentially eligible for inclusion, of whom 5645 (35.7%) and 3850 (24.4%) were excluded because of prior CVD or prior statin prescribing for primary prevention, respectively. Therefore, 6918 women (i.e. 43.1% of the potentially eligible women with CKD defined by Read code alone) and 5659 men (i.e. 35.9% of the potentially eligible men with CKD defined by Read code alone) were, therefore, included in analysis of CKD defined by Read code alone. The mean age of women was 63.0 years and mean age of men was 59.2 years.
During follow-up of the CKD defined by Read code alone cohort, there were 541 CVD events in 25,544 person-years’ follow-up in women [21.18 (95% CI 19.48 to 23.02) events per 1000 person-years] and 569 CVD events in 21,459 person-years’ follow-up in men [26.50 (95% CI 24.40 to 28.80) events per 1000 person-years].
Discrimination in people with CKD defined by Read code alone was good [Harrell’s c-statistic: women, 0.755 (95% CI 0.728 to 0.782); men, 0.734 (95% CI 0.708 to 0.760)] and explained variance in the model was 34.2% in women and 29.7% in men (see Table 30).
Ignoring competing risks (see Appendix 1, Figure 30, parts a and c), calibration in women with CKD defined by Read code alone was reasonable (allowing for small number of events and, therefore, a relatively noisy plot) and good for men (with some underprediction for both at higher predicted risk). Accounting for competing risks (see Appendix 1, Figure 30, parts b and d), there was overprediction in women at moderate and higher predicted risk and overprediction in men at higher predicted risk.
Chronic kidney disease defined by Read code and estimated glomerular filtration rate
Laboratory values were extracted for only people included in the CVD study cohort and so it is not possible to calculate the proportions excluded because of prior CVD or prior statin prescribing. There were 71,094 women and 33,699 men with CKD defined by Read code or eGFR included in analysis, with an older mean age than the cohort with CKD defined by Read code alone (mean age: women, 70.1 years; men, 69.1 years).
During follow-up of the CKD defined by Read code or eGFR cohort, there were 8877 CVD events in 348,982 person-years’ follow-up in women [25.44 (95% CI 24.92 to 25.96) events per 1000 person-years] and 5273 CVD events in 146,730 person-years’ follow-up in men [35.90 (95% CI 35.00 to 36.90) events per 1000 person-years].
Discrimination in people with CKD defined by Read code or eGFR was somewhat worse than for people with CKD defined by Read code alone. Discrimination was moderate to good [Harrell’s c-statistic: women, 0.705 (95% CI 0.699 to 0.712); men, 0.671 (95% CI 0.663 to 0.680)] and explained variance in the model was somewhat lower than for CKD defined by Read code alone (i.e. 24.9% in women and 17.4% in men) (see Table 30).
Ignoring competing risks (see Appendix 1, Figure 31, parts a and c), calibration in women with CKD defined by Read code or eGFR was excellent, but there was some underprediction in men. Accounting for competing risks (see Appendix 1, Figure 31, parts b and d), there was progressively increasing overprediction in women and men with moderate to high predicted risk.
Results 4: derivation and internal validation of CRISK
There were 989,732 women and 946,784 men aged 25–84 years in the derivation cohort, and 494,865 women and 473,392 men in the validation cohort, with similar distribution of baseline characteristics in each. There were 14,150 incident CVD events in 2,865,660 years of follow-up in women [4.9 (95% CI 4.89 to 4.99) events per 1000 person-years] and 17,689 incident CVD events in 2,632,804 years of follow-up in men [6.7 (95% CI 6.66 to 6.78) events per 1000 person-years].
Two new models were created: (1) CRISK that is a near replication of QRISK3, which accounts for competing risk and (2) CRISK-CCI, which additionally includes the mCCI as a predictor of competing mortality.
In the whole population, discrimination of CRISK and CRISK-CCI was excellent in women and very similar to QRISK3 (CRISK Harrell’s c-statistic: women, 0.863; men, 0.833; CRISK-CCI Harrell’s c-statistic: women, 0.864; men, 0.819) (Table 2). For both new models, discrimination showed similar patterns to QRISK3 in terms of being worse in all age groups (and progressively worse with increasing age) and, to a lesser extent, worse with increasing comorbidity measured by the mCCI (see Table 2).
TABLE 2
Discrimination of CRISK-CCI, CRISK and QRISK3 for men and women in the validation cohort
In terms of calibration (evaluated using only the Aalen–Johansen estimator, which accounts for competing mortality risk in estimated observed risk), QRISK3 overpredicted in both men and women in the whole population, with progressively worse overprediction at higher predicted risk (see Results 1: external validation of QRISK3 in the whole population).
In the whole population of women, there was some overprediction with CRISK at higher levels of predicted risk, but CRISK was better calibrated than QRISK3. Calibration in women with CRISK-CCI was excellent (Figure 3). In younger women, there was some underprediction with all three prediction tools, which were similar, although CRISK was the best calibrated (Figure 4). QRISK3 and CRISK both showed overprediction in middle-aged and older women. CRISK-CCI was well calibrated in women aged 45–64 years and 65–74 years and had some overprediction at higher risk in women aged 75–84 years (but was the best calibrated model). In all CCI categories, there was some overprediction with each model at higher levels of predicted risk, which was greatest with QRISK3 and least with CRISK-CCI, although calibration of all models was broadly the same for mCCI ≥ 3 (Figure 5).
In the whole population of men, calibration using CRISK-CCI was better than calibration using CRISK, which showed some underprediction, whereas QRISK3 somewhat overpredicted CVD risk (see Figure 3). In younger men aged 25–44 years, there was some underprediction with CRISK and QRISK3, but calibration with CRISK-CCI was very good (see Figure 4). In middle-aged and older men, QRISK3 systematically overpredicted. CRISK-CCI was better calibrated in men aged 45–64 years, although CRISK-CCI was overpredicted at the highest decile of predicted risk. CRISK and CRISK-CCI had similar calibration in older men, although CRISK-CCI had greater overprediction at higher levels of predicted risk. In men with increasing CCI, QRISK3 was the least well calibrated, with overprediction in all strata. CRISK-CCI was best calibrated in people with low comorbidity (i.e. mCCI = 0 and mCCI = 1), but had greater overprediction at higher levels of predicted risk than CRISK (see Figure 5).
Summary
QRISK3 external validation
At the whole-population level, QRISK3 has excellent discrimination (which is the ability of the model to distinguish people at higher or lower risk). However, as is expected when examining discrimination in subsets of the modelled population defined by strong predictors of the outcome,72 discrimination was poor to moderate when stratified by age and additionally worse when stratified by level of comorbidity (which was not a predictor in the model). Calibration is the extent to which predicted and observed event rates are similar, and it was excellent in the whole population when ignoring competing mortality risks; however, there was systematic underprediction after competing risks were accounted for. Calibration was considerably worse in older people and in people with higher levels of comorbidity, where QRISK3 systematically overpredicted risk, particularly after competing mortality risks were accounted for.
In people with diabetes, discrimination was excellent in type 1 diabetes and moderate to good in type 2 diabetes. Similar to the whole population, calibration was good, with some overprediction when ignoring competing risks, but there was more consistent overprediction once competing risks were accounted for. Similar findings were found for people with CKD, but it is important to recognise that the populations studied exclude people with prior statin prescribing, which excludes substantial numbers of people with either condition (based on statin prescribing in the primary prevention population, 27.0% of women and 49.9% of men with type 1 diabetes, 45.4% of women and 40.1% of men with type 2 diabetes, and 30.5% of women and 24.4% of men with CKD defined by Read code were excluded).
The published external validation of QRISK2 found excellent discrimination and calibration at the whole-population level when ignoring competing mortality risk (i.e. answering the question ‘what is the risk of CVD assuming this person does not die of anything else in the next 10 years?’).15 This study found similar, but additionally found overprediction and poor calibration in people aged 75–84 years, and moderate calibration in people aged 65–74 years and in people with the highest levels of comorbidity (mCCI = 3).
Once competing mortality risk was accounted for (i.e. answering the question ‘what is the risk of CVD allowing for the risk of death from something else first?’), then there was greater overprediction at the whole-population level, and particularly in older people and in people with more comorbidity. These findings are consistent with other studies examining the impact of competing risks on estimated CVD risk in people without CVD34,73,74 and with established CVD.75
QRISK2 has also been shown to systematically overpredict CVD risk in a contemporary population of people with type 2 diabetes, with increasingly poor discrimination with increasing age76 and underprediction in a contemporary population of people with type 1 diabetes.60 This highlights that good performance at the whole-population level does not necessarily mean good performance in important subgroups,72 and also that models derived in populations excluding prior statin prescribing are likely to be increasingly unrepresentative as statin prescribing increases.
CRISK and CRISK-CCI derivation and internal validation
The two new competing risk models derived (i.e. CRISK and CRISK-CCI) has similar excellent discrimination for CVD events as QRISK3. CRISK was better calibrated than QRISK3 (after accounting for competing mortality in derivation, but without adding any new predictors to the model) and CRISK-CCI was better calibrated again (after adding the mCCI as an additional predictor).
Two studies74,77 in people aged ≥ 65 years have examined the impact of competing mortality risk on CVD prediction. Like this study, the two studies74,77 also found only moderate discrimination of whole-population CVD risk prediction tools in older adults, and that newly derived competing risks models were generally better calibrated than models derived using standard Cox regression.77 In a UK study35 evaluating a new competing risk model against the QRISK2, differences between predicted and observed CVD risk were greatest among people with highest predicted risk, as was found in this study.
Limitations
Limitations of this study are largely those that are found in all studies using routine GP data, including the original QRISK3 derivation.20 First, there is considerable missing data for key predictors. As with QRISK3 derivation, we used multiple imputation for missing data, but the assumption that data are missing at random is a strong one because risk factors are likely to be better recorded in people at higher CVD risk.35 This weakness is balanced against the use of more representative population data than is found in individually recruited research cohorts where data are more complete. Second, we used a more recent index date (1 January 2014) than QRISK3 (1 January 1998), which likely means that we exclude more people with prior statin prescribing. Deriving clinical prediction tools on increasingly historical data is likely biased because CVD incidence is falling,73 but using more recent data with greater rates of exclusion because of prior statin initiation may also be biased.
- Cardiovascular disease risk prediction: external validation of QRISK3 and deriva...Cardiovascular disease risk prediction: external validation of QRISK3 and derivation and internal validation of a new competing risk model (CRISK) - The implications of competing risks and direct treatment disutility in cardiovascular disease and osteoporotic fracture: risk prediction and cost effectiveness analysis
Your browsing activity is empty.
Activity recording is turned off.
See more...